Efficient Super Greedy Boosting for Classification
2020 10th Institute of Electrical and Electronics Engineers International Conference on Cyber Technology in Automation, Control, and Intelligent Systems (CYBER)(2020)
摘要
Boosting is a well-known ensemble meta-algorithm, which has been widely used in machine learning. The main idea of boosting is to assemble several weak learners into a stronger one, which significantly improve their performance. However, numerous iterations are usually required for the boosting-type methods to achieve good performance, and thus inevitably bring heavy computational burden. In this paper, we propose a novel boosting method called Super Greedy Boosting (SGBoosting) for binary classification, to reduce the computational cost of the existing boosting-type methods. Instead of one weak learner selected at per iteration of the existing boosting-type methods, the proposed method selects a mini batch of weak learners at per iteration according to the so-called super greedy scheme, and thus needs much fewer iterations to yield sufficient number of weak learners to achieve high accuracy. A series of toy simulations and real data experiments are conducted to demonstrate the efficiency and effectiveness of the proposed Super Greedy Boosting method. The numerical results show that the proposed method can significantly reduce the computational cost with the comparable generalization performance of the state-of-the-art boosting-type methods.
更多查看译文
关键词
Classification,Boosting,Greedy,ADMM
AI 理解论文
溯源树
样例
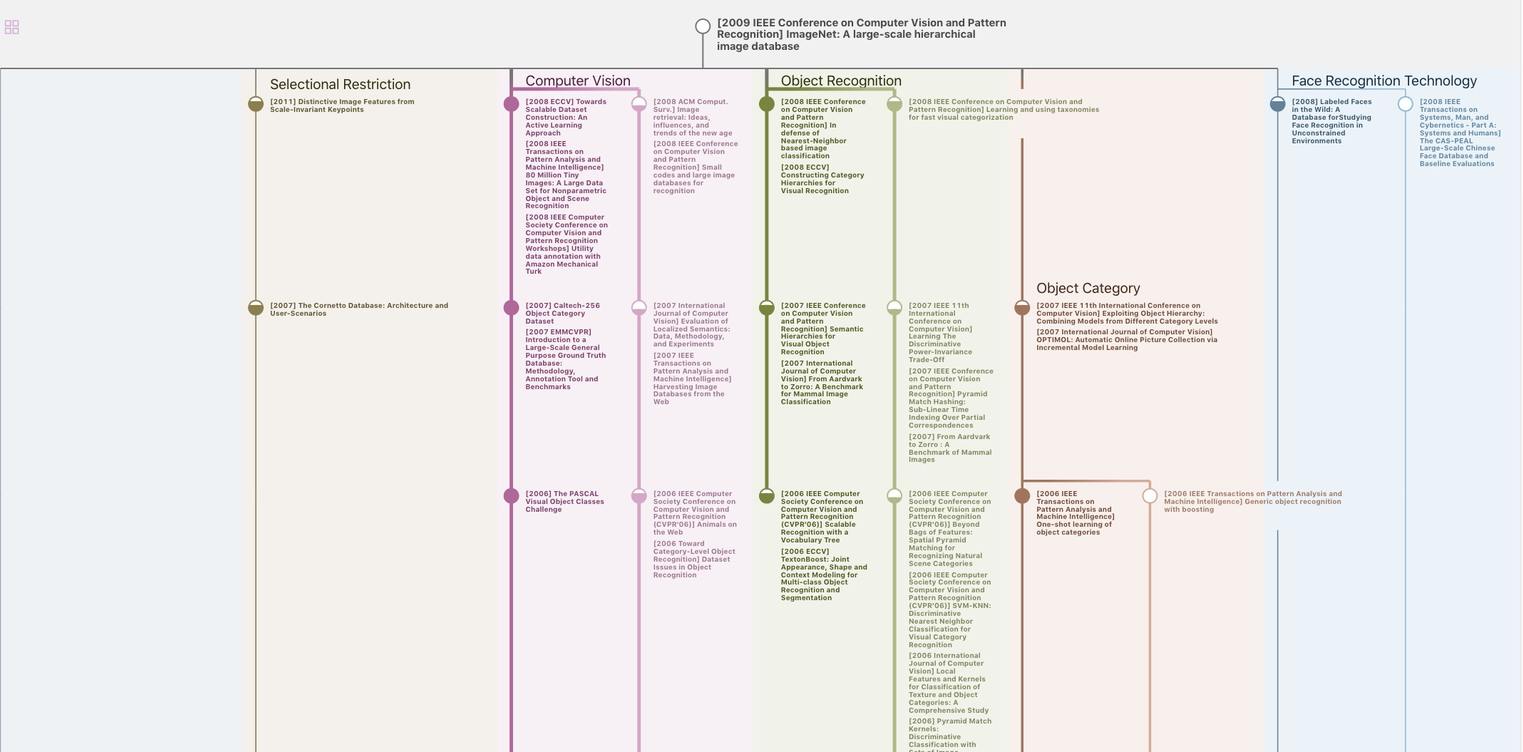
生成溯源树,研究论文发展脉络
Chat Paper
正在生成论文摘要