Deep learning features encode interpretable morphologies within histological images
SCIENTIFIC REPORTS(2022)
摘要
Convolutional neural networks (CNNs) are revolutionizing digital pathology by enabling machine learning-based classification of a variety of phenotypes from hematoxylin and eosin (H E) whole slide images (WSIs), but the interpretation of CNNs remains difficult. Most studies have considered interpretability in a post hoc fashion, e.g. by presenting example regions with strongly predicted class labels. However, such an approach does not explain the biological features that contribute to correct predictions. To address this problem, here we investigate the interpretability of H E-derived CNN features (the feature weights in the final layer of a transfer-learning-based architecture). While many studies have incorporated CNN features into predictive models, there has been little empirical study of their properties. We show such features can be construed as abstract morphological genes (“mones”) with strong independent associations to biological phenotypes. Many mones are specific to individual cancer types, while others are found in multiple cancers especially from related tissue types. We also observe that mone-mone correlations are strong and robustly preserved across related cancers. Importantly, linear mone-based classifiers can very accurately separate 38 distinct classes (19 tumor types and their adjacent normals, AUC = 97.1%± 2.8% for each class prediction), and linear classifiers are also highly effective for universal tumor detection (AUC = 99.2%± 0.12% ). This linearity provides evidence that individual mones or correlated mone clusters may be associated with interpretable histopathological features or other patient characteristics. In particular, the statistical similarity of mones to gene expression values allows integrative mone analysis via expression-based bioinformatics approaches. We observe strong correlations between individual mones and individual gene expression values, notably mones associated with collagen gene expression in ovarian cancer. Mone-expression comparisons also indicate that immunoglobulin expression can be identified using mones in colon adenocarcinoma and that immune activity can be identified across multiple cancer types, and we verify these findings by expert histopathological review. Our work demonstrates that mones provide a morphological H E decomposition that can be effectively associated with diverse phenotypes, analogous to the interpretability of transcription via gene expression values. Our work also demonstrates mones can be interpreted without using a classifier as a proxy.
更多查看译文
关键词
Computational biology and bioinformatics,Computational models,Image processing,Machine learning,Statistical methods,Science,Humanities and Social Sciences,multidisciplinary
AI 理解论文
溯源树
样例
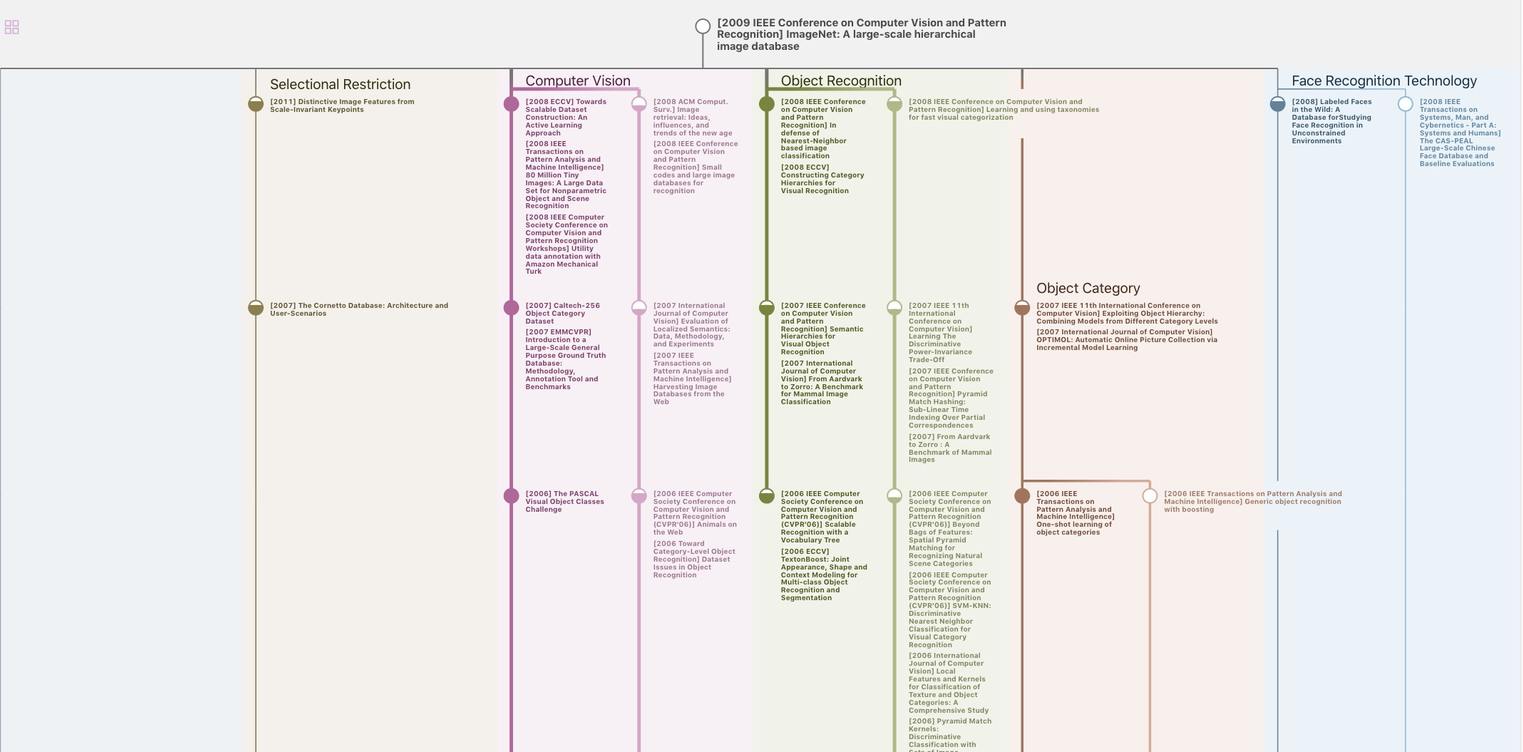
生成溯源树,研究论文发展脉络
Chat Paper
正在生成论文摘要