Learning To Estimate Regions Of Attraction Of Autonomous Dynamical Systems Using Physics-Informed Neural Networks
arxiv(2021)
摘要
When learning to perform motor tasks in a simulated environment, neural networks must be allowed to explore their action space to discover new potentially viable solutions. However, in an online learning scenario with physical hardware, this exploration must be constrained by relevant safety considerations in order to avoid damage to the agent's hardware and environment. We aim to address this problem by training a neural network, which we will refer to as a "safety network", to estimate the region of attraction (ROA) of a controlled autonomous dynamical system. This safety network can thereby be used to quantify the relative safety of proposed control actions and prevent the selection of damaging actions. Here we present our development of the safety network by training an artificial neural network (ANN) to represent the ROA of several autonomous dynamical system benchmark problems. The training of this network is predicated upon both Lyapunov theory and neural solutions to partial differential equations (PDEs). By learning to approximate the viscosity solution to a specially chosen PDE that contains the dynamics of the system of interest, the safety network learns to approximate a particular function, similar to a Lyapunov function, whose zero level set is boundary of the ROA. We train our safety network to solve these PDEs in a semi-supervised manner following a modified version of the Physics Informed Neural Network (PINN) approach, utilizing a loss function that penalizes disagreement with the PDE's initial and boundary conditions, as well as non-zero residual and variational terms. In future work we intend to apply this technique to reinforcement learning agents during motor learning tasks.
更多查看译文
关键词
autonomous dynamical systems,neural networks,estimate regions,learning,physics-informed
AI 理解论文
溯源树
样例
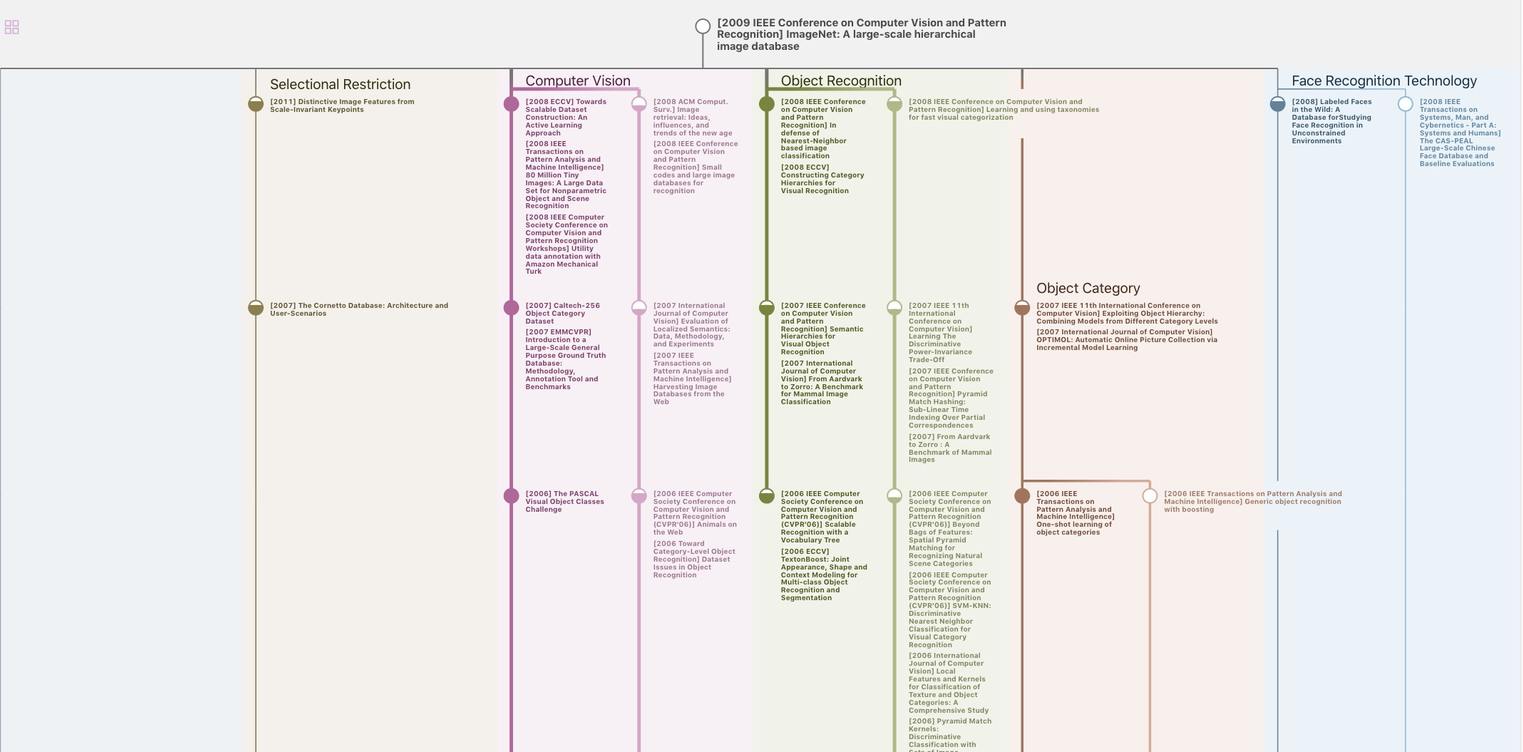
生成溯源树,研究论文发展脉络
Chat Paper
正在生成论文摘要