Flexible Image Analysis For Law Enforcement Agencies With Deep Neural Networks To Determine: Where, Who And What
COUNTERTERRORISM, CRIME FIGHTING, FORENSICS, AND SURVEILLANCE TECHNOLOGIES II(2018)
摘要
Due to the increasing need for effective security measures and the integration of cameras in commercial products, a huge amount of visual data is created today. Law enforcement agencies (LEAs) are inspecting images and videos to find radicalization, propaganda for terrorist organizations and illegal products on darknet markets. This is time consuming. Instead of an undirected search, LEAs would like to adapt to new crimes and threats, and focus only on data from specific locations, persons or objects, which requires flexible interpretation of image content. Visual concept detection with deep convolutional neural networks (CNNs) is a crucial component to understand the image content. This paper has five contributions. The first contribution allows image-based geo-localization to estimate the origin of an image. CNNs and geotagged images are used to create a model that determines the location of an image by its pixel values. The second contribution enables analysis of fine-grained concepts to distinguish sub-categories in a generic concept. The proposed method encompasses data acquisition and cleaning and concept hierarchies. The third contribution is the recognition of person attributes (e.g., glasses or moustache) to enable query by textual description for a person. The person-attribute problem is treated as a specific sub-task of concept classification. The fourth contribution is an intuitive image annotation tool based on active learning. Active learning allows users to define novel concepts flexibly and train CNNs with minimal annotation effort. The fifth contribution increases the flexibility for LEAs in the query definition by using query expansion. Query expansion maps user queries to known and detectable concepts. Therefore, no prior knowledge of the detectable concepts is required for the users. The methods are validated on data with varying locations (popular and non-touristic locations), varying person attributes (CelebA dataset), and varying number of annotations.
更多查看译文
关键词
Deep learning, concept detection, convolutional neural networks, image-based geo-localization, fine-grained concept detection, person attributes, active learning, query expansion
AI 理解论文
溯源树
样例
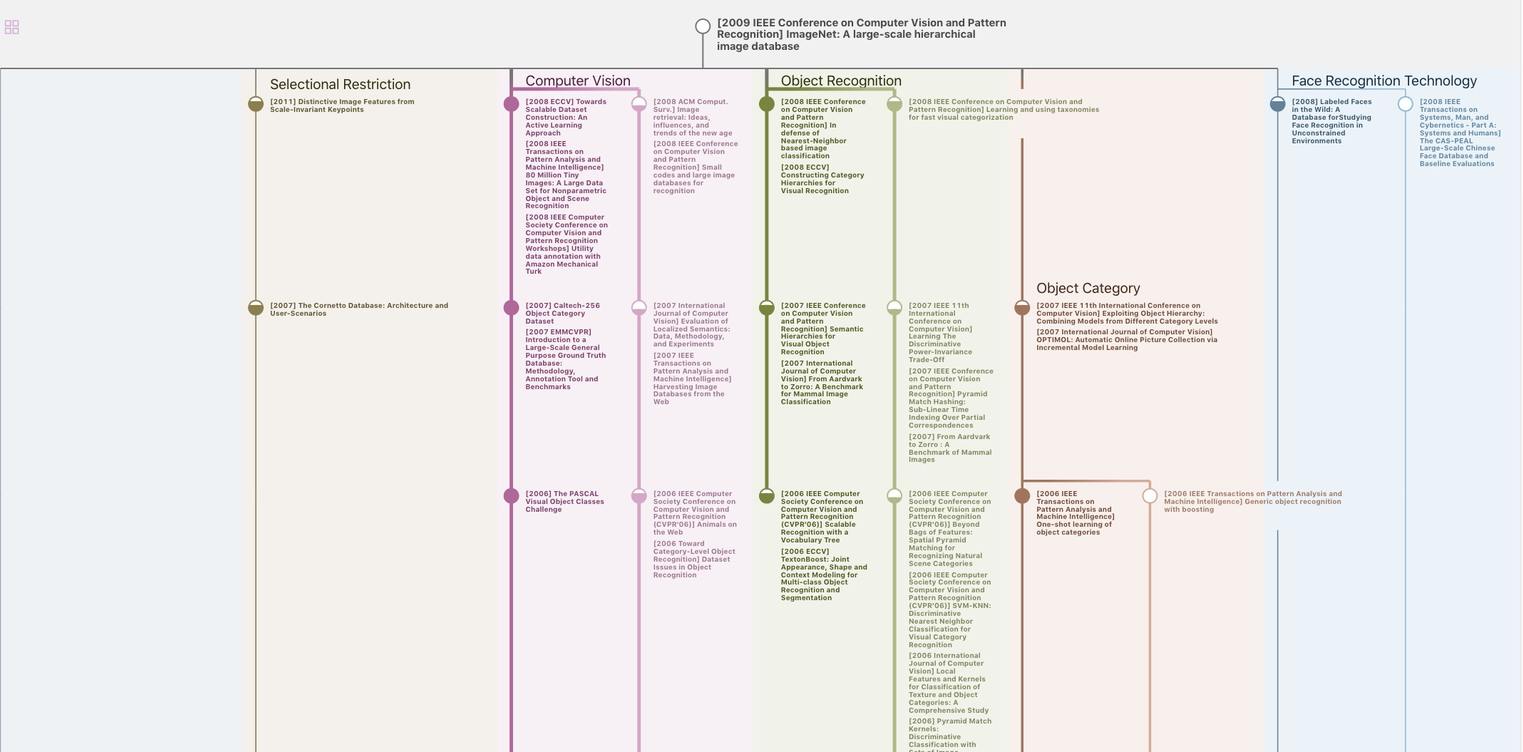
生成溯源树,研究论文发展脉络
Chat Paper
正在生成论文摘要