Large-scale dendritic spine extraction and analysis through petascale computing
biorxiv(2021)
摘要
The synapse is a central player in the nervous system serving as the key structure that permits the relay of electrical and chemical signals from one neuron to another. The anatomy of the synapse contains important information about the signals and the strength of signal it transmits. Because of their small size, however, electron microscopy (EM) is the only method capable of directly visualizing synapse morphology and remains the gold standard for studying synapse morphology. Historically, EM has been limited to small fields of view and often only in 2D, but recent advances in automated serial EM (“connectomics”) have enabled collecting large EM volumes that capture significant fractions of neurons and the different classes of synapses they receive (i.e. shaft, spine, soma, axon). However, even with recent advances in automatic segmentation methods, extracting neuronal and synaptic profiles from these connectomics datasets are difficult to scale over large EM volumes. Without methods that speed up automatic segmentation over large volumes, the full potential of utilizing these new EM methods to advance studies related to synapse morphologies will never be fully realized. To solve this problem, we describe our work to leverage Argonne leadership-scale supercomputers for segmentation of a 0.6 terabyte dataset using state of the art machine learning-based segmentation methods on a significant fraction of the 11.69 petaFLOPs supercomputer Theta at Argonne National Laboratory. We describe an iterative pipeline that couples human and machine feedback to produce accurate segmentation results in time frames that will make connectomics a more routine method for exploring how synapse biology changes across a number of biological conditions. Finally, we demonstrate how dendritic spines can be algorithmically extracted from the segmentation dataset for analysis of spine morphologies. Advancing this effort at large compute scale is expected to yield benefits in turnaround time for segmentation of individual datasets, accelerating the path to biology results and providing population-level insight into how thousands of synapses originate from different neurons; we expect to also reap benefits in terms of greater accuracy from the more compute-intensive algorithms these systems enable.
### Competing Interest Statement
The authors have declared no competing interest.
更多查看译文
关键词
dendritic spine extraction,large-scale
AI 理解论文
溯源树
样例
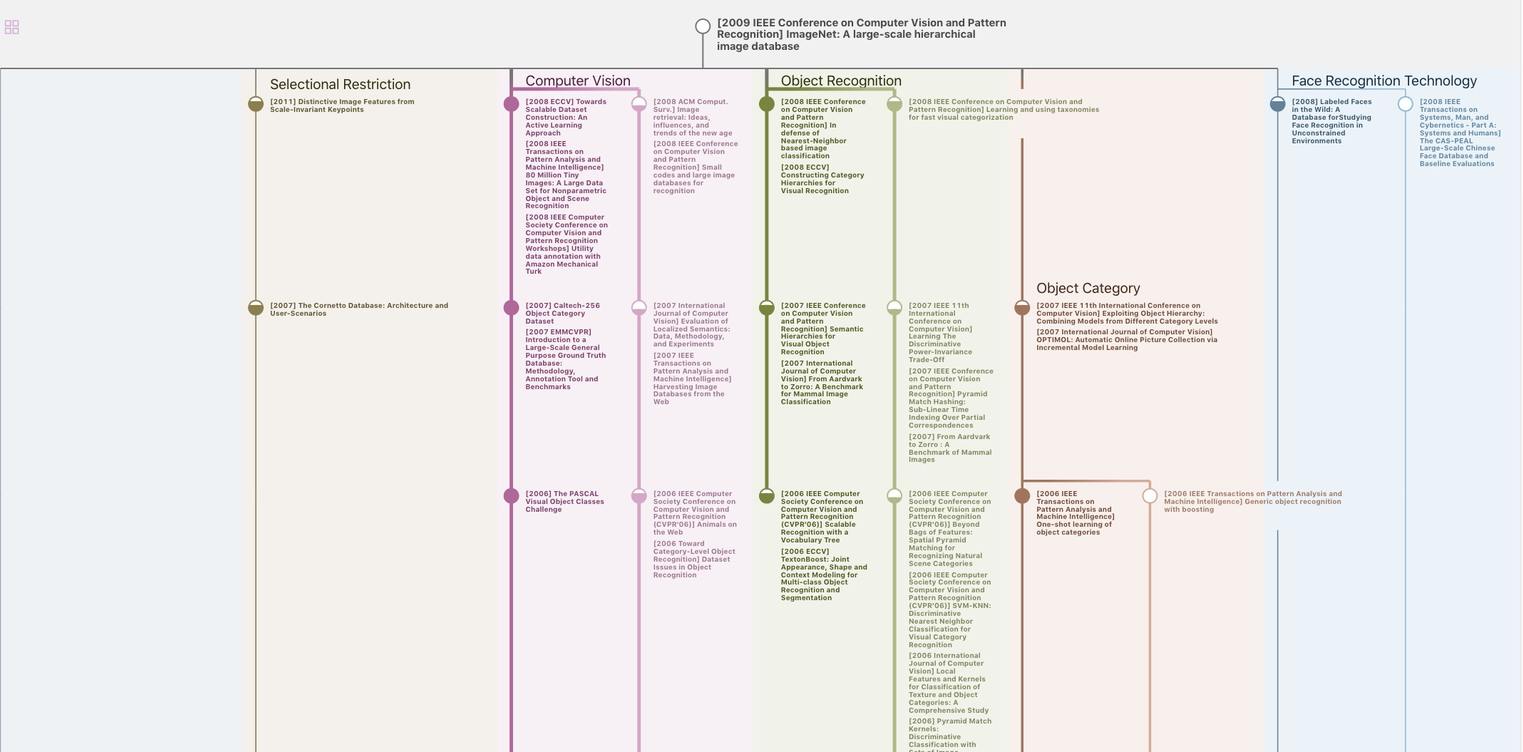
生成溯源树,研究论文发展脉络
Chat Paper
正在生成论文摘要