Coalition Situational Understanding via Explainable Neuro-Symbolic Reasoning and Learning
ARTIFICIAL INTELLIGENCE AND MACHINE LEARNING FOR MULTI-DOMAIN OPERATIONS APPLICATIONS III(2021)
摘要
Achieving coalition situational understanding (CSU) involves both insight, i.e., recognising existing situations, and foresight, i.e., learning and reasoning to draw inferences about those situations, exploiting assets from across a coalition, including sensor feeds of various modalities, and analytic services. Recent years have seen significant advances in artificial intelligence (AI) and machine learning (ML) technologies applicable to CSU. However, state-of-the-art ML techniques based on deep neural networks require large volumes of training data; unfortunately, representative training examples of situations of interest in CSU are usually sparse. Moreover, to be useful, ML-based analytic services cannot be 'black boxes;' they must be capable of explaining their outputs. In this paper we describe an integrated CSU architecture that combines deep neural networks with symbolic learning and reasoning to address the problem of sparse training data. We also demonstrate how explainability can be achieved for deep neural networks operating on multimodal sensor feeds. We also show how the combined neuro-symbolic system achieves a layered approach to explainability. The work focuses on real-time decision making settings at the tactical edge, with both the symbolic and neural network parts of the system-including the explainabilty approaches-able to deal with temporal features.
更多查看译文
关键词
situational understanding, coalition, artificial intelligence, machine learning, machine reasoning, explainability
AI 理解论文
溯源树
样例
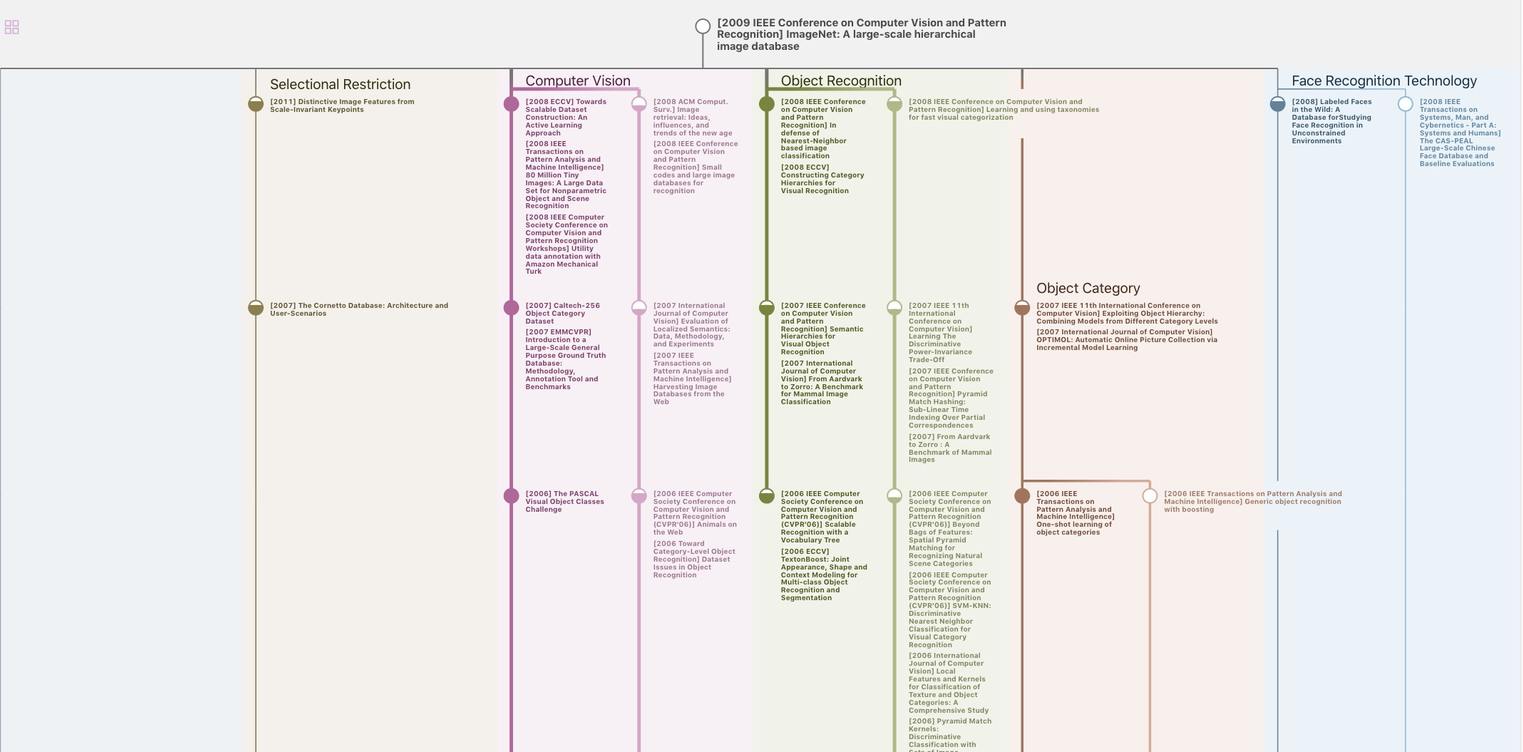
生成溯源树,研究论文发展脉络
Chat Paper
正在生成论文摘要