Clustering single cell CITE-seq data with a canonical correlation based deep learning method
biorxiv(2021)
摘要
Single cell sequencing examines the sequence information from individual cells with optimized next generation sequencing (NGS) technologies. It provides researchers a higher resolution of cellular differences and a better understanding of the function of an individual cell in the context of its microenvironment. CITE-seq, or Cellular Indexing of Transcriptomes and Epitopes by sequencing, is one of the latest innovations in the domain of single cell sequencing. It enables researchers to simultaneously capture RNA and surface protein expression on the same cells so that we can correlate the two data types, identify biomarkers and better characterize cell phenotypes. Although multi-omics sequencing technologies developed rapidly, data analyzing methods tailored for multiomics sequencing data are lacking. Several serious problems have to be faced. An important one is how to integrate the information from different modalities, namely scRNA and protein data, efficiently.
In this paper, we introduce a canonical correlation based deep learning method called scCTClust for clustering analysis over CITE-seq data. We impute and extract the characteristics of the high dimensional RNA part of data with a ZINB model-based autoencoder. A t-kernel distance is introduced to measure the similarity between query cell and cluster centroids. And the protein data rectifies the feature extraction of scRNA data in a canonical correlation analysis(CCA) way. Extensive data experiments shows that scCTClust can precisely recover the dropout values for RNA sequencing data and extract authentic information from both modalities, getting a much better clustering result than state-of-the-art methods, no matter single-omic or multi-omics clustering algorithms.
### Competing Interest Statement
The authors have declared no competing interest.
更多查看译文
关键词
canonical correlation,clustering,deep learning,cite-seq
AI 理解论文
溯源树
样例
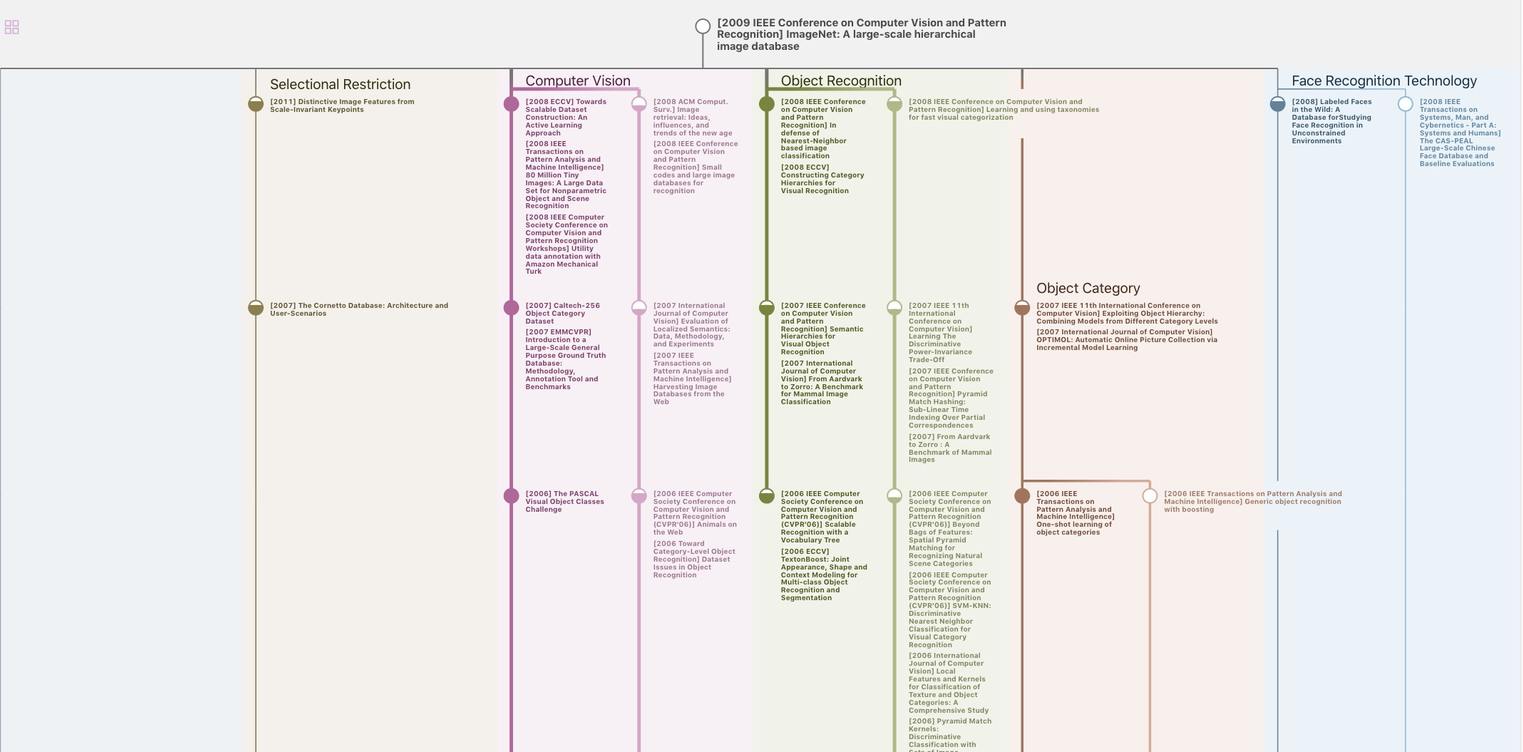
生成溯源树,研究论文发展脉络
Chat Paper
正在生成论文摘要