A combined test for feature selection on sparse metaproteomics data-an alternative to missing value imputation
PEERJ(2022)
摘要
One of the difficulties encountered in the statistical analysis of metaproteomics data is the high proportion of missing values, which are usually treated by imputation. Nevertheless, imputation methods are based on restrictive assumptions regarding missingness mechanisms, namely "at random" or "not at random". To circumvent these limitations in the context of feature selection in a multi-class comparison, we propose a univariate selection method that combines a test of association between missingness and classes, and a test for difference of observed intensities between classes. This approach implicitly handles both missingness mechanisms. We performed a quantitative and qualitative comparison of our procedure with imputation-based feature selection methods on two experimental data sets, as well as simulated data with various scenarios regarding the missingness mechanisms and the nature of the difference of expression (differential intensity or differential presence) Whereas we observed similar performances in terms of prediction on the experimental data set, the feature ranking and selection from various imputation-based methods were strongly divergent. We showed that the combined test reaches a compromise by correlating reasonably with other methods, and remains efficient in all simulated scenarios unlike imputation-based feature selection methods.
更多查看译文
关键词
Metaproteomics, Feature selection, Missing value imputation, Combined test
AI 理解论文
溯源树
样例
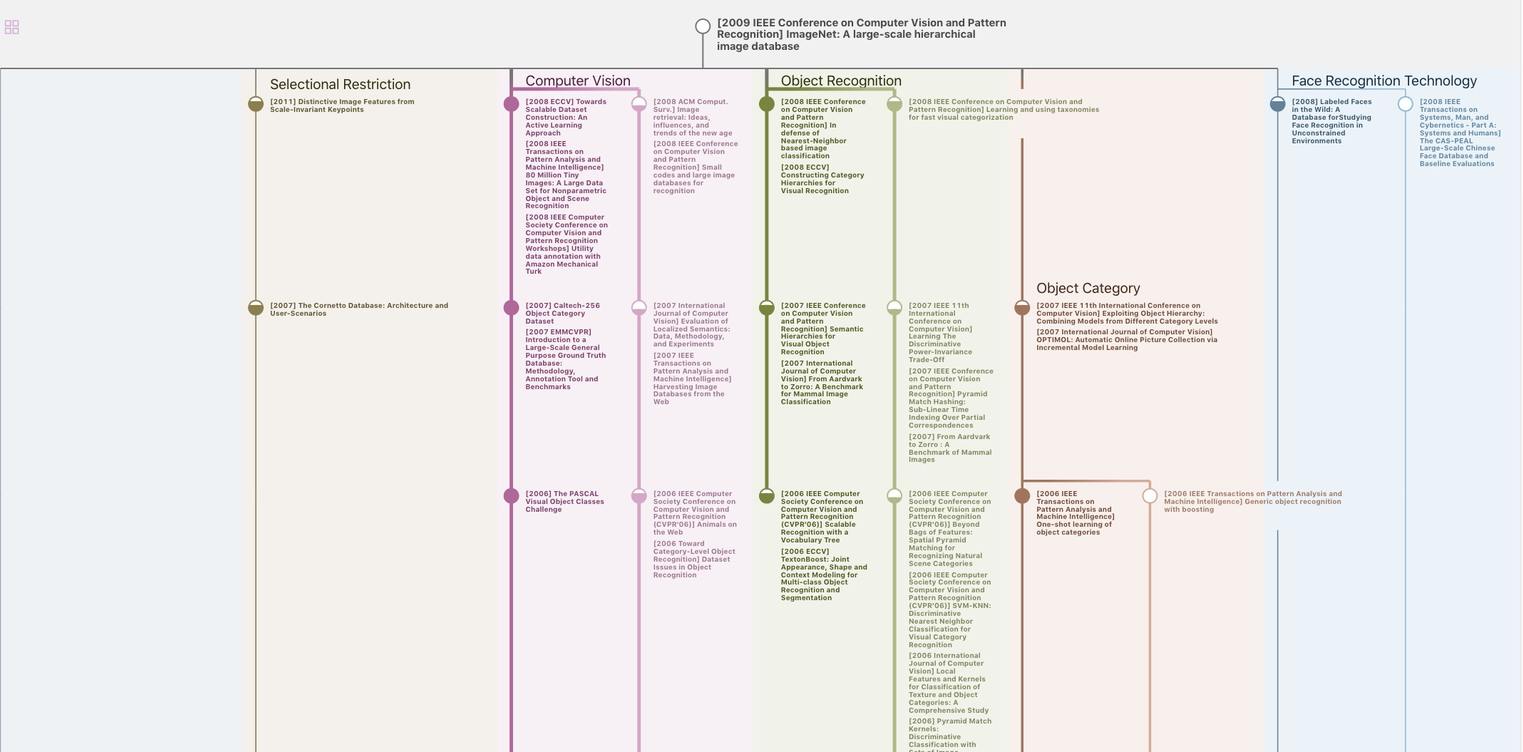
生成溯源树,研究论文发展脉络
Chat Paper
正在生成论文摘要