Quantitative Single-Molecule Imaging with Statistical Machine Learning
biorxiv(2021)
摘要
Single-molecule localization microscopy (SMLM) is a super-resolution technique capable of rendering nanometer scale images of cellular structures. Recently, much effort has gone into developing SMLM into a quantitative method capable of determining the abundance and stoichiometry of macromolecular complexes. These methods often require knowledge of the complex photophysical properties of photoswitchable flourophores. We previously developed a simpler method built upon the observation that most photswitchable fluorophores emit an exponentially distributed number of blinks before photobleaching, but its utility was limited by the need to calibrate for the blinking distribution. Here we extend this method by incorporating a machine learning technique known as Expectation-Maximization (EM) and apply it to a statistical mixture model of monomers, dimers and trimers. We show that the protomer fractions and the underlying single-fluorophore blinking distributions can be inferred, simultaneously, from SMLM datasets, obviating the need for an additional calibration and greatly expanding the applicability of this technique. To illustrate the utility of our approach, we benchmark the method on both simulated datasets and experimental datasets assembled from dSTORM images of Alexa-647 labeled DNA nanostructures.
### Competing Interest Statement
The authors have declared no competing interest.
更多查看译文
关键词
imaging,machine learning,single-molecule
AI 理解论文
溯源树
样例
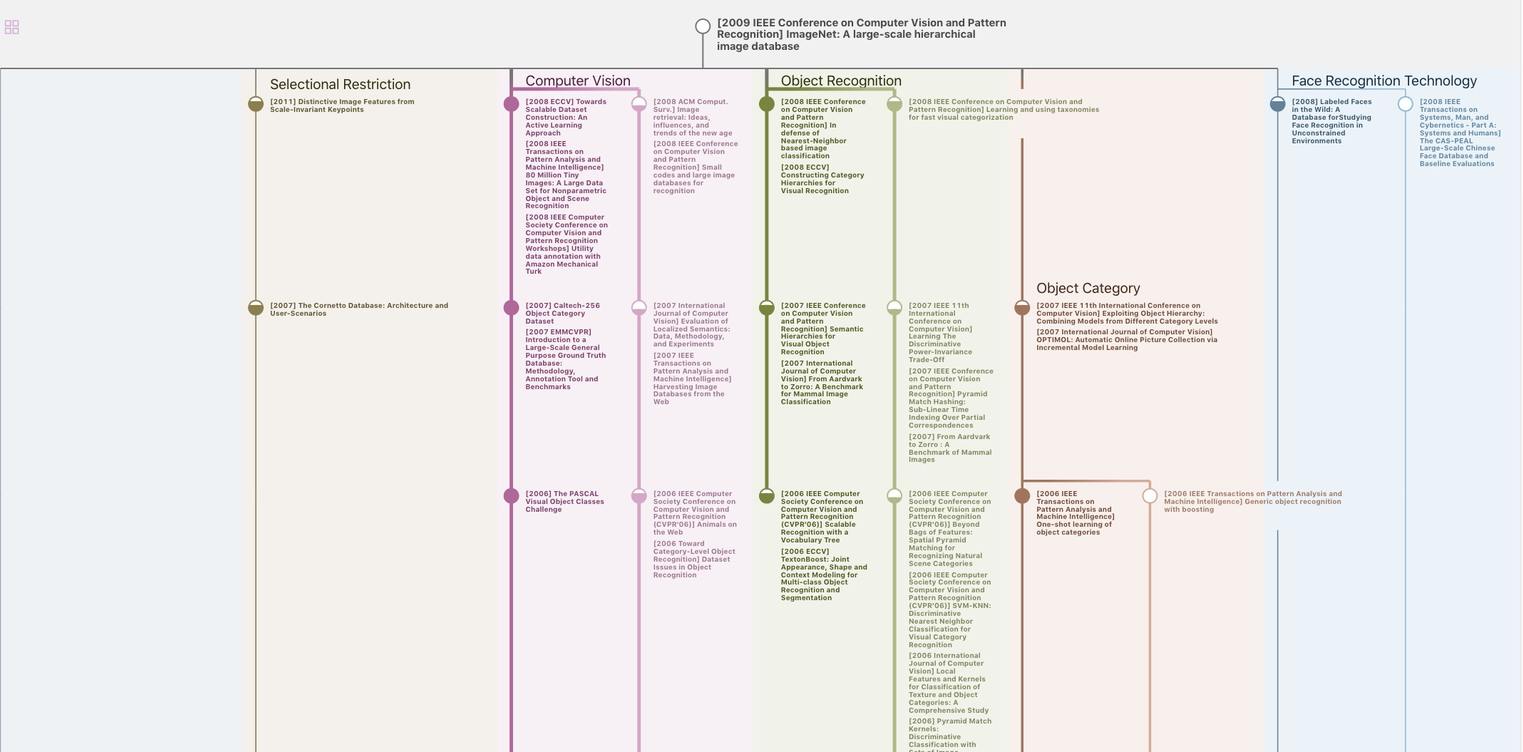
生成溯源树,研究论文发展脉络
Chat Paper
正在生成论文摘要