Predicting Regional Soybean Yield Using Crop Growth Simulation Model
KOREAN JOURNAL OF REMOTE SENSING(2017)
摘要
The present study was to develop an approach for predicting soybean yield using a crop growth simulation model at the regional level where the detailed and site-specific information on cultivation management practices is not easily accessible for model input. CROPGRO-Soybean model included in Decision Support System for Agrotechnology Transfer (DSSAT) was employed for this study, and Illinois which is a major soybean production region of USA was selected as a study region. As a first step to predict soybean yield of Illinois using CROPGRO-Soybean model, genetic coefficients representative for each soybean maturity group (MG I similar to VI) were estimated through sowing date experiments using domestic and foreign cultivars with diverse maturity in Seoul National University Farm (37.27 degrees N, 126.99 degrees E) for two years. The model using the representative genetic coefficients simulated the developmental stages of cultivars within each maturity group fairly well. Soybean yields for the grids of 10 kmx10 km in Illinois state were simulated from 2,000 to 2,011 with weather data under 18 simulation conditions including the combinations of three maturity groups, three seeding dates and two irrigation regimes. Planting dates and maturity groups were assigned differently to the three sub-regions divided longitudinally. The yearly state yields that were estimated by averaging all the grid yields simulated under non-irrigated and fully-Irrigated conditions showed a big difference from the statistical yields and did not explain the annual trend of yield increase due to the improved cultivation technologies. Using the grain yield data of 9 agricultural districts in Illinois observed and estimated from the simulated grid yield under 18 simulation conditions, a multiple regression model was constructed to estimate soybean yield at agricultural district level. In this model a year variable was also added to reflect the yearly yield trend. This model explained the yearly and district yield variation fairly well with a determination coefficients of R-2 = 0.61 (n = 108). Yearly state yields which were calculated by weighting the model-estimated yearly average agricultural district yield by the cultivation area of each agricultural district showed very close correspondence (R-2 = 0.80) to the yearly statistical state yields. Furthermore, the model predicted state yield fairly well in 2012 in which data were not used for the model construction and severe yield reduction was recorded due to drought.
更多查看译文
关键词
CROPGRO-Soybean model, Multiple regression, Regional soybean yield, yield prediction, 10-km grid
AI 理解论文
溯源树
样例
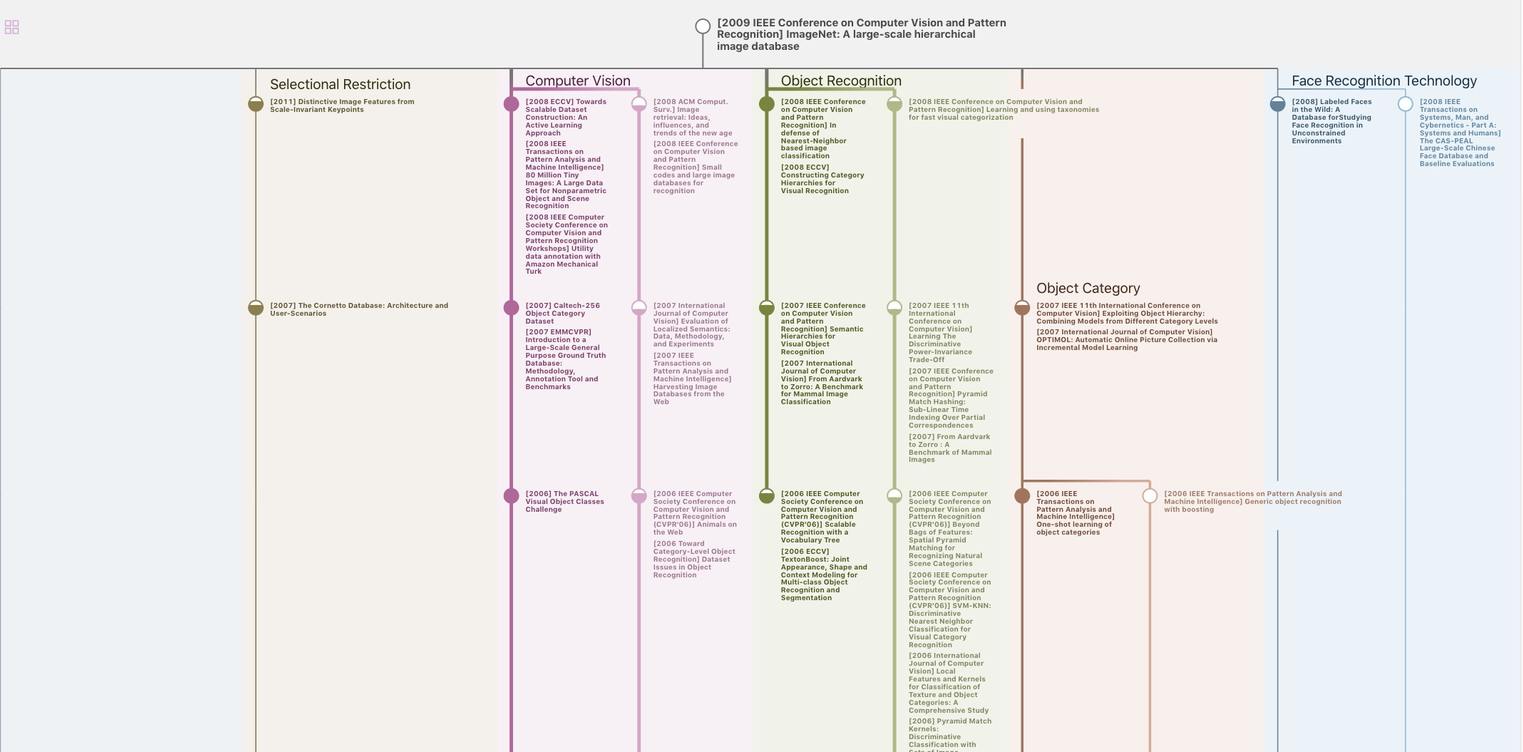
生成溯源树,研究论文发展脉络
Chat Paper
正在生成论文摘要