An Approach For Noise Induced Object Classification Accuracy Improvement
CYBER SENSING 2019(2019)
摘要
Among various parameters, large scene object detection and classification accuracy depends on image quality. In general, deep neural networks (DNN) are trained to achieve a desired recognition accuracy on a set of targets. However, DNNs become tuned to the training data used and may not generalize to new unseen data artifacts. Classification accuracy of a previously trained DNN is significantly reduced when classification is run on an image altered with additive noise. In this research, we propose image pre-processing to reduce the impact of noise induced low classification accuracy. Our approach consists of applying compressive sensing inspired pre-processing techniques to noisy images. We then compare the object recognition accuracy of a pretrained model on pre-processed noisy images and unprocessed noisy images. We will present our technical method, results, and analysis on relevant synthetic aperture radar data.
更多查看译文
关键词
synthetic-aperture-radar, target recognition, sparse approximation, noise removal, deep neural networks, convolutional neural networks, principle component analysis
AI 理解论文
溯源树
样例
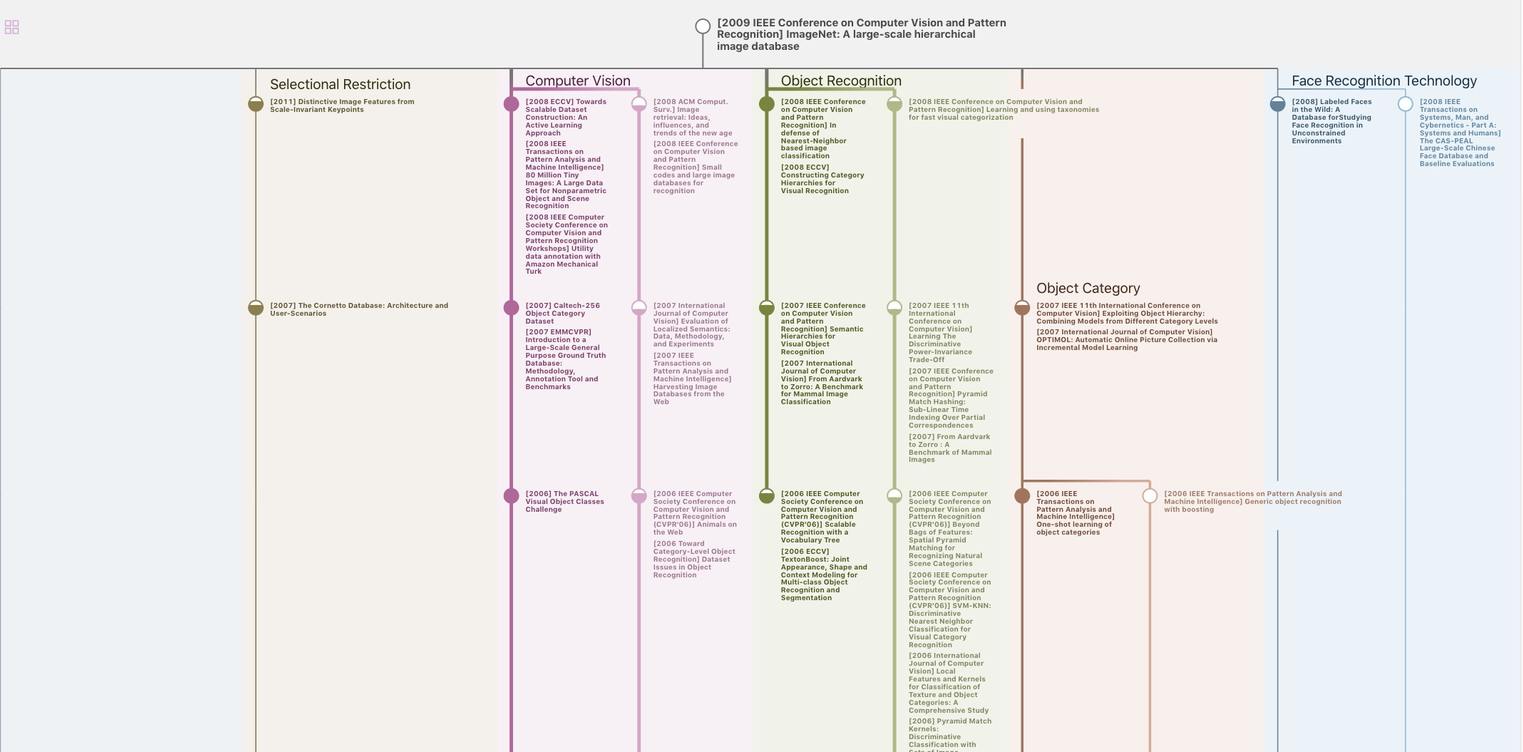
生成溯源树,研究论文发展脉络
Chat Paper
正在生成论文摘要