Convex-PLR – Revisiting affinity predictions and virtual screening using physics-informed machine learning
bioRxiv(2021)
摘要
Virtual screening is an essential part of the modern drug design pipeline, which significantly accelerates the discovery of new drug candidates. Structure-based virtual screening involves ligand conformational sampling, which is often followed by re-scoring of docking poses. A great variety of scoring functions have been designed for this purpose. The advent of structural and affinity databases and the progress in machine-learning methods have recently boosted scoring function performance. Nonetheless, the most successful scoring functions are typically designed for specific tasks or systems. All-purpose scoring functions still perform poorly on the virtual screening tests, compared to precision with which they are able to predict co-crystal binding poses. Another limitation is the low interpretability of the heuristics being used.
We analyzed scoring functions’ performance in the CASF benchmarks and discovered that the vast majority of them have a strong bias towards predicting larger binding interfaces. This motivated us to develop a physical model with additional entropic terms with the aim of penalizing such a preference. We parameterized the new model using affinity and structural data, solving a classification problem followed by regression. The new model, called Convex-PL R , demonstrated high-quality results on multiple tests and a substantial improvement over its predecessor Convex-PL. Convex-PL R can be used for molecular docking together with VinaCPL, our version of AutoDock Vina, with Convex-PL integrated as a scoring function. Convex-PL R , Convex-PL, and VinaCPL are available at .
### Competing Interest Statement
The authors have declared no competing interest.
* CASF
: Comparative Assessment of Scoring Functions
RMSD
: Root Mean Squared Deviation
SVM
: Support Vector Machinesn
PDB
: Protein Data Bankn
SAS
: Solvent-Accessible Surfacen
SASA
: Solvent-Accessible Surface Area
更多查看译文
关键词
affinity predictions,virtual screening,machine learning,convex-pl-r,physics-informed
AI 理解论文
溯源树
样例
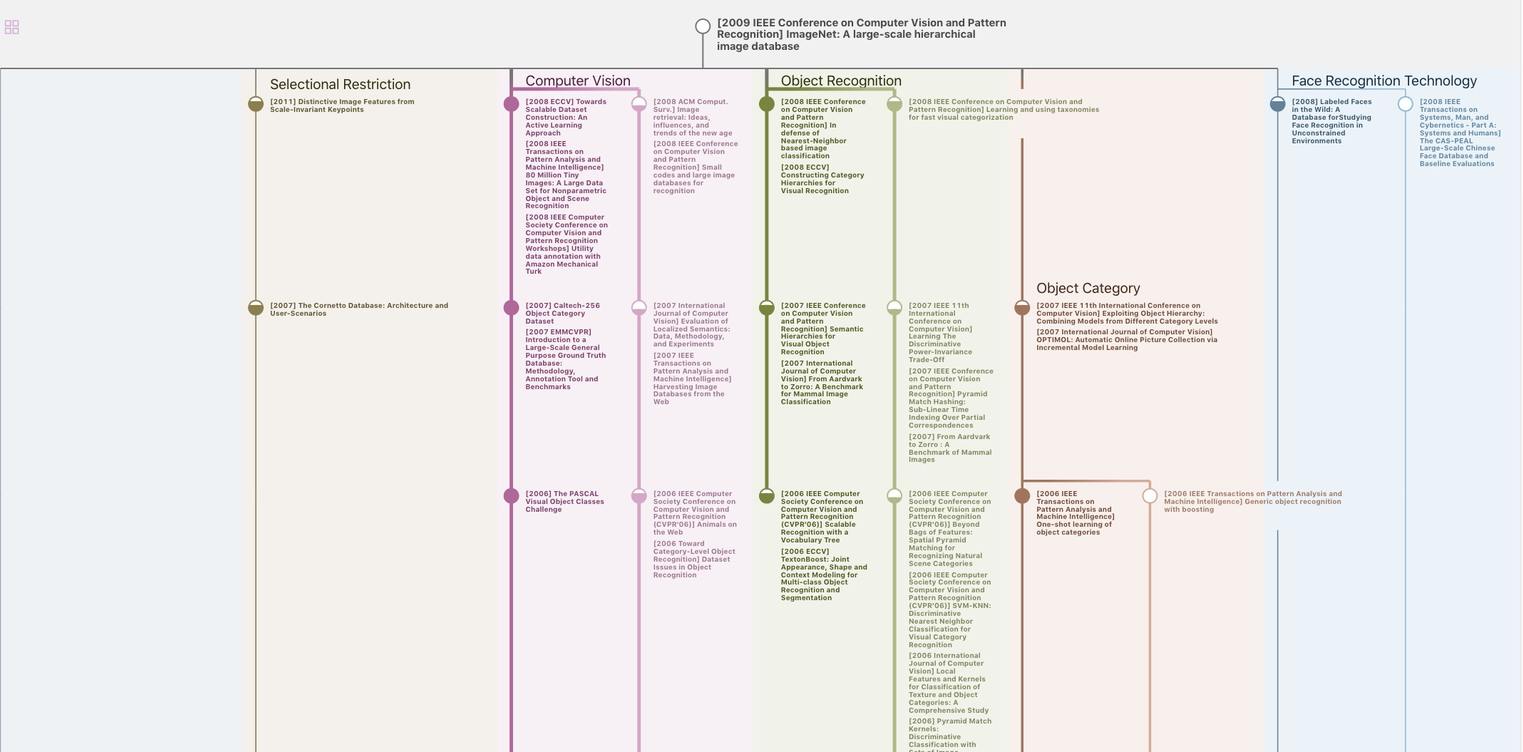
生成溯源树,研究论文发展脉络
Chat Paper
正在生成论文摘要