Model-based identification of conditionally-essential genes from transposon-insertion sequencing data
PLoS Computational Biology(2022)
摘要
The understanding of bacterial gene function has been greatly enhanced by recent advancements in the deep sequencing of microbial genomes. Transposon insertion sequencing methods combines next-generation sequencing techniques with transposon mutagenesis for the exploration of the essentiality of genes under different environmental conditions. We propose a model-based method that uses regularized negative binomial regression to estimate the change in transposon insertions attributable to gene-environment changes in this genetic interaction study without transformations or uniform normalization. An empirical Bayes model for estimating the local false discovery rate combines unique and total count information to test for genes that show a statistically significant change in transposon counts. When applied to RB-TnSeq (randomized barcode transposon sequencing) and Tn-seq (transposon sequencing) libraries made in strains of Caulobacter crescentus using both total and unique count data the model was able to identify a set of conditionally beneficial or conditionally detrimental genes for each target condition that shed light on their functions and roles during various stress conditions.
更多查看译文
关键词
genes,model-based,conditionally-essential,transposon-insertion
AI 理解论文
溯源树
样例
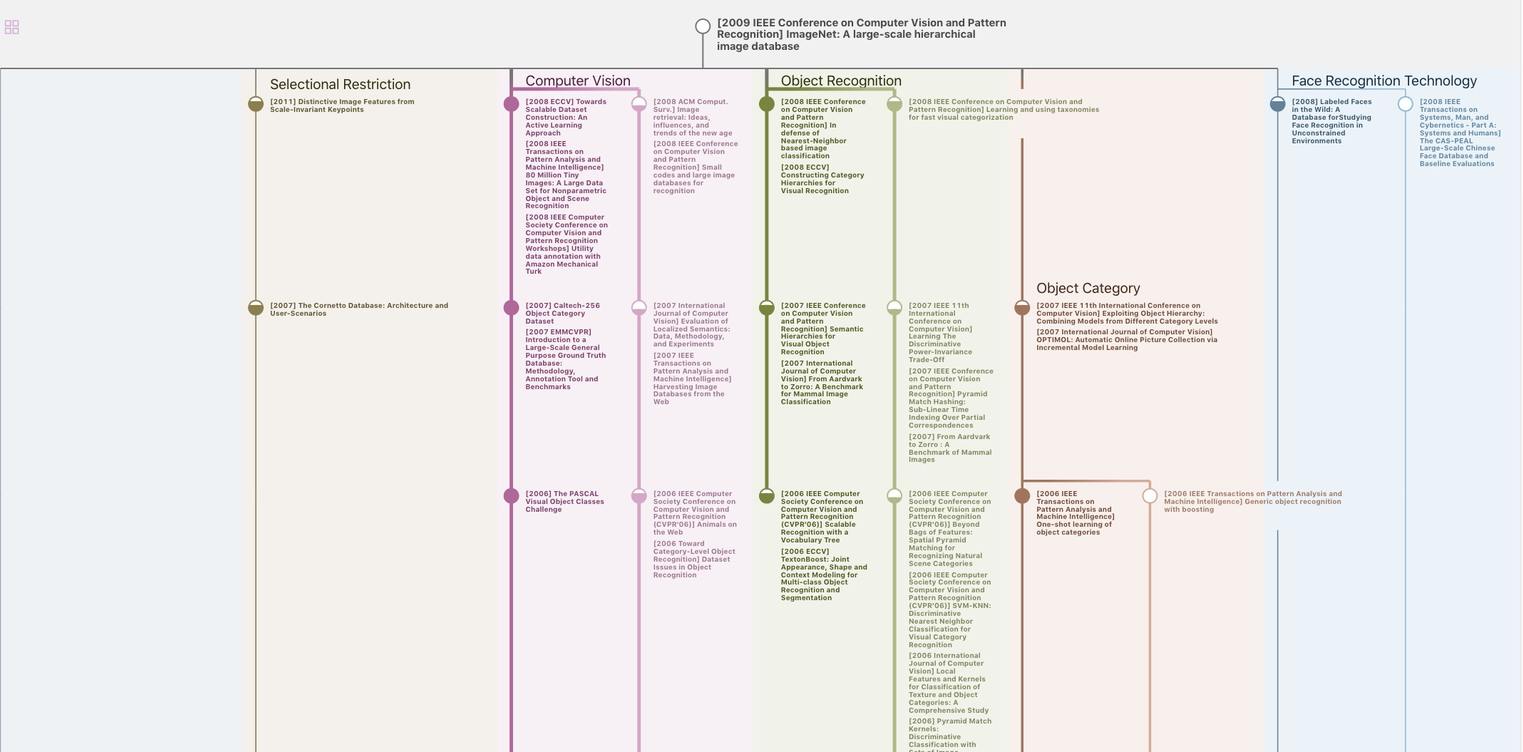
生成溯源树,研究论文发展脉络
Chat Paper
正在生成论文摘要