Minimizing Prediction Time for Catheter-Associated Urinary Tract Infection Using Big Data Mining Model
2019 2nd International Conference on Computer Applications & Information Security (ICCAIS)(2019)
摘要
This paper focuses on minimizing prediction time for Catheter-Associated Urinary Tract Infection (CAUTI) as one of the main types of Healthcare Associated Infections (HAIs) through a big data analytics model. Big data raises the bar as a result of additional features. It is mainly characterized by tremendous amount of data that is composed of different forms. It also deals with the rapid data flow rate that is generated from multiple sources, and to top it off the quality of the data is questionable. Data mining (DM) approach consumes significantly less time, provides higher accuracy, and prevents personal subjective decisions. The paper evaluates seven data mining algorithms with real patients dataset. It includes more than 28,000 cases for a period of five years. The modeling process considers the latest definition of the Centers for Disease Control and Prevention (CDC), published in January 2019. The model is evaluated through calculations of different assessment factors such as accuracy, computation speed, and precision (true positive and true negative). The research results show the best suitable algorithm is Naïve Bayes. It overcomes the other data mining techniques utilized in similar works.
更多查看译文
关键词
Big Data Analytics,Data Mining,Healthcare Associated Infections,Catheter-Associated Urinary Tract Infection
AI 理解论文
溯源树
样例
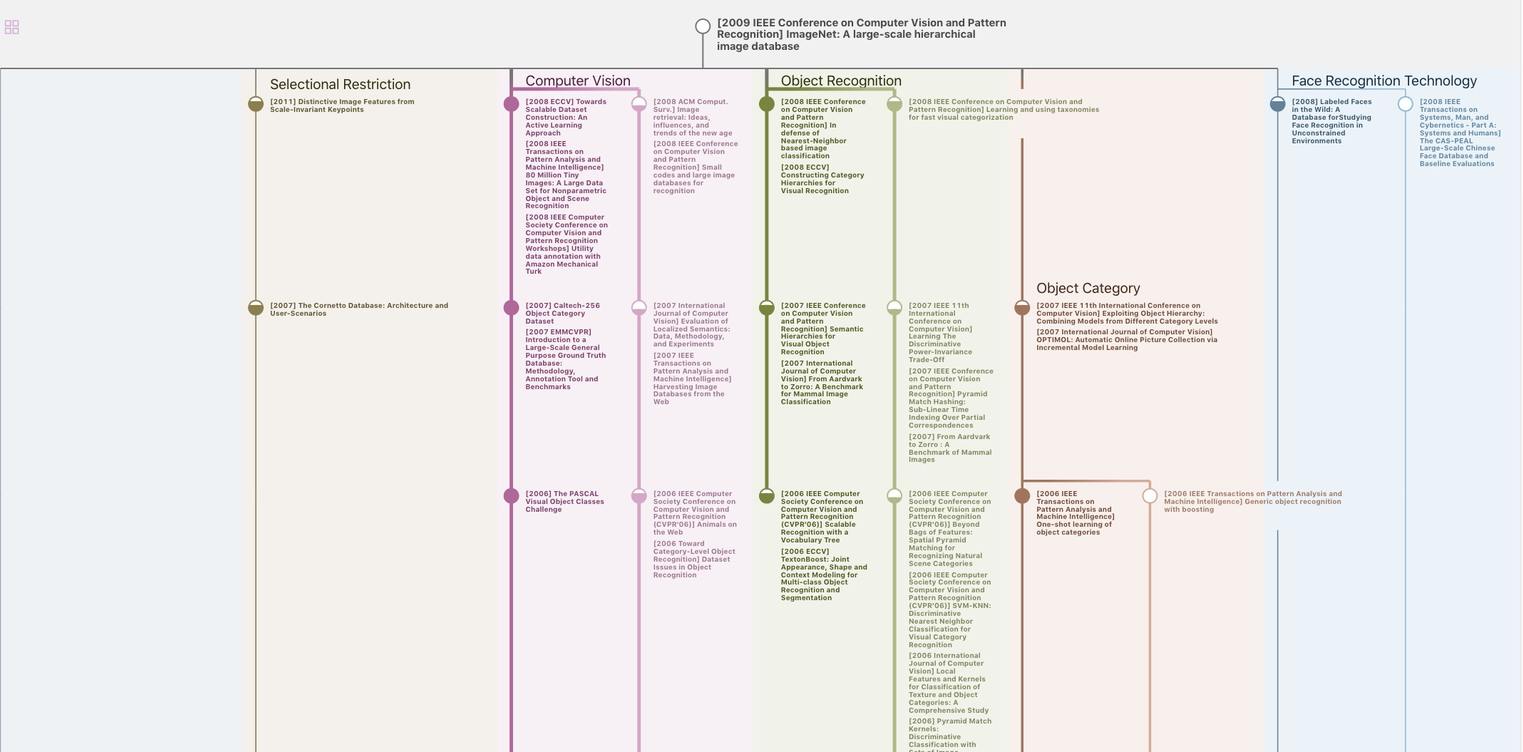
生成溯源树,研究论文发展脉络
Chat Paper
正在生成论文摘要