Dynamic Forgetting Krls For Online Time Series Prediction
PROCEEDINGS OF THE FIFTH INTERNATIONAL SYMPOSIUM ON TEST AUTOMATION & INSTRUMENTATION, VOLS 1 AND 2(2014)
摘要
To solve the low accuracy problem for online time series prediction in time varying environments, this paper proposes a dynamic forgetting kernel recursive least square (ICRLS) method. First, an exponential forgetting factor is introduced to the Approximate Linear Dependency KRLS (ALD-KRLS) which makes the newer samples have greater weight than the older ones. Thus this exponential forgetting ALD-ICRLS improves the ability to track in the time varying environments. Moreover, a dynamic forgetting factor method is developed by adjusting the forgetting degree according to the prediction error feedback. Therefore, this further enhances the prediction accuracy. Experiments results on classical Lorenz simulation data and NASA Li-ion battery capacity dataset demonstrate that the proposed algorithm improves the prediction accuracy without affecting the efficiency of original algorithm.
更多查看译文
关键词
time series prediction, online prediction, KRLS, forgetting factor
AI 理解论文
溯源树
样例
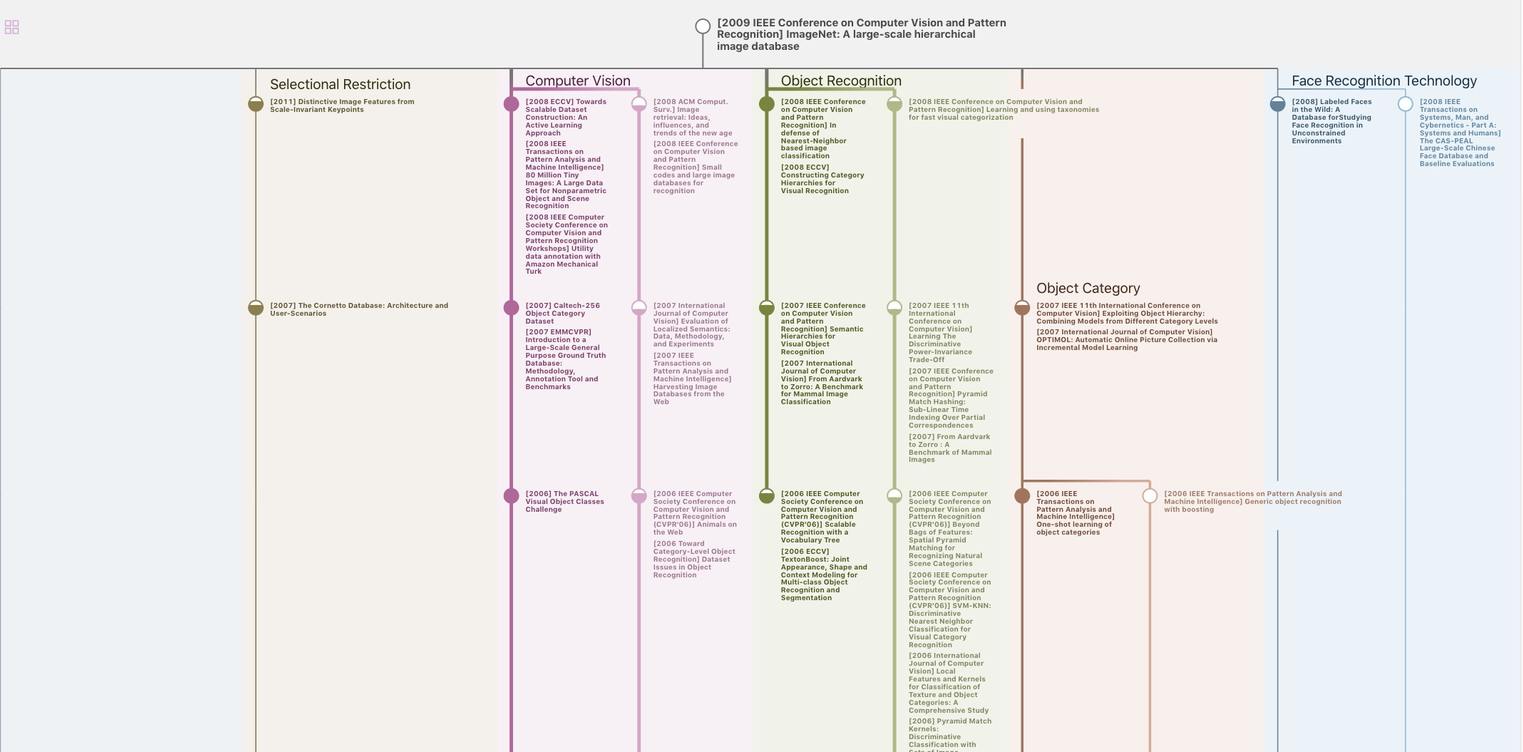
生成溯源树,研究论文发展脉络
Chat Paper
正在生成论文摘要