New land-usechange scenarios for Brazil: Refining global SSPs with a regional spatially-explicit allocation model
PLOS ONE(2022)
摘要
The future of land use and cover change in Brazil, particularly due to deforestation and forest restoration processes, is critical for the future of global climate and biodiversity, given the richness of its five biomes. These changes in Brazil depend on the interlink between global factors due to its role as one of the main exporters of commodities globally and the national to local institutional, socioeconomic, and biophysical contexts. Aiming to develop scenarios that consider the balance between global (e.g., GDP growth, population growth, per capita consumption of agricultural products, international trade policies, and climatic conditions) and local factors (e.g., land use, agrarian structure, agricultural suitability, protected areas, distance to roads, and other infrastructure projects), a new set of land-use change scenarios for Brazil were developed that aligned with the global structure Shared Socioeconomic Pathways (SSPs) and Representative Concentration Pathway (RCPs) developed by the global change research community. The narratives of the new scenarios align with SSP1/RCP 1.9 (Sustainable development scenario), SSP2/RCP 4.5 (Middle of the road scenario), and SSP3/RCP 7.0 (Strong inequality scenario). The scenarios were developed by combining the LuccME spatially explicit land change allocation modeling framework and the INLAND surface model to incorporate the climatic variables in water deficit. Based on detailed biophysical, socioeconomic, and institutional factors for each biome in Brazil, we have created spatially explicit scenarios until 2050, considering the following classes: forest vegetation, grassland vegetation, planted pasture, agriculture, a mosaic of small land uses, and forestry. The results aim to detail global models regionally. They could be used regionally to support decision-making and enrich the global analysis.
更多查看译文
关键词
global ssps,brazil,land,spatially-explicit
AI 理解论文
溯源树
样例
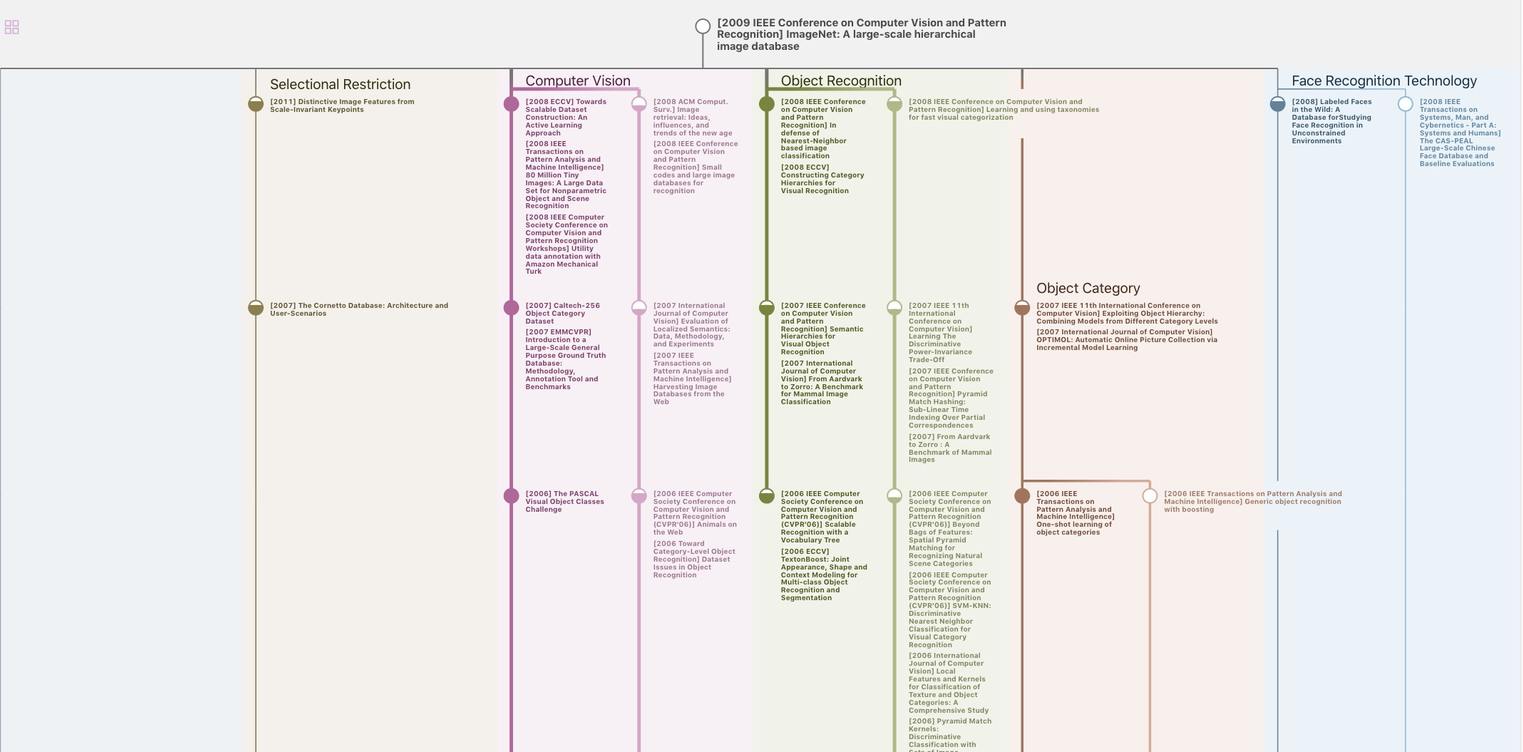
生成溯源树,研究论文发展脉络
Chat Paper
正在生成论文摘要