Composite Goodness-of-fit Tests with Kernels
arxiv(2021)
摘要
Model misspecification can create significant challenges for the implementation of probabilistic models, and this has led to development of a range of inference methods which directly account for this issue. However, whether these more involved methods are required will depend on whether the model is really misspecified, and there is a lack of generally applicable methods to answer this question. One set of tools which can help are goodness-of-fit tests, where we test whether a dataset could have been generated by a fixed distribution. Kernel-based tests have been developed to for this problem, and these are popular due to their flexibility, strong theoretical guarantees and ease of implementation in a wide range of scenarios. In this paper, we extend this line of work to the more challenging composite goodness-of-fit problem, where we are instead interested in whether the data comes from any distribution in some parametric family. This is equivalent to testing whether a parametric model is well-specified for the data.
更多查看译文
关键词
goodness-of-fit
AI 理解论文
溯源树
样例
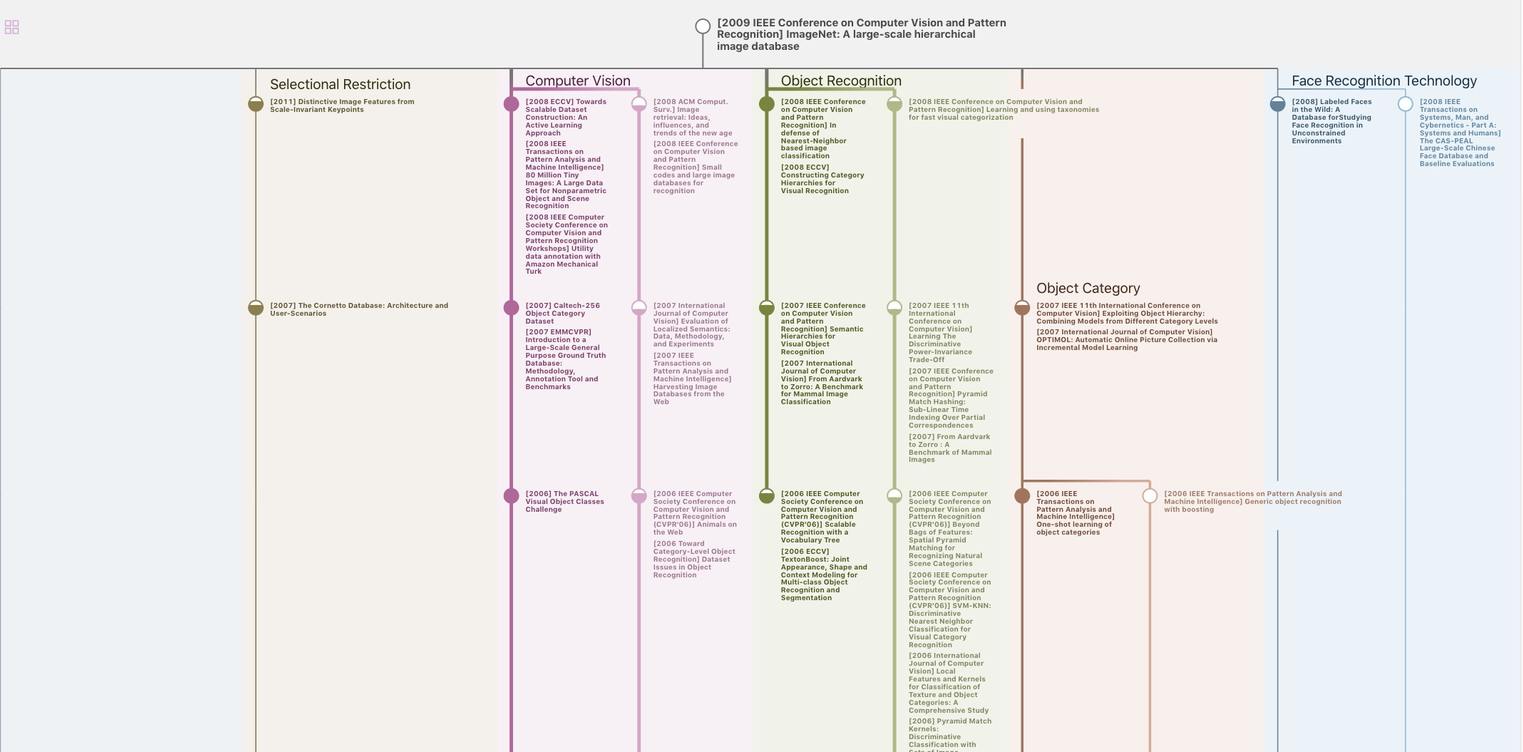
生成溯源树,研究论文发展脉络
Chat Paper
正在生成论文摘要