An approximate stochastic optimal control framework to simulate nonlinear neuro-musculoskeletal models in the presence of noise
PLOS COMPUTATIONAL BIOLOGY(2022)
摘要
Optimal control simulations have shown that both musculoskeletal dynamics and physiological noise are important determinants of movement. However, due to the limited efficiency of available computational tools, deterministic simulations of movement focus on accurately modelling the musculoskeletal system while neglecting physiological noise, and stochastic simulations account for noise while simplifying the dynamics. We took advantage of recent approaches where stochastic optimal control problems are approximated using deterministic optimal control problems, which can be solved efficiently using direct collocation. We were thus able to extend predictions of stochastic optimal control as a theory of motor coordination to include muscle coordination and movement patterns emerging from non-linear musculoskeletal dynamics. In stochastic optimal control simulations of human standing balance, we demonstrated that the inclusion of muscle dynamics can predict muscle co-contraction as minimal effort strategy that complements sensorimotor feedback control in the presence of sensory noise. In simulations of reaching, we demonstrated that nonlinear multi-segment musculoskeletal dynamics enables complex perturbed and unperturbed reach trajectories under a variety of task conditions to be predicted. In both behaviors, we demonstrated how interactions between task constraint, sensory noise, and the intrinsic properties of muscle influence optimal muscle coordination patterns, including muscle co-contraction, and the resulting movement trajectories. Our approach enables a true minimum effort solution to be identified as task constraints, such as movement accuracy, can be explicitly imposed, rather than being approximated using penalty terms in the cost function. Our approximate stochastic optimal control framework predicts complex features, not captured by previous simulation approaches, providing a generalizable and valuable tool to study how musculoskeletal dynamics and physiological noise may alter neural control of movement in both healthy and pathological movements. Author summary Model-based simulations have advanced our insight in how movement is controlled, but computational limitations have prevented us from simultaneously considering the effects of nonlinear musculoskeletal dynamics and noise on neural control on movement strategies. Here we present a novel simulation framework that addresses this methodological gap and demonstrate its potential to predict features of motor control that have not been predicted previously. Our framework enables neural control mechanisms to be simulated and the optimal control strategy in the presence of noise to be computed. We demonstrate for the first time that muscle co-contraction-a motor strategy thought to be energetically costly-can help to reduce the effort required to stabilize standing postural control, even when feedback is present. We also demonstrate that multi-segmental models of the arm actuated by muscle can predict complex reach and perturbed reach trajectories that were not explained by simplified models. The ability to predict muscle activation patterns and kinematics of joints extends the theory of optimal control to variables that can be used to directly explain muscle and joint coordination. Our computational approach is broadly applicable and may be extendable to other normal and impaired movements, as well as aid in the design of assistive devices.
更多查看译文
AI 理解论文
溯源树
样例
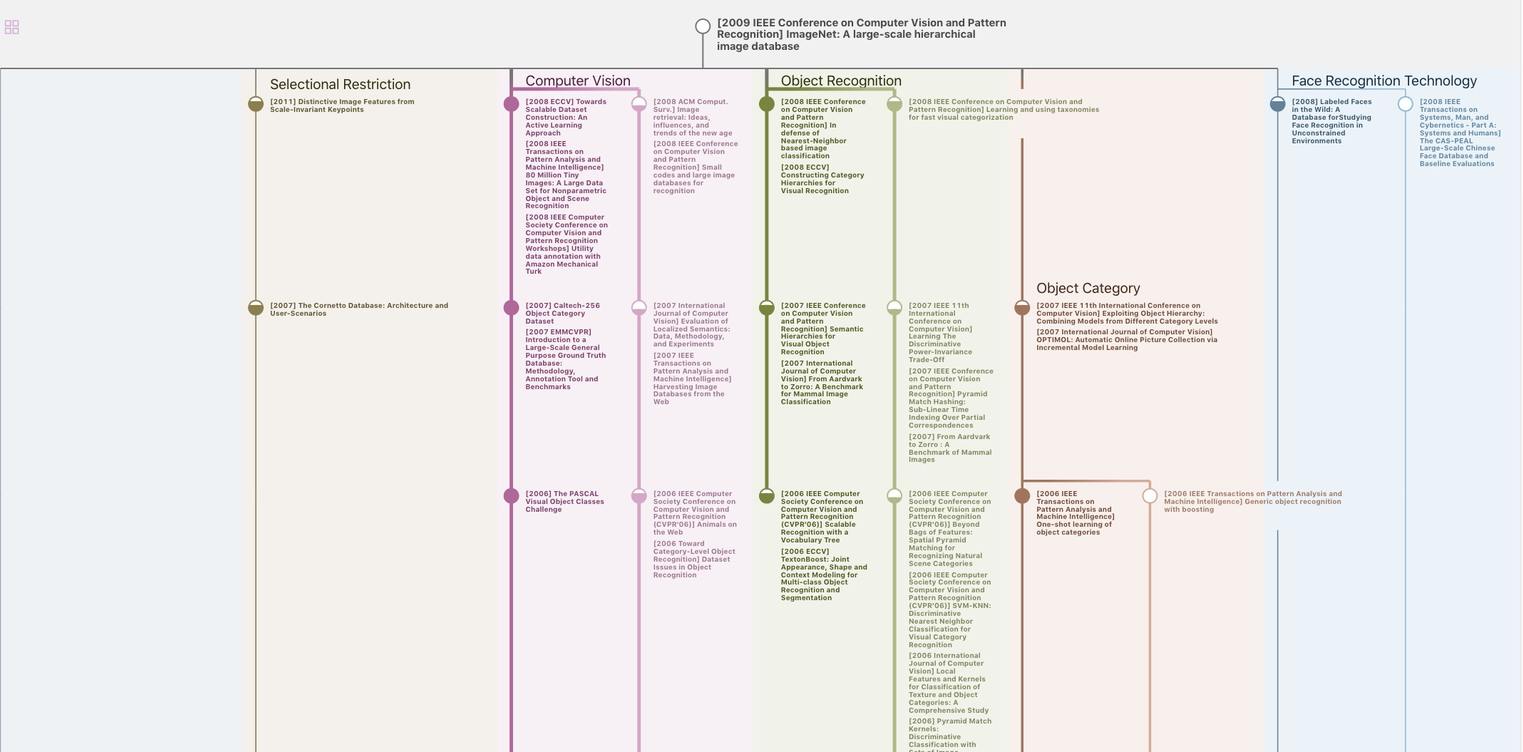
生成溯源树,研究论文发展脉络
Chat Paper
正在生成论文摘要