Cervical Histopathology Image Clustering Using Graph Based Unsupervised Learning
wos(2020)
摘要
In order to apply the important topological information to solve a Cervical Histopathology Image Clustering (CHIC) problem, a Graph Based Unsupervised Learning (GBUL) approach is proposed in this paper. First, the GBUL method applies color features and k-means clustering for a first-stage “coarse” clustering. Then, a Skeletonization Based Node Generation (SBNG) approach is introduced to approximate the distribution of cervical cell nuclei. Thirdly, based on the SBNG nodes, a minimum spanning tree graph is constructed. Next, graph features and additional geometrical features are extracted based on the constructed graph. Finally, the k-means clustering is applied again for the second-stage clustering. In the experiment, a practical cervical histopathology image dataset with ten whole scanned images is tested, obtaining a promising CHIC result and showing a huge potential in the cancer risk prediction field.
更多查看译文
关键词
Cervical cancer, Histopathology image, Clustering, Unsupervised learning, Skeletonization, Graph theory
AI 理解论文
溯源树
样例
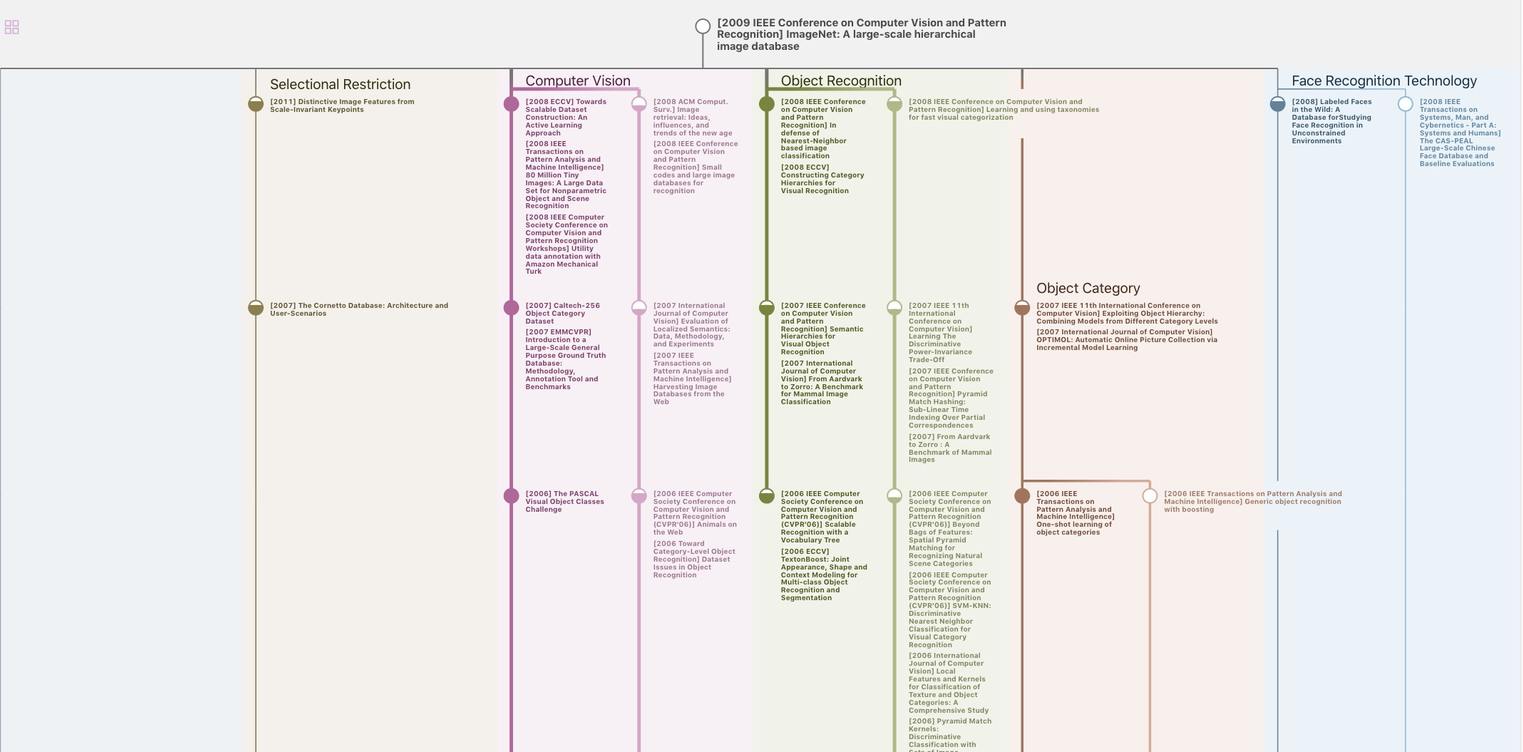
生成溯源树,研究论文发展脉络
Chat Paper
正在生成论文摘要