Expert-integrated automated machine learning uncovers hemodynamic predictors in spinal cord injury
PLOS ONE(2021)
摘要
Automated machine learning (AutoML) is positioned to democratize artificial intelligence (AI) by reducing the amount of human input and ML expertise needed to create prediction models. However, successful translation of ML in biomedicine requires moving beyond optimizing only for prediction accuracy and towards discovering reproducible clinical and biological inferences. Here, we present a model-agnostic framework to reinforce AutoML using strategies and tools of explainable and reproducible AI, including novel metrics for performance precision and feature instability. The framework enables clinicians to interpret AutoML-generated models for clinical and biological verifiability and consequently integrate domain expertise during model development. We applied the framework towards spinal cord injury prognostication and identified a detrimental relationship between intraoperative hypertension and patient outcome. Furthermore, our analysis captured evolving clinical practices such as faster time-to-surgery and blood pressure management that affected clinical model validation. Altogether, we illustrate how augmenting AutoML for inferential reproducibility empowers biomedical discovery and builds trust in AI processes towards effective clinical integration.
### Competing Interest Statement
SK, JS, JH, AL, RS, and CM are current employees of DataRobot and own shares of the company. Access to the DataRobot Automated Machine Learning platform was awarded through application and selection by the DataRobot AI for Good program. DataRobot affiliated authors provided editorial contributions during the preparation of the manuscript. All other authors declare no competing financial interests.
更多查看译文
AI 理解论文
溯源树
样例
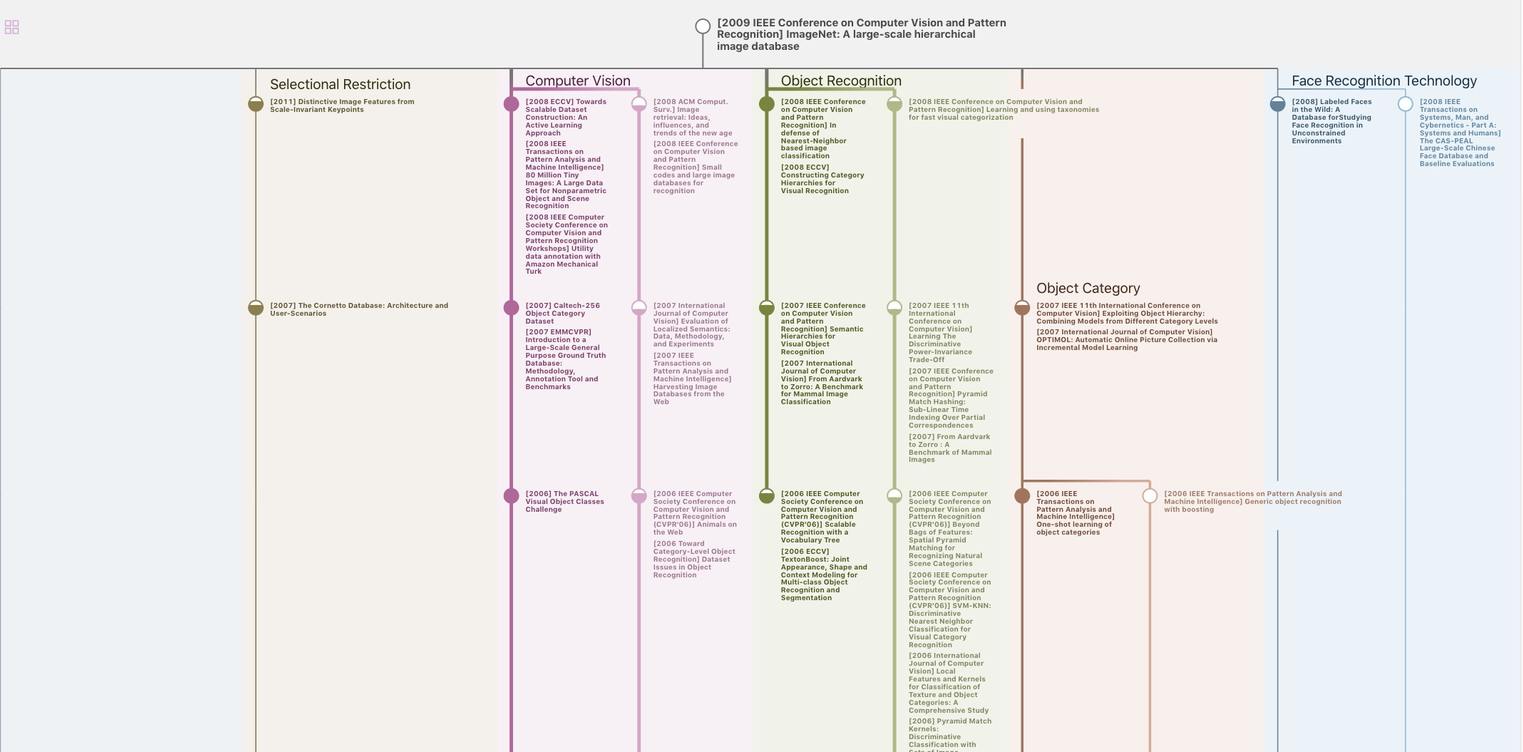
生成溯源树,研究论文发展脉络
Chat Paper
正在生成论文摘要