Multi-font Devanagari Text Recognition Using LSTM Neural Networks
wos(2020)
摘要
Current research in OCR is focusing on the effect of multi-font and multi-size text on OCR accuracy. To the best of our knowledge, no study has been carried out to study the effect of multi-fonts and multi-size text on the accuracy of Devanagari OCRs. The most popular Devanagari OCRs in the market today are Tesseract OCR, Indsenz OCR and eAksharayan OCR. In this research work, we have studied the effect of font styles, namely Nakula, Baloo, Dekko, Biryani and Aparajita on these three OCRs. It has been observed that the accuracy of the Devanagari OCRs is dependent on the type of font style in text document images. Hence, we have proposed a multi-font Devanagari OCR (MFD_OCR), text line recognition model using long short-term memory (LSTM) neural networks. We have created training dataset Multi_Font_Train, which consists of text document images and its corresponding text file. This consists of each text line in five different font styles, namely Nakula, Baloo, Dekko, Biryani and Aparajita. The test dataset is created using the text from benchmark dataset [1] for each of the font styles as mentioned above, and they are named as BMT_Nakula, BMT_Baloo, BMT_Dekko, BMT_Biryani and BMT_Aparajita test dataset. On the evaluation of all OCRs, the MFD_OCR showed consistent accuracy across all these test datasets. It obtained comparatively good accuracy for BMT_Dekko and BMT_Biryani test datasets. On performing detailed error analysis, we noticed that compared to other Devanagari OCRs, the MFD_OCR has consistent, insertion and deletion type of errors, across all test dataset for each font style. The deletion errors are negligible, ranging from 0.8 to 1.4%.
更多查看译文
关键词
Multi-font, OCR, Devanagari
AI 理解论文
溯源树
样例
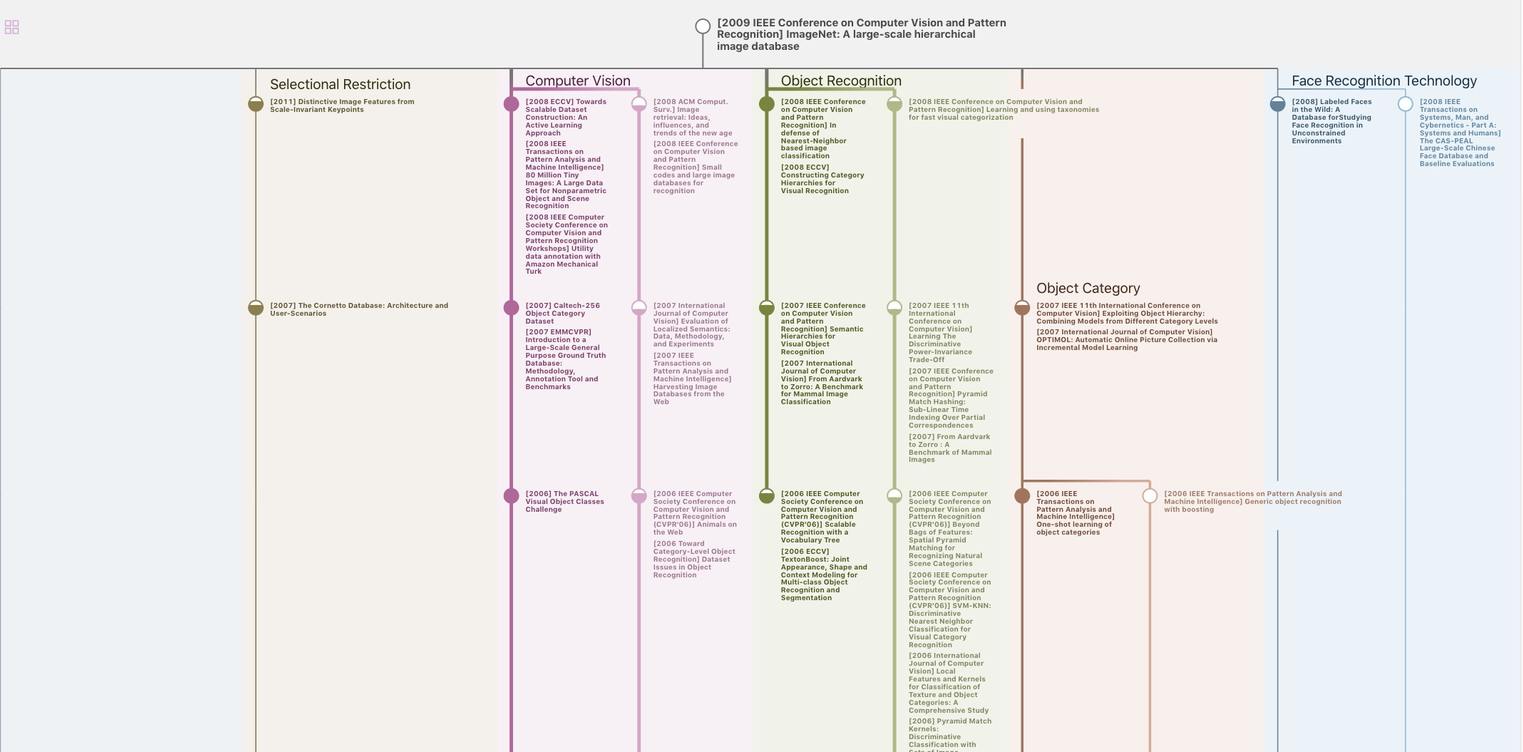
生成溯源树,研究论文发展脉络
Chat Paper
正在生成论文摘要