Convolutional-Neural-Network Based Breast Thickness Correction In Digital Breast Tomosynthesis
15TH INTERNATIONAL WORKSHOP ON BREAST IMAGING (IWBI2020)(2020)
摘要
This work addresses equalization and thickness estimation of breast periphery in digital breast tomosynthesis (DBT). Breast compression in DBT would lead to a relatively uniform thickness at inner breast but not at the periphery. Proper peripheral enhancement or thickness correction is needed for diagnostic convenience and for accurate volumetric breast density estimation. Such correction methods have been developed albeit with several shortcomings. We present a thickness correction method based on a supervised learning scheme with a convolutional neural network (CNN), which is one of the widely-used deep learning structures, to improve the pixel value of the peripheral region. The network was successfully trained and showed a robust and satisfactory performance in our numerical phantom study.
更多查看译文
关键词
digital breast tomosynthesis, CNN, peripheral equalization, thickness correction
AI 理解论文
溯源树
样例
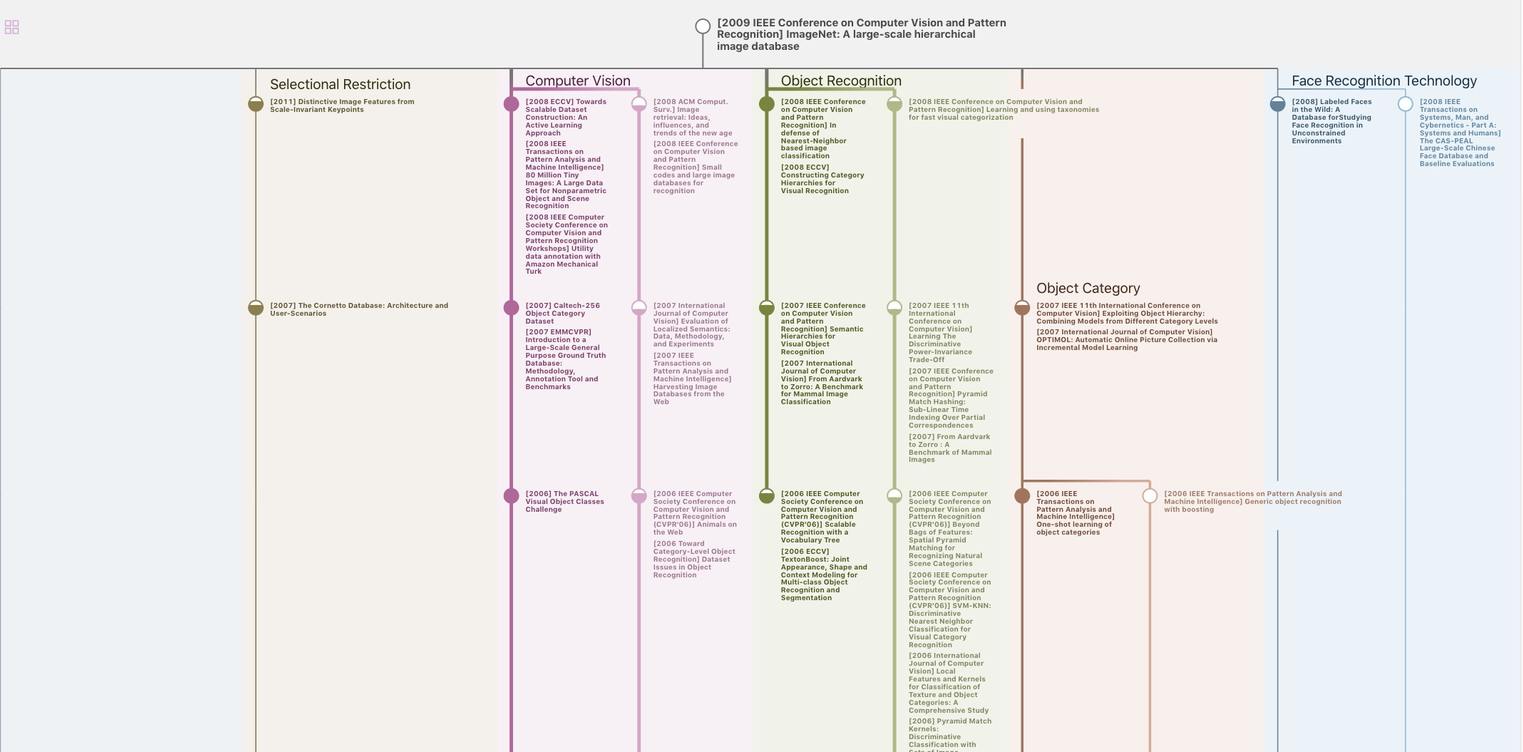
生成溯源树,研究论文发展脉络
Chat Paper
正在生成论文摘要