Prioritised Learning In Snowdrift-Type Games
MATHEMATICS(2020)
摘要
Cooperation is a ubiquitous and beneficial behavioural trait despite being prone to exploitation by free-riders. Hence, cooperative populations are prone to invasions by selfish individuals. However, a population consisting of only free-riders typically does not survive. Thus, cooperators and free-riders often coexist in some proportion. An evolutionary version of a Snowdrift Game proved its efficiency in analysing this phenomenon. However, what if the system has already reached its stable state but was perturbed due to a change in environmental conditions? Then, individuals may have to re-learn their effective strategies. To address this, we consider behavioural mistakes in strategic choice execution, which we refer to as incompetence. Parametrising the propensity to make such mistakes allows for a mathematical description of learning. We compare strategies based on their relative strategic advantage relying on both fitness and learning factors. When strategies are learned at distinct rates, allowing learning according to a prescribed order is optimal. Interestingly, the strategy with the lowest strategic advantage should be learnt first if we are to optimise fitness over the learning path. Then, the differences between strategies are balanced out in order to minimise the effect of behavioural uncertainty.
更多查看译文
关键词
incompetence, evolutionary games, learning, cooperation, snowdrift game
AI 理解论文
溯源树
样例
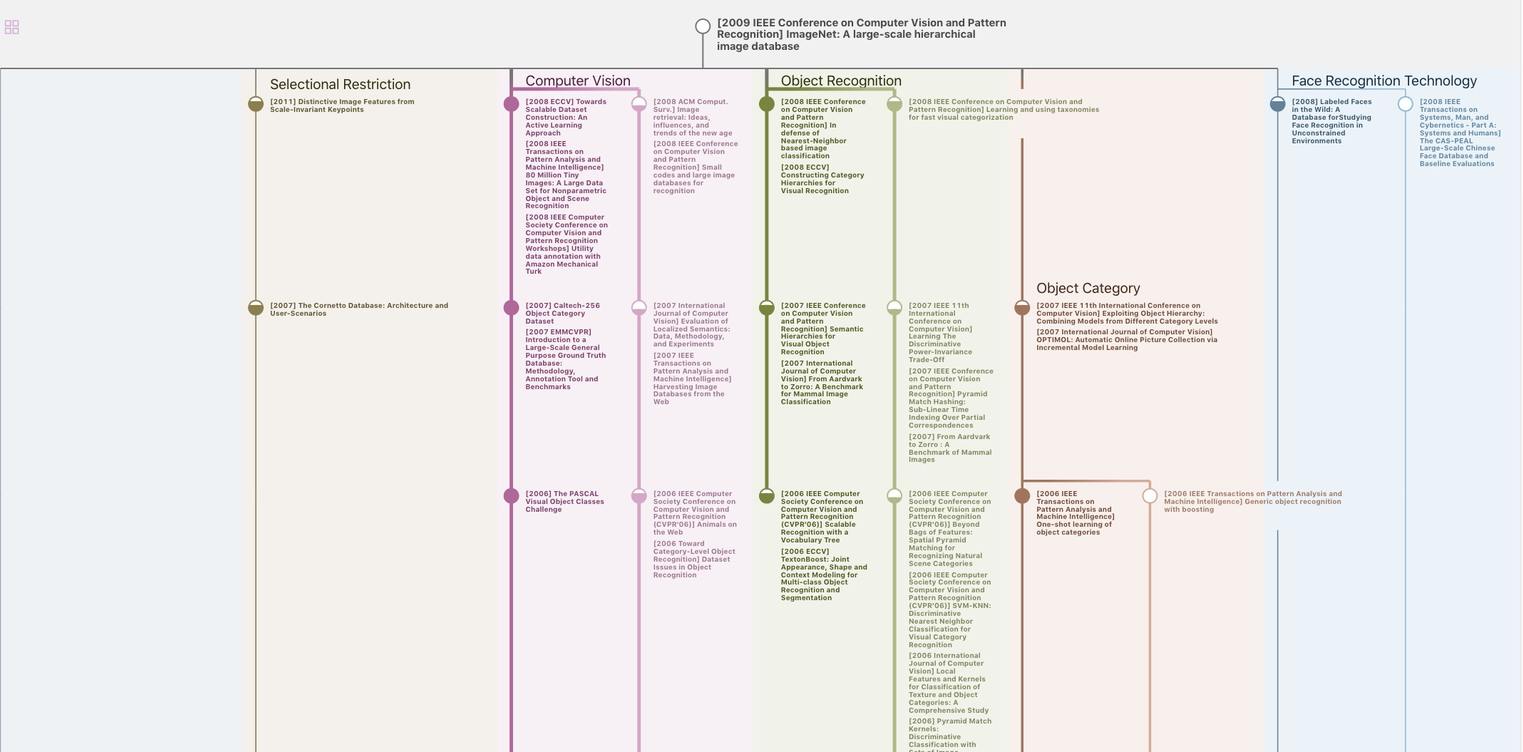
生成溯源树,研究论文发展脉络
Chat Paper
正在生成论文摘要