Faster $p$-Norm Regression Using Sparsity
arxiv(2021)
摘要
For a matrix $A\in \mathbb{R}^{n\times d}$ with $n\geq d$, we consider the dual problems of $\min \|Ax-b\|_p^p, \, b\in \mathbb{R}^n$ and $\min_{A^\top x=b} \|x\|_p^p,\, b\in \mathbb{R}^d$. We improve the runtimes for solving these problems to high accuracy for every $p>1$ for sufficiently sparse matrices. We show that recent progress on fast sparse linear solvers can be leveraged to obtain faster than matrix-multiplication algorithms for any $p > 1$, i.e., in time $\tilde{O}(pn^\theta)$ for some $\theta < \omega$, the matrix multiplication constant. We give the first high-accuracy input sparsity $p$-norm regression algorithm for solving $\min \|Ax-b\|_p^p$ with $1 < p \leq 2$, via a new row sampling theorem for the smoothed $p$-norm function. This algorithm runs in time $\tilde{O}(\text{nnz}(A) + d^4)$ for any $1
更多
查看译文
AI 理解论文
溯源树
样例
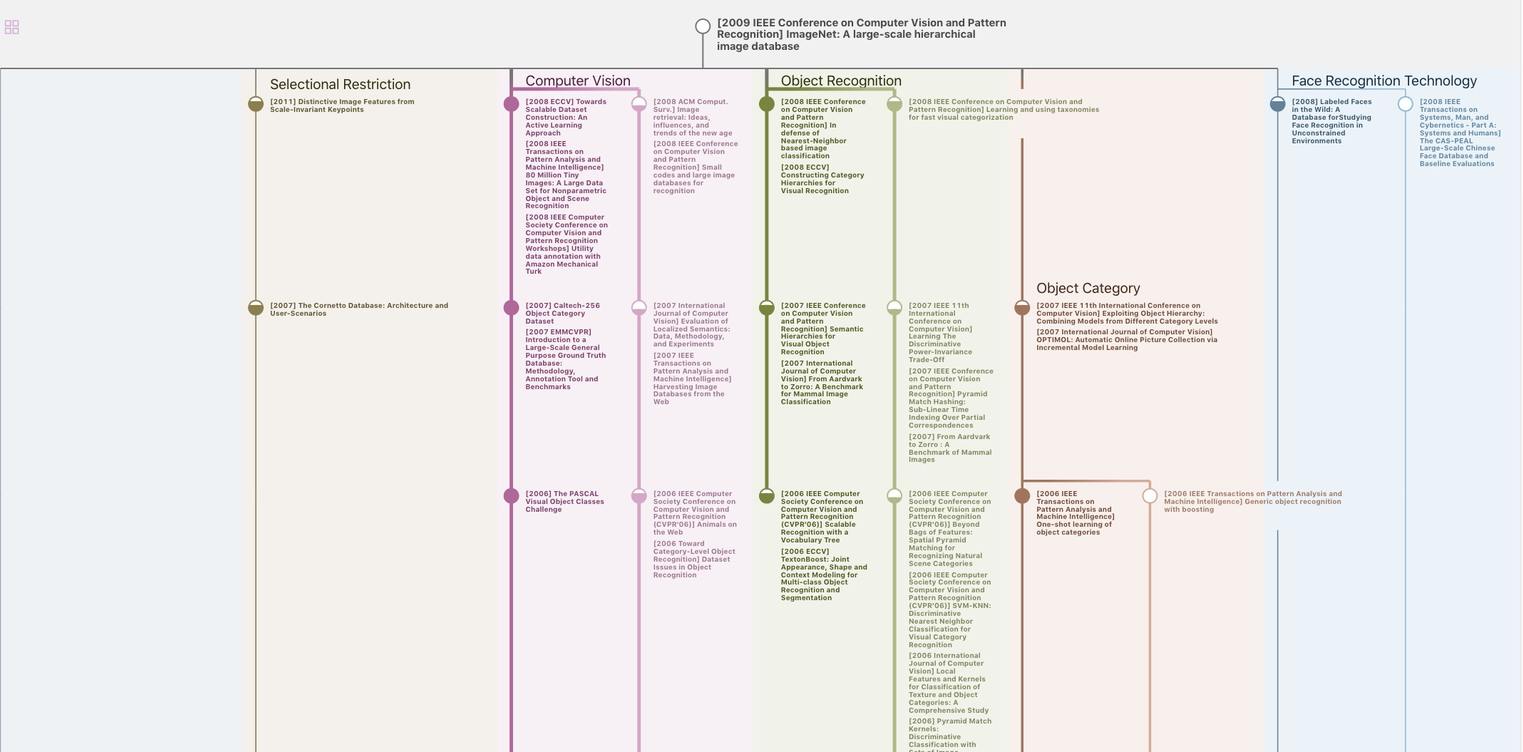
生成溯源树,研究论文发展脉络
Chat Paper
正在生成论文摘要