Generalized Sobol Indices Dediacted To The Dependent Variables: Performance Tests Of The Hogs Algorithm Coupled To Several Parametric Estimators
JOURNAL OF THE SFDS(2017)
摘要
The algorithm "Hierarchically Orthogonal Gram-Schmidt"(HOGS) (Chastaing et al., 2015) estimates generalized Sobol indices dedicated to models with dependent inputs, quantifying explicitly the model sensitivity due to correlations. HOGS constructs a meta-model for each variable of interest by projection on a functional basis suited to indices calculation. Regression coefficients are obtained with the ordinary least-square estimator (OLS) or penalized regression methods Lasso, Ridge and Elastic Net (EN). Four study cases are proposed: three toy models allowing to investigate HOGS functioning and numerical properties, and the LNAS (Log-Normal Allocation and Senescence) model dedicated to the complex dynamics of plant growth. Several HOGS configurations and meta-model accuracy are tested by means of a consistency index. An interpretation of Sobol indices is given for LNAS. It appears that HOGS-OLS is the most efficient method when simulation resources are not limited. Otherwise, considering the issue of parameter estimation with sparsity highlights that: i) EN is more robust but more costly than Lasso, ii) the basis constructed by HOGS is too large which creates artificial sparsity. A modification of HOGS has been proposed to reduce the dimension of the basis.
更多查看译文
关键词
sensitivity analysis, HDMR, HOGS, penalized regression
AI 理解论文
溯源树
样例
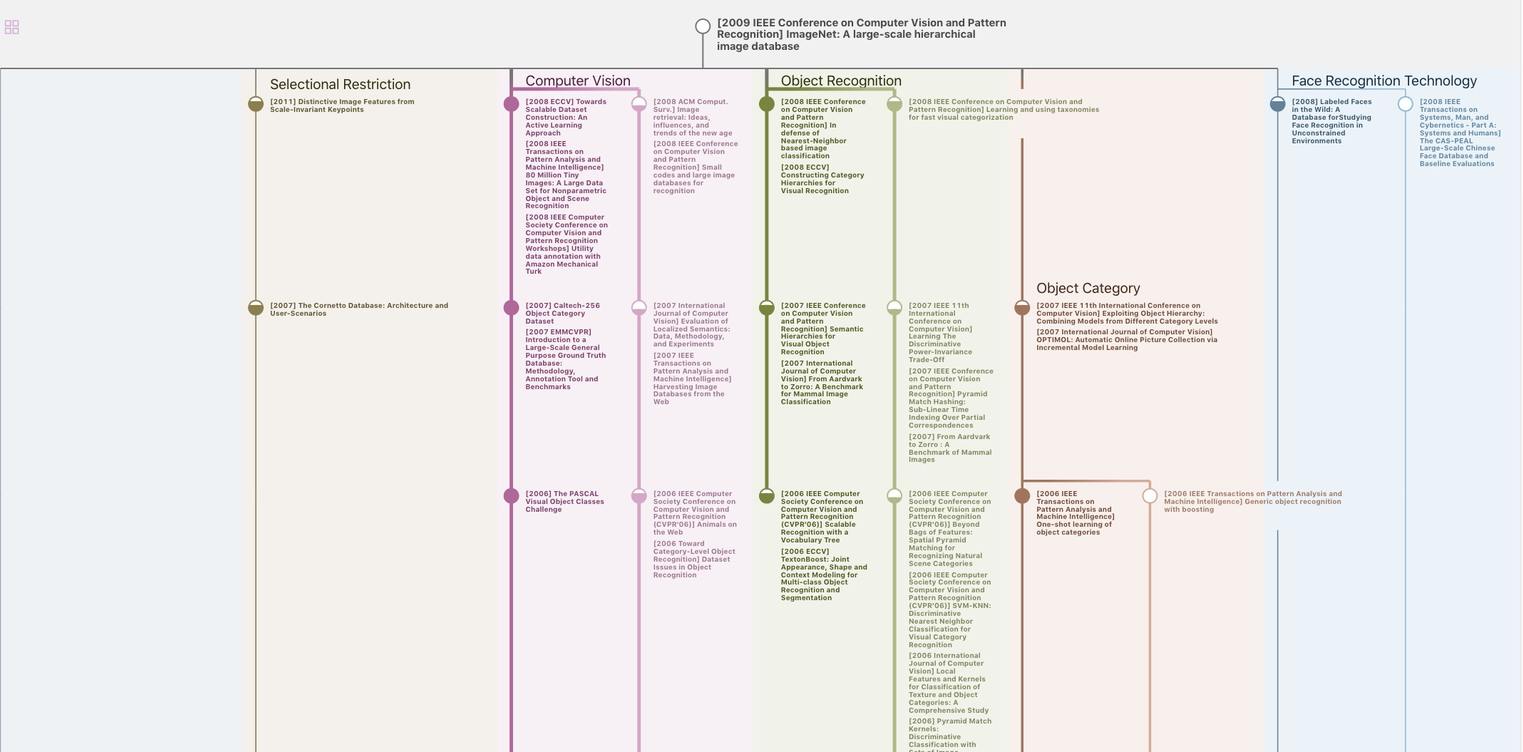
生成溯源树,研究论文发展脉络
Chat Paper
正在生成论文摘要