Modeling Site-Specific Nucleotide Biases Affecting Himar1 Transposon Insertion Frequencies in TnSeq Datasets
mSystems(2021)
摘要
In bacterial TnSeq experiments, a library of transposons insertion mutants is generated, selected under various growth conditions, and sequenced to determine the profile of insertions at different sites in the genome, from which the fitness of mutant strains can be inferred. The widely used Himar1 transposon is known to be restricted to insertions at TA dinucleotides, but otherwise, few site-specific biases have been identified. As a result, most analytical approaches assume that insertion counts are expected a priori to be randomly distributed among TA sites in non-essential regions. However, recent analyses of independent Himar1 Tn libraries in M. tuberculosis have identified a local sequence pattern that is non-permissive for Himar1 insertion. This suggests there are site-specific biases that affect the frequency of insertions of the Himar1 transposon at different TA sites. In this paper, we use statistical and machine learning models to characterize patterns in the nucleotides surrounding TA sites associated with high and low insertion counts. We not only affirm that the previously discovered non-permissive pattern (CG)GnTAnC(CG) suppresses insertions, but conversely show that an A in the -3 position or T in the +3 position from the TA site encourages them. We demonstrate that these insertion preferences exist in Himar1 TnSeq datasets other than M. tuberculosis , including mycobacterial and non-mycobacterial species. We build predictive models of Himar1 insertion preferences as a function of surrounding nucleotides. The final predictive model explains about half of the variance in insertion counts, presuming the rest comes from stochastic variability between libraries or due to sampling differences during sequencing. Based on this model, we present a new method, called the TTN-Fitness method, to improve the identification of conditionally essential genes or genetic interactions, i.e., to better distinguish true biological fitness effects by comparing the observed counts to expected counts using a site-specific model of insertion preferences. Compared to previous methods like Hidden Markov Models, the TTN-Fitness method can make finer distinctions among genes whose disruption causes a fitness defect (or advantage), separating them out from the large pool of non-essentials, and is able to classify the essentiality of many smaller genes (with few TA sites) that were previously characterized as uncertain. ### Competing Interest Statement The authors have declared no competing interest.
更多查看译文
AI 理解论文
溯源树
样例
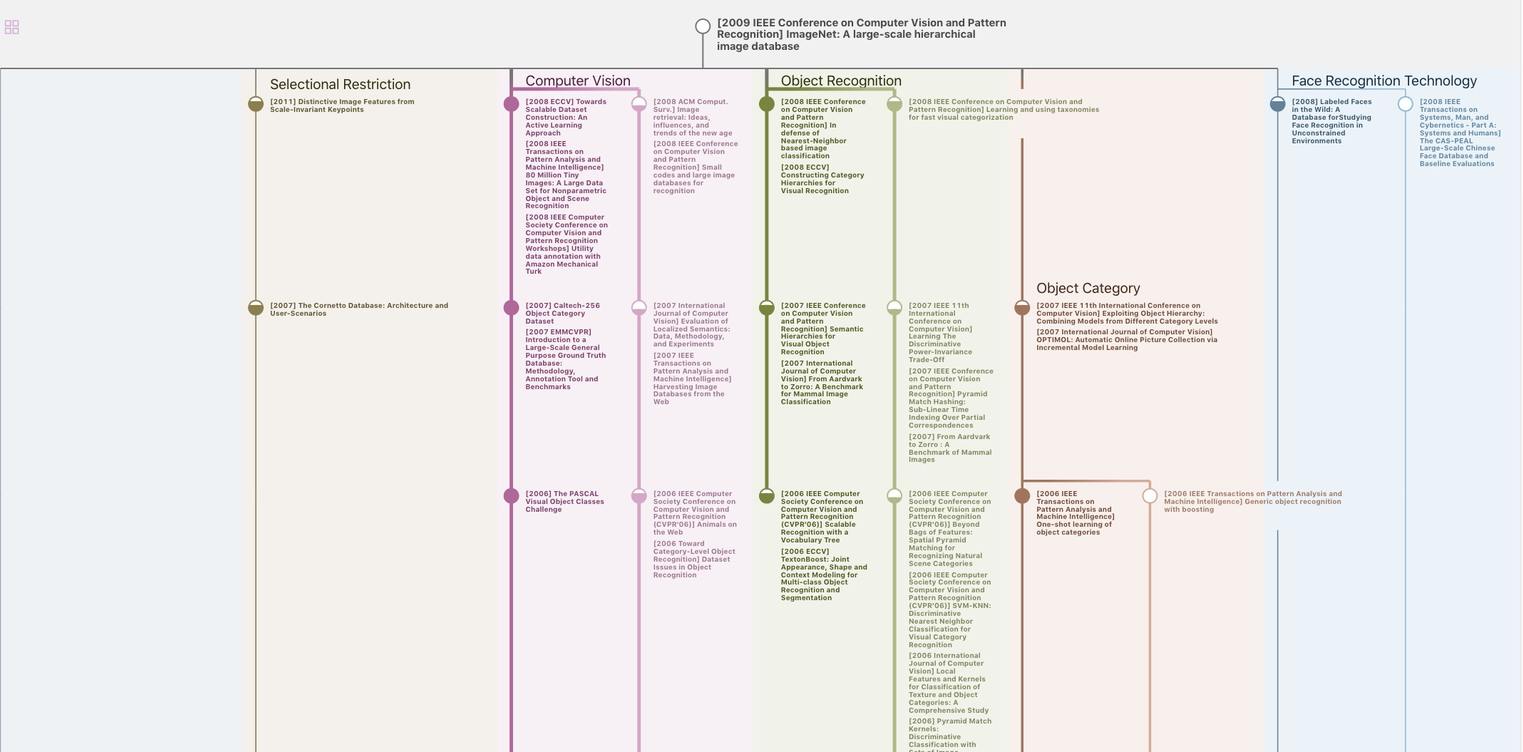
生成溯源树,研究论文发展脉络
Chat Paper
正在生成论文摘要