Disentangled Inference for GANs With Latently Invertible Autoencoder
International Journal of Computer Vision(2022)
摘要
Generative Adversarial Networks (GANs) can synthesize more and more realistic images. However, one fundamental issue hinders their practical applications: the incapability of encoding real samples in the latent space. Many semantic image editing applications rely on inverting the given image into the latent space and then manipulating inverted code. One possible solution is to learn an encoder for GAN via Variational Auto-Encoder. However, the entanglement of the latent space poses a major challenge for learning the encoder. To tackle the challenge and enable inference in GANs, we propose a novel method named Latently Invertible Autoencoder (LIA). In LIA, an invertible network and its inverse mapping are symmetrically embedded in the latent space of an autoencoder. The decoder of LIA is first trained as a standard GAN with the invertible network, and then the encoder is learned from a disentangled autoencoder by detaching the invertible network from LIA. It thus avoids the entanglement problem caused by the latent space. Extensive experiments on the FFHQ face dataset and three LSUN datasets validate the effectiveness of LIA for the image inversion and its applications. Code and models are available at https://github.com/genforce/lia .
更多查看译文
关键词
GAN, VAE, Inference, Disentanglement
AI 理解论文
溯源树
样例
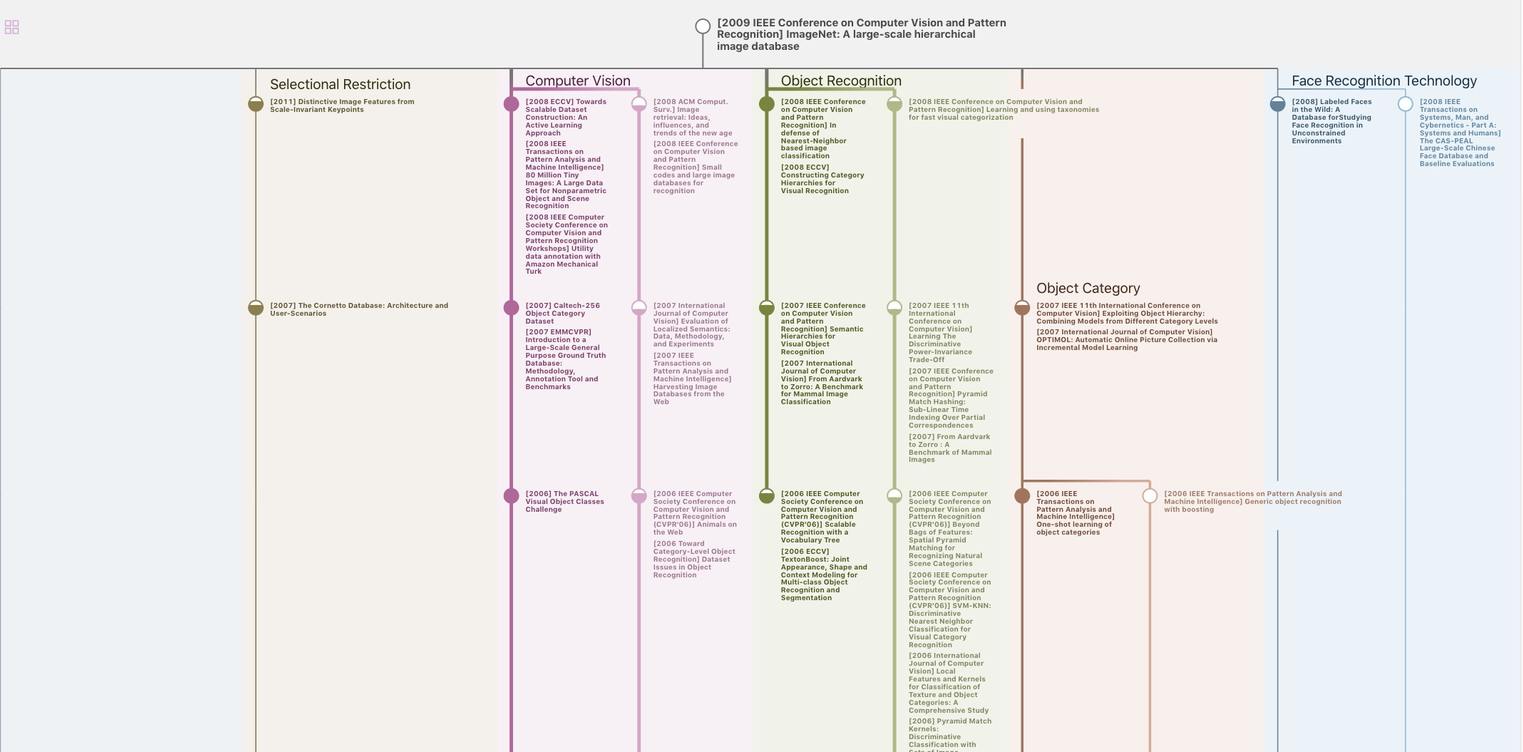
生成溯源树,研究论文发展脉络
Chat Paper
正在生成论文摘要