OPLD: Towards improved non-intrusive office plug load disaggregation
2015 IEEE International Conference on Building Efficiency and Sustainable Technologies(2015)
摘要
Practical energy auditing in offices poses several challenges unlike homes e.g. physically large space, diverse energy appliances types, several appliance instances and occupancy non-obstructiveness. However, improved energy-auditing measures using predictive analytics can benefit energy savings and reduce building operational costs. A review of some publicly available energy datasets such as AMPds, BLUED, ECO, REDD etc. is presented to help understand practical limitations in relying and applying them alike for office plug load audits. A possible approach to predict miscellaneous electrical plug loads (MELs) is proposed using Office Plug Load Dataset (OPLD) based on empirical characteristics and measurements of MELs devices. This work in progress study is one of the first attempts to characterize office desktop appliances across multiple states through a very large experimental dataset. The dataset might be effective in identifying individual appliances & its states in aggregate signature. This can find promising application in improving our understanding on office MELs and thus disaggregating them from single-point measurement.
更多查看译文
关键词
offfices,MELs,characterization,OPLD,energy audits
AI 理解论文
溯源树
样例
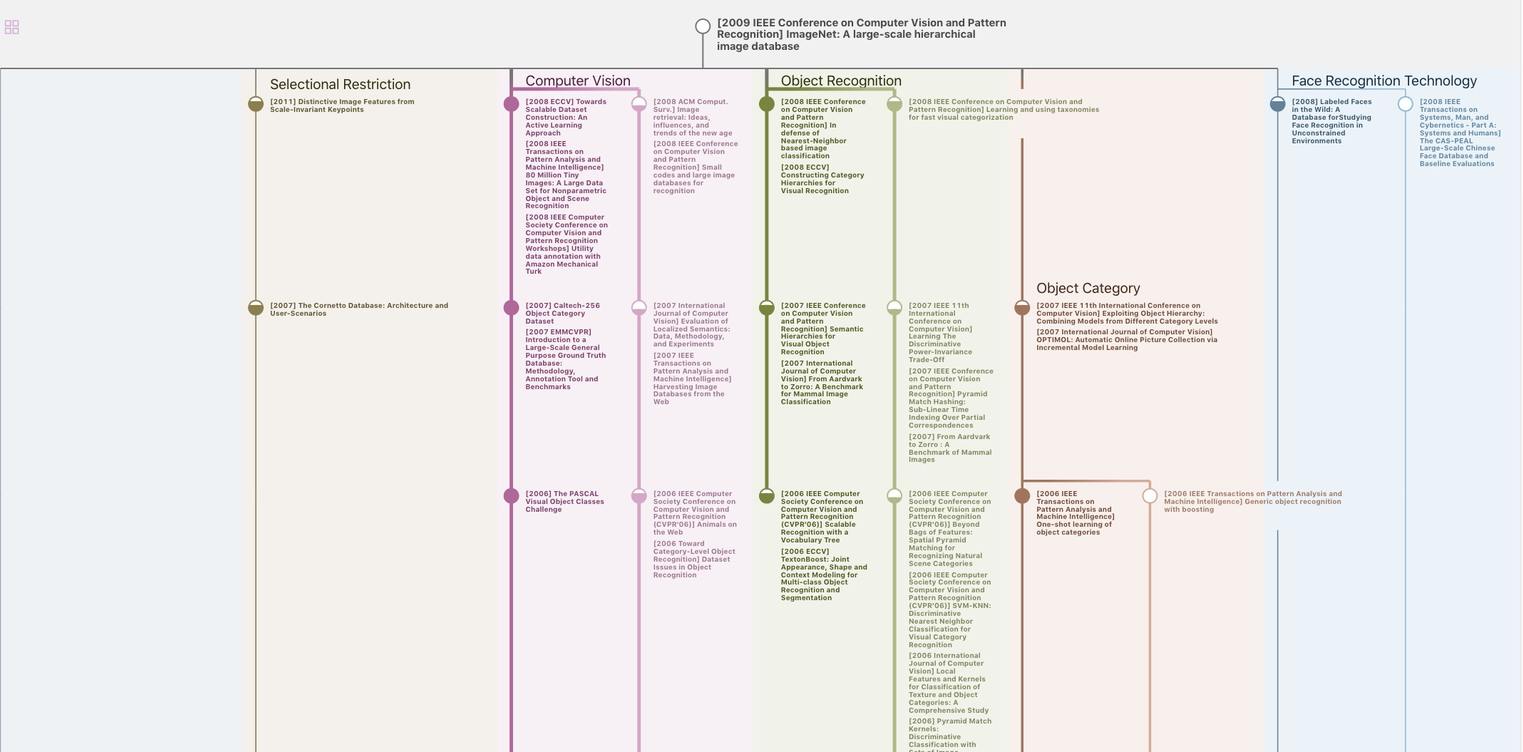
生成溯源树,研究论文发展脉络
Chat Paper
正在生成论文摘要