Automatic Knowledge Learning Using Case-Based Reasoning
2016 INTERNATIONAL JOINT CONFERENCE ON NEURAL NETWORKS (IJCNN)(2016)
摘要
This paper demonstrates the use of a classical Case-Based Reasoning (CBR) approach applied to the automatic train conduction scenario. We use a CBR model, where the adaptation task consists on a multi-objective optimization approach. To realize the case study we have used a train simulator. It is capable of conducting a train in a pre-defined railway providing relevant data about the conduction, such as, travel time and fuel consumption. In the experiments, we compare the data from the case study with a real scenario. The results are very promising considering fuel consumption and travel time; in some scenarios the economy of fuel was about 51%, and, we obtained a reduction about 69.5% of travel time in the best-case scenario. The CBR architecture proved to be efficient to the case study, because the past experiences assured a more efficient and economic train conduction.
更多查看译文
关键词
case-based reasoning,train conduction,multio-bjective optimization
AI 理解论文
溯源树
样例
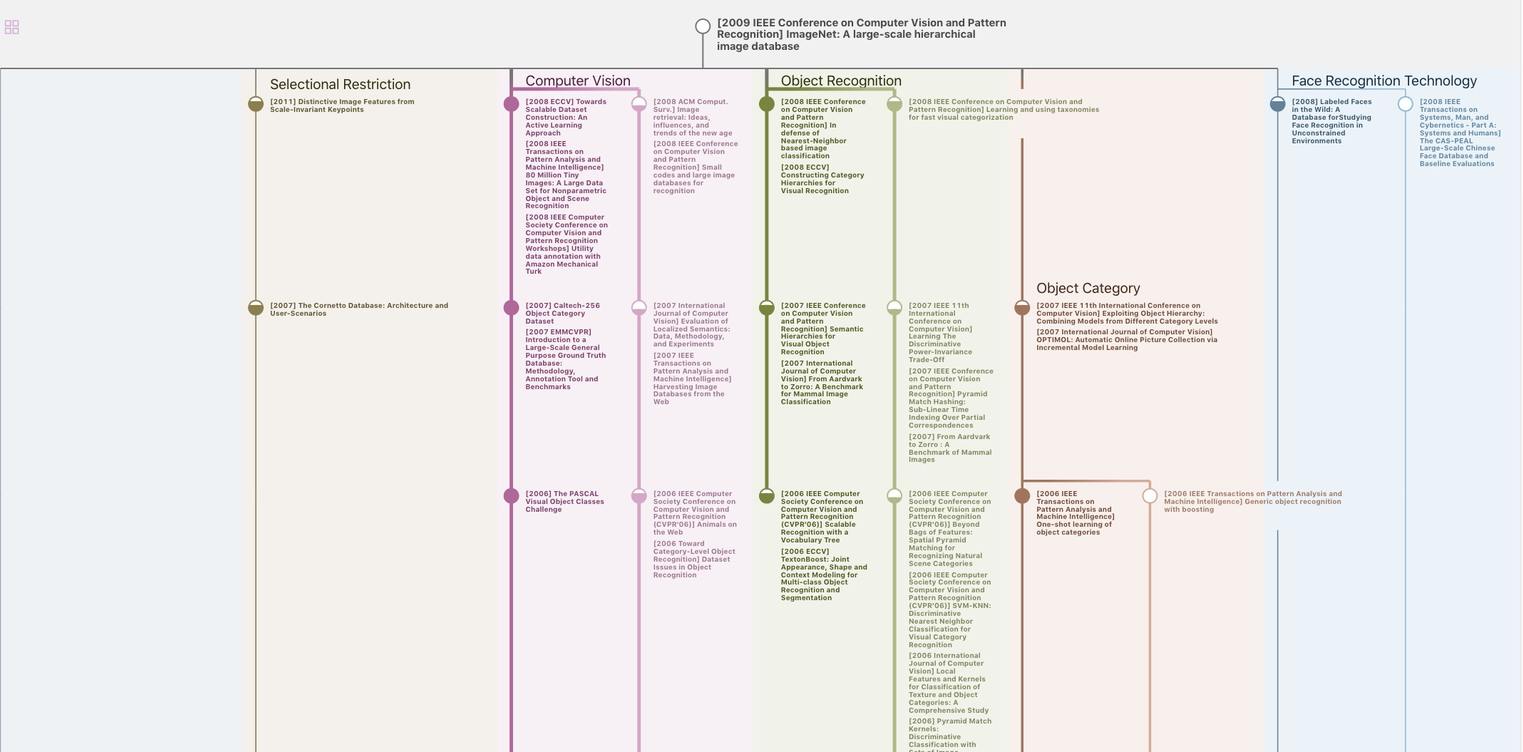
生成溯源树,研究论文发展脉络
Chat Paper
正在生成论文摘要