Building Cognitive Profiles of Learners Using EEG
2020 11th International Conference on Information and Communication Systems (ICICS)(2020)
摘要
Cognitive load refers to the used amount of working memory. It is very hard to be detected, especially in on-line and computer-supported learning. Thus, it is one of the most important challenges for educational technologists and instructional designers to address. Electroencephalogram (EEG) is a methodology that monitors the electric activity in the brain. EEG has been effective in detecting subjects’ emotional and cognitive states. In this paper, an approach for detecting the basic cognitive states that affect learning outcomes using EEG signals is proposed. Detected states include engagement, instantaneous attention, focused attention, working memory, planning, shifting and visual perception. The proposed approach consists of the following. First, 127 students in their undergraduate university-level studies undergo scientifically-validated cognitive assessment tests that evoke and measure their full cognitive profiles while putting on a 14-channel wearable EEG headset. Then, the collected data are used to train deep models as well as shallow classifiers to automatically predict the analyzed cognitive states. Although the main advantage of the deep learning models is avoiding the hand-crafted features needed for the shallow classifiers, the shallow classifiers outperformed the deep learning-based ones with a minimum accuracy of 92% as compared to the deep models with a maximum obtained accuracy of 78%.
更多查看译文
关键词
EEG,Cognition,Classification,Adaptive Learning
AI 理解论文
溯源树
样例
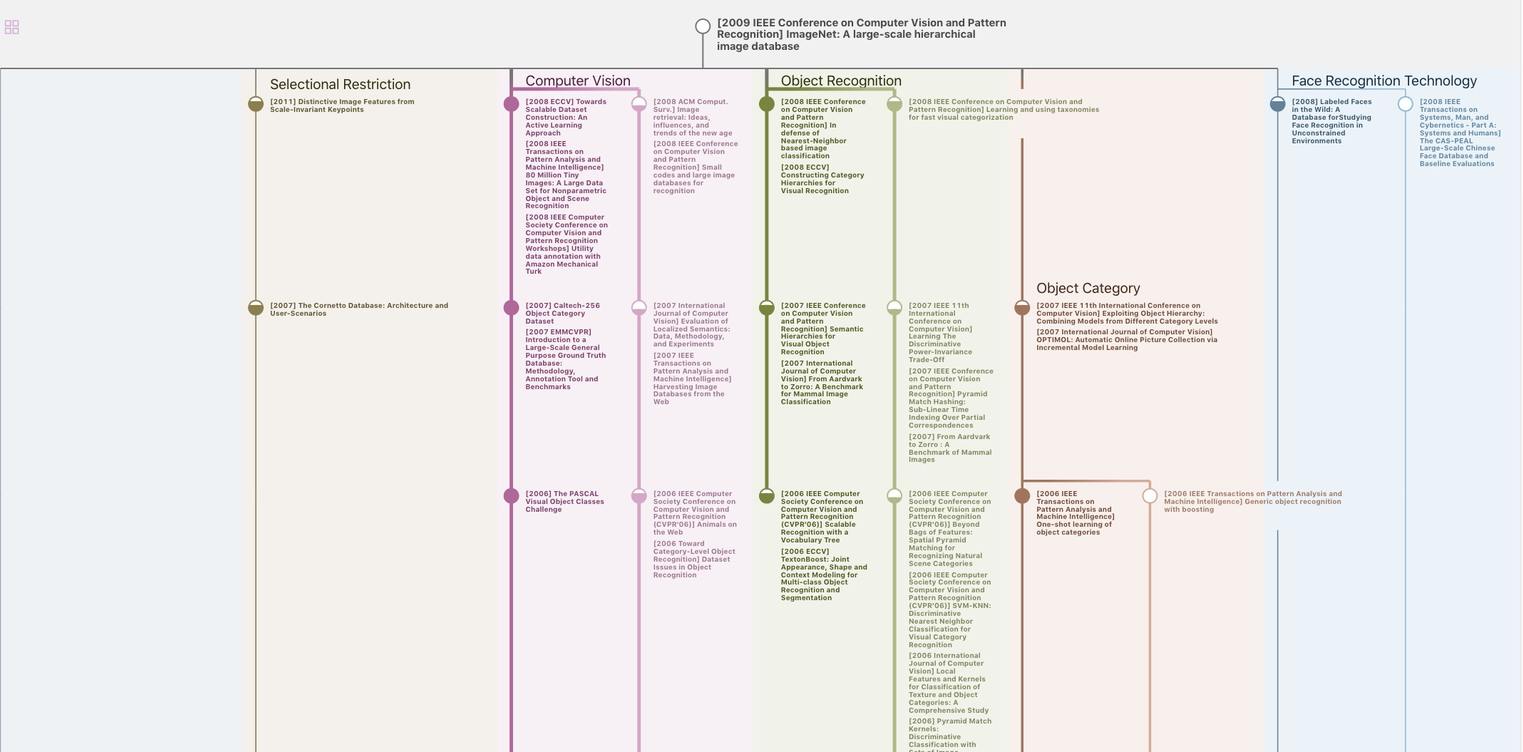
生成溯源树,研究论文发展脉络
Chat Paper
正在生成论文摘要