Threshold Selection for Brain Connectomes
bioRxiv (Cold Spring Harbor Laboratory)(2022)
摘要
Introduction Structural and functional brain connectomes built using macroscale data collected through magnetic resonance imaging (MRI) may contain noise that contributes to false-positive edges, which can obscure structure-function relationships with implications for data interpretation. Thresholding procedures are routinely applied in practice to optimize network density by removing low-signal edges, but there is limited consensus regarding the appropriate selection of thresholds. We compare existing methods and propose a novel alternative objective function thresholding (OFT) method.
Methods The performance of thresholding approaches, including a percolation-based approach and an objective function-based approach, is assessed by (a) computing the normalized mutual information (NMI) of community structure between a known network and a simulated, perturbed networks to which various forms of thresholding have been applied, and (b) comparing the density and the clustering coefficient (CC) between the baseline and thresholded networks.
Results In our analysis, the proposed objective function-based threshold exhibits the best performance in terms of high similarity between the underlying networks and their perturbed, thresholded counterparts, as quantified by NMI and CC analysis on the simulated functional networks.
Discussion Existing network thresholding methods yield widely different results when graph metrics are computed. Thresholding based on the objective function appears to maintain a set of edges such that the resulting network shares the community structure and clustering features present in the original network. This outcome provides proof-of-principle evidence that thresholding based on the objective function could offer a useful approach to reducing the network density of functional connectivity data.
Impact Statement Network thresholding refers to removing edges between node pairs in a functional network that have weak edge-weights that may arise from unwanted variability or noise. Since edge-weight cutoffs used to generate a binary network can be sensitive to thresholding, we introduce a novel thresholding algorithm. We find that when applied to networks derived via perturbations, namely through simulated functional connectivity of a known network, this approach yields a binary network that is more similar to the known network compared to using existing thresholding approaches. Thus, our algorithm is a competitive candidate for use in thresholding of brain connectome.
### Competing Interest Statement
The authors have declared no competing interest.
* MRI
: magnetic resonance image
ROI
: region of interest
SC
: structural connectome
FC
: functional connectome
GCC
: giant connected component
LFR
: Lancichinetti-Fortunato-Radicchi
LFR-sf
: the scale free LRF networks
NMI
: normalized mutual information
更多查看译文
关键词
brain,threshold,selection
AI 理解论文
溯源树
样例
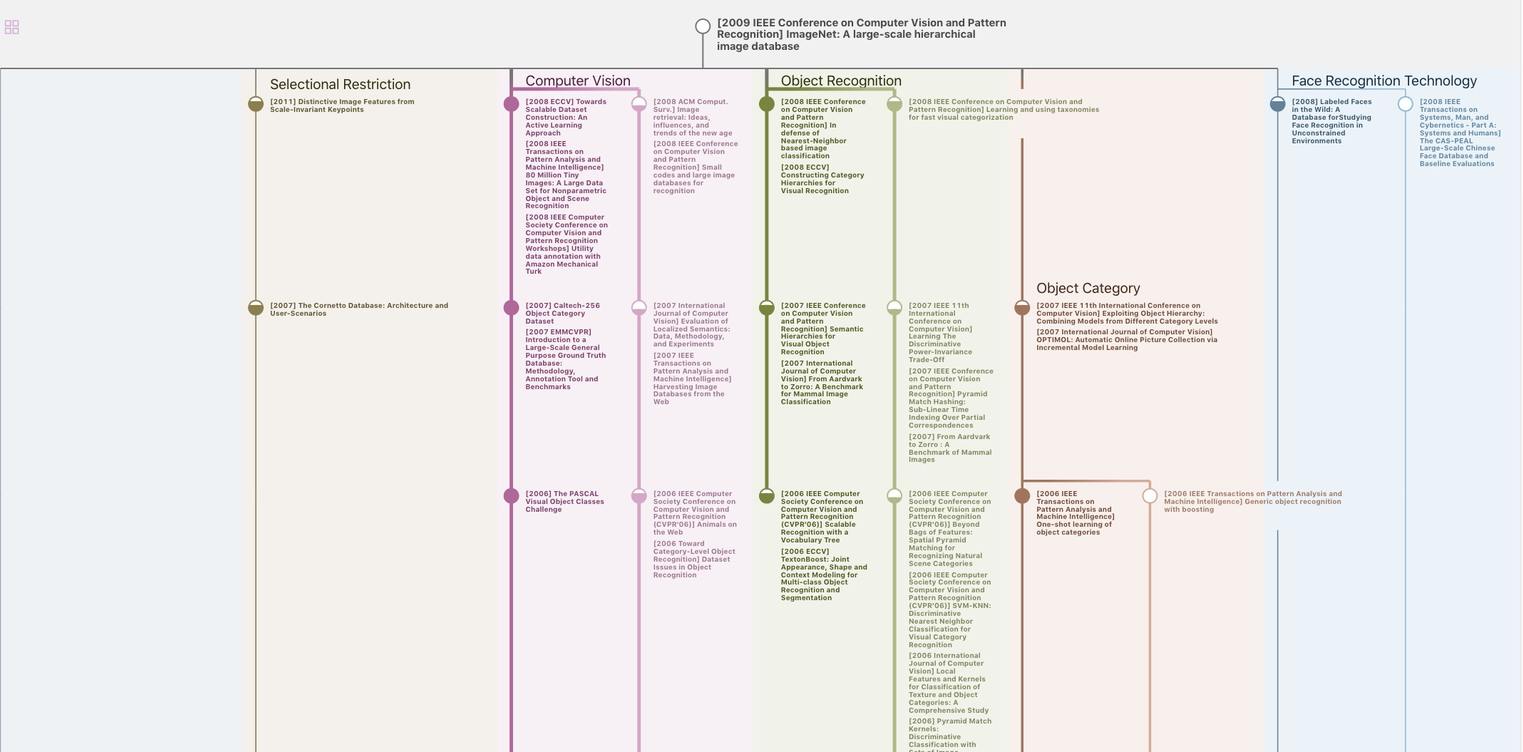
生成溯源树,研究论文发展脉络
Chat Paper
正在生成论文摘要