Automated Identification of Epileptic Seizures from EEG Signals Using FBSE-EWT Method
BIOMEDICAL SIGNAL PROCESSING: ADVANCES IN THEORY, ALGORITHMS AND APPLICATIONS(2020)
摘要
Epilepsy is a neurological disorder that leads to the occurrence of recurrent seizures. The electroencephalogram (EEG) signal is commonly used to record the electrical functioning from the brain. These recorded EEG signals have non-stationary and non-linear characteristics. In this chapter, we have introduced a new methodology based on Fourier-Bessel series expansion (FBSE) and empirical wavelet transform (EWT) for the classification of epileptic seizure EEG signals. The scale-space representation based detection of boundaries has been adapted for the segmentation of the FBSE spectrum obtained with EEG signals, and the EWT is utilized to obtain narrow sub-band signals. Then, the Hilbert marginal spectrum (HMS) of these sub-band signals have been obtained with FBSE-EWT. Afterwards, the line length and entropy features have been computed from obtained HMSs corresponding to different oscillatory levels of the EEG signals. To reduce the feature space, the Kruskal-Wallis test based feature ranking is applied. The selected features after feature ranking are used in random forest (RF) classifier for classifying normal from healthy subjects and seizure from epileptic subjects using EEG signals. The classification is also validated with 10-fold cross-validation and 50% training-50% testing data techniques. In addition to these techniques, the 5-fold cross-validation and 40% training-60% testing data techniques have been also applied on the significant features corresponding to maximum classification accuracies obtained with 10-fold cross-validation and 50% training-50% testing data techniques in order to reduce computational complexity. The assessment of classification performance is also evaluated in terms of classification accuracy for different sample lengths of EEG signals. The obtained maximum classification accuracy in this proposed method is 100%, with 50% training-50% testing data technique. The proposed method may help the neurologists for the identification of healthy and epileptic subjects from EEG signals.
更多查看译文
AI 理解论文
溯源树
样例
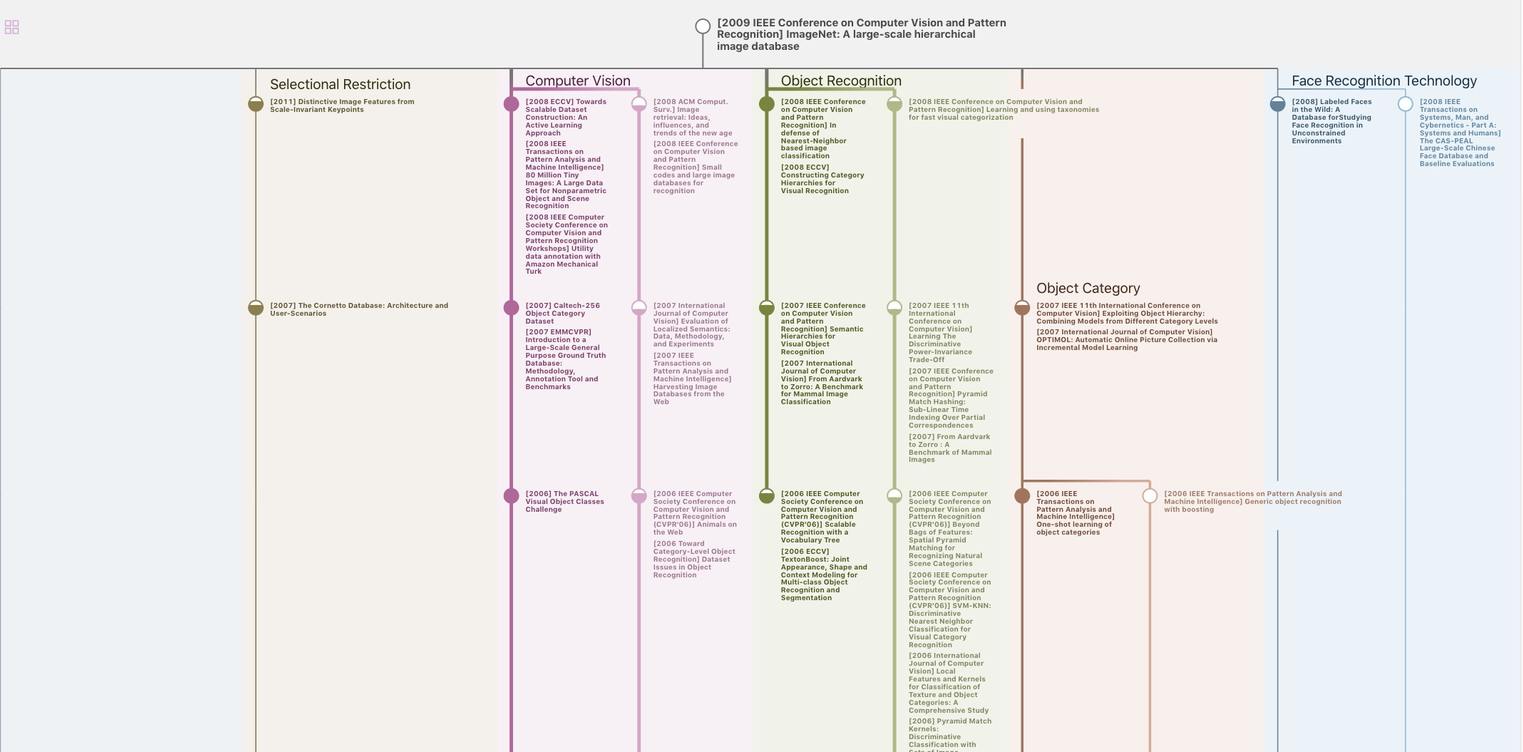
生成溯源树,研究论文发展脉络
Chat Paper
正在生成论文摘要