Deep Reinforcement Learning for Autonomous Traffic Light Control
2018 3rd IEEE International Conference on Intelligent Transportation Engineering (ICITE)(2018)
摘要
In urban areas, the efficiency of traffic flows largely depends on signal operation and expansion of the existing signal infrastructure is not feasible due to spatial, economic and environmental constraints. In this paper, we address the problem of congestion around the road intersections. We developed our traffic simulator to optimally simulate various traffic scenarios, closely related to real-world traffic situations. We contend that adaptive real-time traffic optimization is the key to improving existing infrastructure's effectiveness by enabling the traffic control system to learn, adapt and evolve according to the environment it is exposed to. We put forward a vision-based, deep reinforcement learning approach based on a policy gradient algorithm to configure traffic light control policies. The algorithm is fed real-time traffic information and aims to optimize the flows of vehicles travelling through road intersections. Our preliminary test results demonstrate that, as compared to the traffic light control methodologies based on previously proposed models, configuration of traffic light policies through this novel method is extremely beneficial.
更多查看译文
关键词
component,Autonomous Traffic Control,Machine Learning,Deep Reinforcement Learning,3d Virtual Reality Simulator
AI 理解论文
溯源树
样例
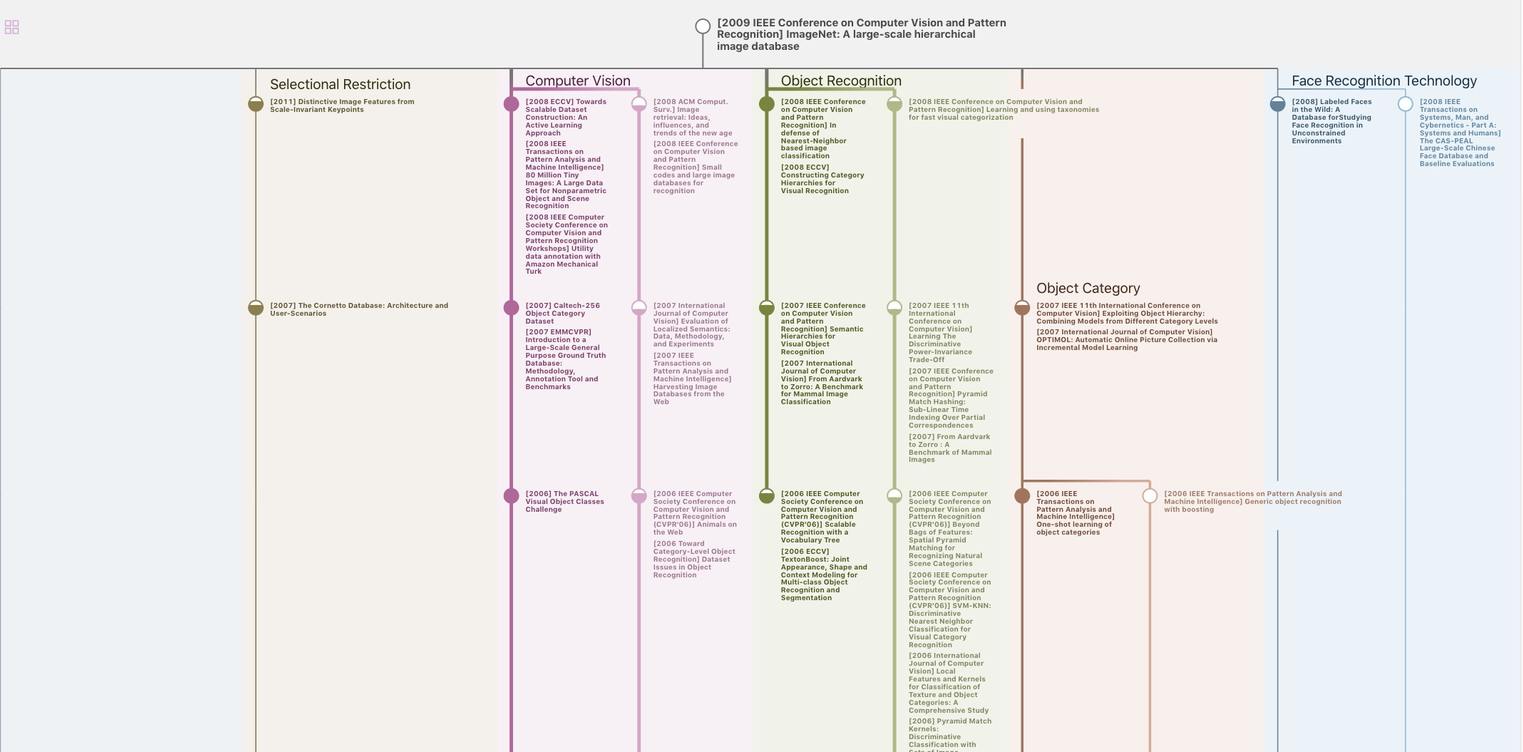
生成溯源树,研究论文发展脉络
Chat Paper
正在生成论文摘要