Predicting Solidification Cracking Susceptibility Of Stainless Steels Using Machine Learning
INTERNATIONAL CONFERENCE ON MODELLING OF CASTING, WELDING AND ADVANCED SOLIDIFICATION PROCESSES (MCWASP XV)(2020)
摘要
Machine learning, which reveals the complex nonlinear relationship in the archived data, is a powerful complement to theory, experiment, and modeling. In this study we attempted to predict solidification cracking susceptibility of stainless steels as a function of chemistry and processing parameters using machine learning with a data set that contains about 600 longitudinal varestraint test results. Four machine learning models, i.e. decision tree, random forest, shallow neural network and deep neural network, were used to mine the data set. Our results show: deep neural network outperformed other models in prediction accuracy; tree-based models have accepted accuracy and better interpretability than neural network; machine learning models transforms scattered experimental data points into a map in high-dimensional chemistry and processing parameters space. The combination of different machine learning models reveals that the solidification cracking susceptibility of stainless steels was mainly determined by the ratio of Ni content to Cr content, impurity element content and the strain level.
更多查看译文
AI 理解论文
溯源树
样例
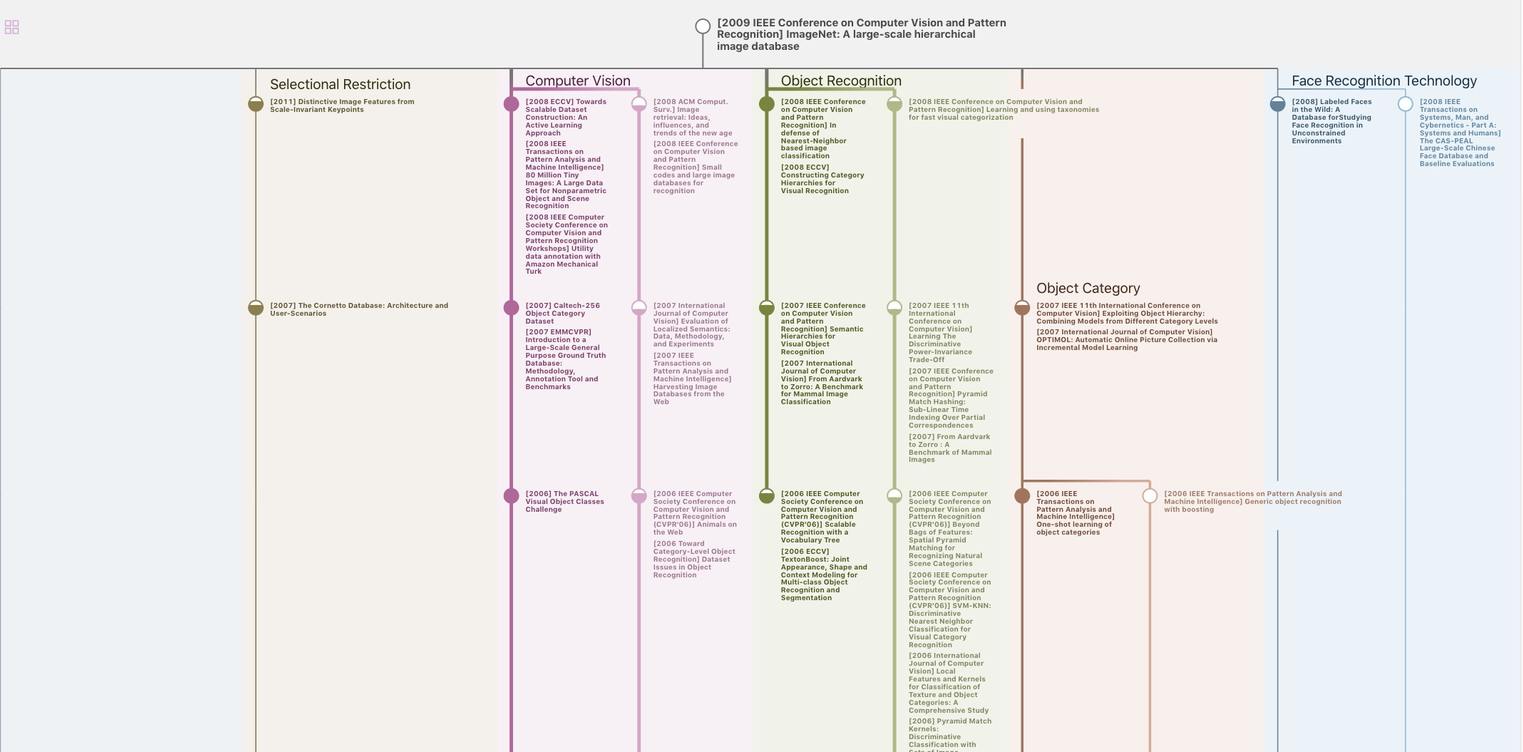
生成溯源树,研究论文发展脉络
Chat Paper
正在生成论文摘要