Ddeep3m-Based Neuronal Cell Counting In 2d Large-Scale Images
OPTICS IN HEALTH CARE AND BIOMEDICAL OPTICS IX(2020)
摘要
The number of neuronal cells is fundamentally important for brain functions. However, it can be difficult to obtain the accurate number of neuronal cells in large-scale brain imaging, which is nearly inevitable with traditional image segmentation techniques due to the low contrast and noisy background. Here, we introduce a Docker-based deep convolutional neural network (DDeep3M) for better counting neurons in the stimulated Raman scattering (SRS) microscopy images. To reconcile the memory limit of computational resource, a high-resolution 2D SRS image of whole coronal slice of mouse brain is divided into multiple patch images. Each patch image is then fed into the DDeep3M and predicted as a probability map. A higher contrast image targeting neurons (i.e. the predicted image) can be acquired by stitching the patches of probability map together. With this routine segmentation method applied in both raw SRS image and the predicted image, the DDeep3M achieves the accuracy of over 0.96 for cell counting which is much better than the result of traditional segmentation methods. Compared with the U-Net, which is one of the most popular deep learning networks for medical image segmentation, DDeep3M demonstrates a better result when handling such large-scale image. Thus, DDeep3M can be really helpful for large-scale cell counting in brain research.
更多查看译文
关键词
DDeep3M, segmentation, deep learning, cell counting, brain, ImageJ, large scale
AI 理解论文
溯源树
样例
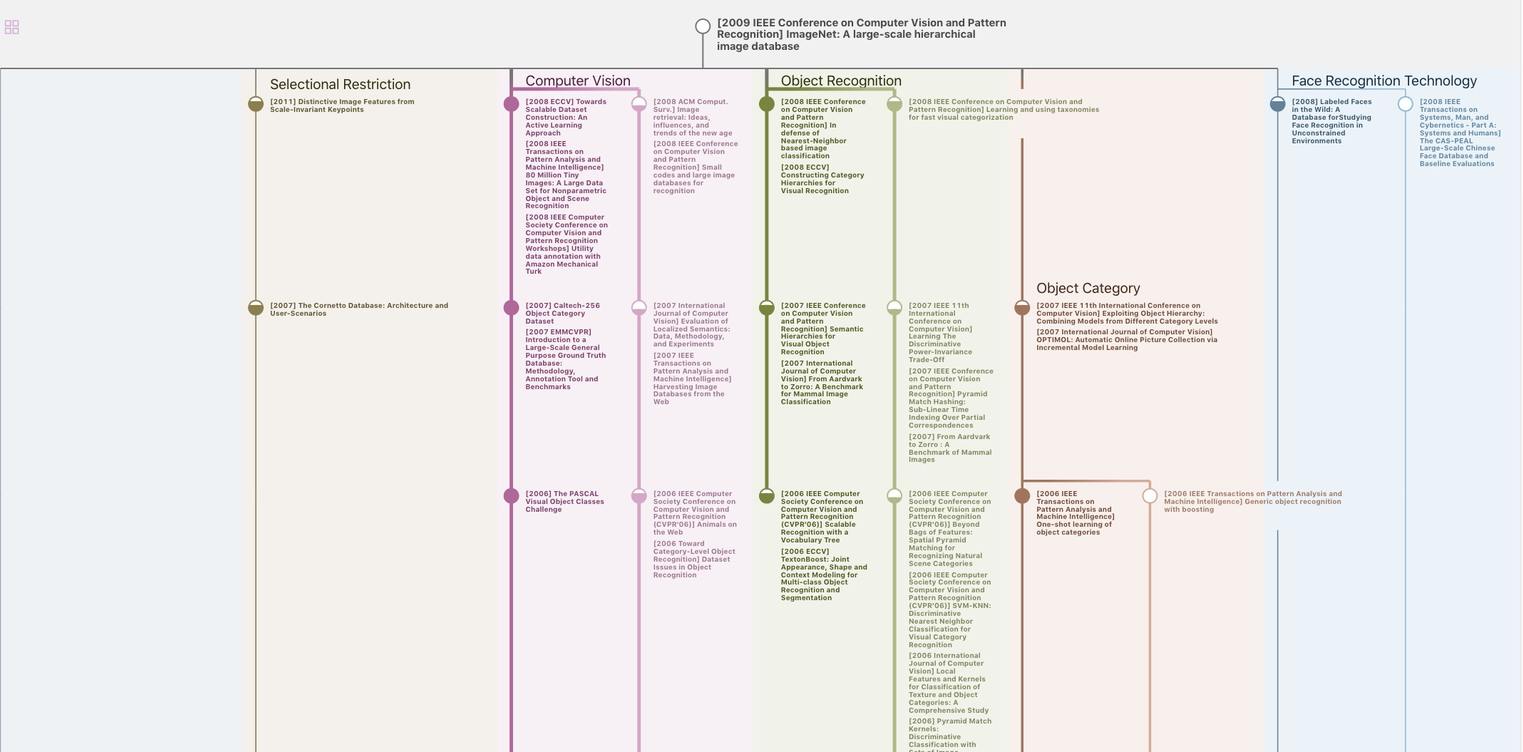
生成溯源树,研究论文发展脉络
Chat Paper
正在生成论文摘要