Generating Adversarial Examples For Static Pe Malware Detector Based On Deep Reinforcement Learning
5TH ANNUAL INTERNATIONAL CONFERENCE ON INFORMATION SYSTEM AND ARTIFICIAL INTELLIGENCE (ISAI2020)(2020)
摘要
Machine learning technology has been applied in filed of malware detection; it can improve efficiency of malware detection to deal with more and more increasing malware variants. However, malware detection model based on machine learning also has weakness which can be cheated by adversarial examples. Researching on method of generating adversarial examples could be benefit to exposing vulnerability of malware detection model and designing better malware detector. In this paper, we propose a reinforcement learning environment named gym-malware-mini based on gym-malware through which we generate adversarial examples using DQN and A2C deep reinforcement learning algorithm. As a result, DQN agent learned better policy of generating adversarial examples in gym-malware-mini, Success rate is increased by 18% compared with gym-malware. Success rate of DQN agent and A2C agent is increased by 20% and 15% compared with random agent in gym-malware-mini environment.
更多查看译文
AI 理解论文
溯源树
样例
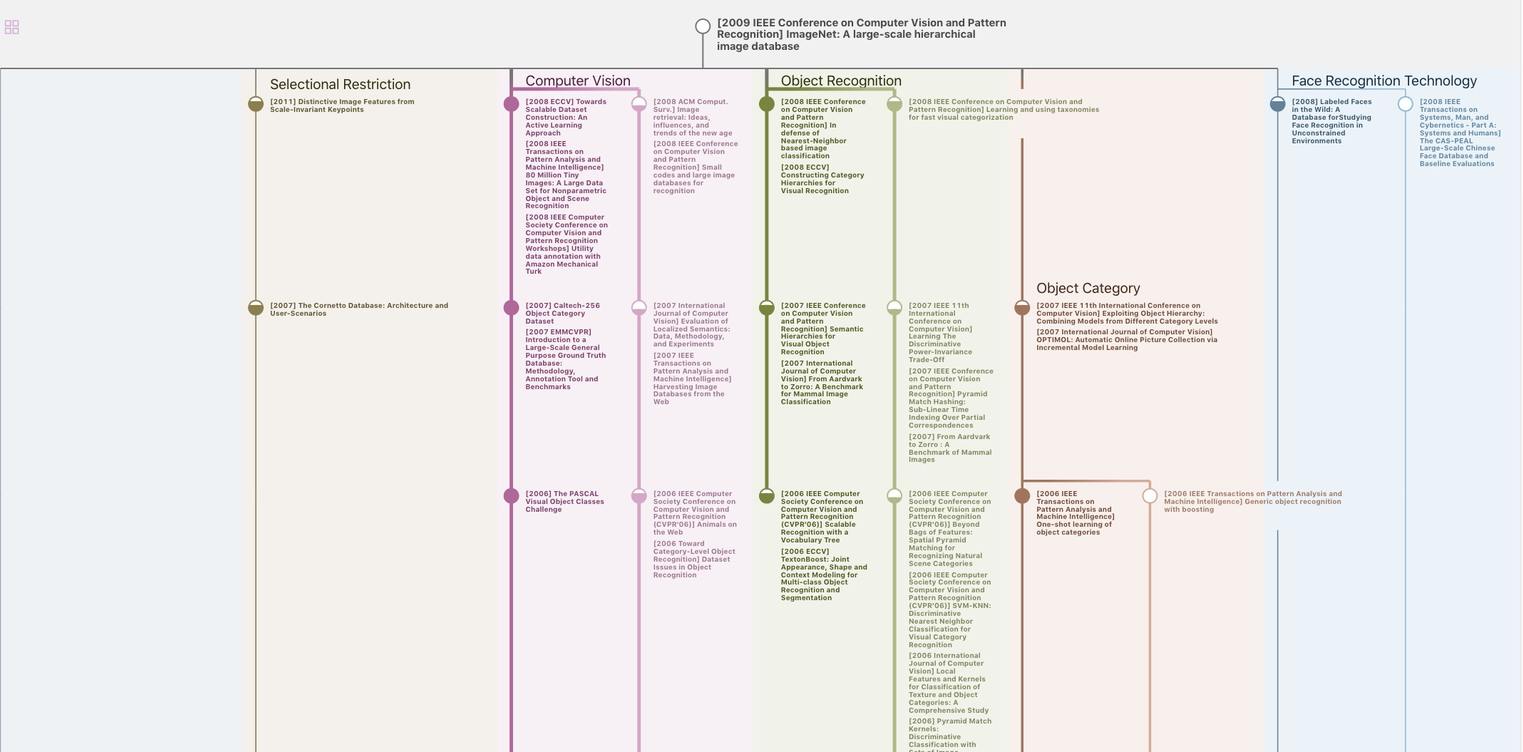
生成溯源树,研究论文发展脉络
Chat Paper
正在生成论文摘要