Liver and Brain Levels of 11 beta-hydroxysteroid Dehydrogenase Type 1 Enzyme in Obesity: Preliminary Results from PET Imaging Studies
JOURNAL OF NUCLEAR MEDICINE(2022)
摘要
232 Objectives: Cortisol is known to promote adipocyte differentiation and maturation, and prolonged exposure to excess cortisol contributes to development of obesity and metabolic dysregulation. The intracellular enzyme 11β-hydroxysteroid dehydrogenase type 1 (11β-HSD1) enzyme catalyzes the conversion of inactive cortisone to active cortisol. The amount of 11β-HSD1 in liver and brain may be a key to understanding obesity pathophysiology, but has not been directly quantified in vivo in humans. Recently, we characterized the PET radioligand 11C-AS2471907[1], including its 18F-labeled counterpart [2], both of which have high uptake in the liver and brain and can be used for quantitative measurement of 11β-HSD1 in vivo. We have also observed reduced 11β-HSD1 levels in the human brain with increasing body mass index (BMI)[3]. With this in mind, we performed PET imaging studies to examine 11β-HSD1 levels in both the liver and brain.
Methods: Two Sprague-Dawley rats were injected with 18F-AS2471907 via tail vein to examine specific binding in the liver. A baseline scan was performed in one rat (weight: 276g) with 17.5 MBq of 18F-AS2471907 (mass: 0.271µg). A preblocking study was performed in a second rat (309 g) with administration of the 11β-HSD1 inhibitor AS2471907 (10.8mg/kg) at 15 min prior to the injection of 9.1 MBq of 18F-AS2471907 (mass: 0.192µg). Listmode PET data were acquired for 60 min and rebinned into frames of 8x30s, 3x120s, and 10x300s on the Inveon microPET/CT scanner. PET reconstructions were performed with 3D-OSEM-MAP (2 OSEM iterations with 16 subsets) followed by 25 MAP iterations. An IDIF was derived from an ROI drawn on the abdominal aorta during early time frames (0-30s). Liver ROIs were drawn on summed images from 20-50min and TACs were generated. Volume of distribution (VT, mL/cm3) was calculated using the Logan graphical approach using the aorta TAC as the IDIF and t* of 25min. Three human individuals (2F/1M, 29-33y) with a range of BMIs from lean to obese (22.6, 27.5 and 34.4) underwent PET/CT imaging after injection of 11C- or 18F-AS2471907. Two participants were scanned for 2 h with 1 bed position centered over the liver with the exception of 30-60min when whole-body images were acquired using step and shoot bed positions from head to mid-thigh. For the third participant, 18x5 min whole-body continuous bed motion images were acquired. Regions-of-interest (ROI) were drawn manually for the brain and liver on a summed 0-10min PET image. Both ROIs were applied to the dynamic PET images to generate time-activity-curves (TACs). TACs were summed to generate SUV (30-60min) for liver and brain.
Results: Reduced specific binding can be seen in the rat liver when comparing baseline (Fig 1A) and blocking (Fig 1B) SUV (20-50 min) images. Baseline Logan VT in the rat liver was 13.0 and reduced to 4.2 in blocking scan, indicating that 68% of the liver signal is specific binding. In the human scans, liver uptake levels are consistent with a rank order increase in SUV with increasing BMI (Fig 1C), while uptake levels in the brain are consistent with a rank order decrease in SUV with increasing BMI (Fig 1D).
Conclusions: These preliminary studies suggest a high fraction of specific binding in the rat liver and that obesity is associated with increased 11β-HSD1 levels in the liver and a decrease in the brain. Although a small cohort, the negative correlation of decreasing brain 11β-HSD1 level with increasing BMI is consistent with our previous results[3]. Caveats from the current study include the lack of blood sampling to perform quantitative modeling evaluation. PET imaging study in a larger cohort of lean and obese individuals is ongoing with arterial blood sampling for quantitative analysis that may fully elucidate a multi-organ understanding of cortisol and 11β-HSD1 in obesity.
REFERENCES: [1] Gallezot, JNM, 2019 [2] Bhatt, JCBFM, 2019 [3] Bini, SNMMI Annual Meeting, 2018 [4] Rask, JCEM, 2002 [5] Yau, Front. Aging Neurosci, 2012
更多查看译文
AI 理解论文
溯源树
样例
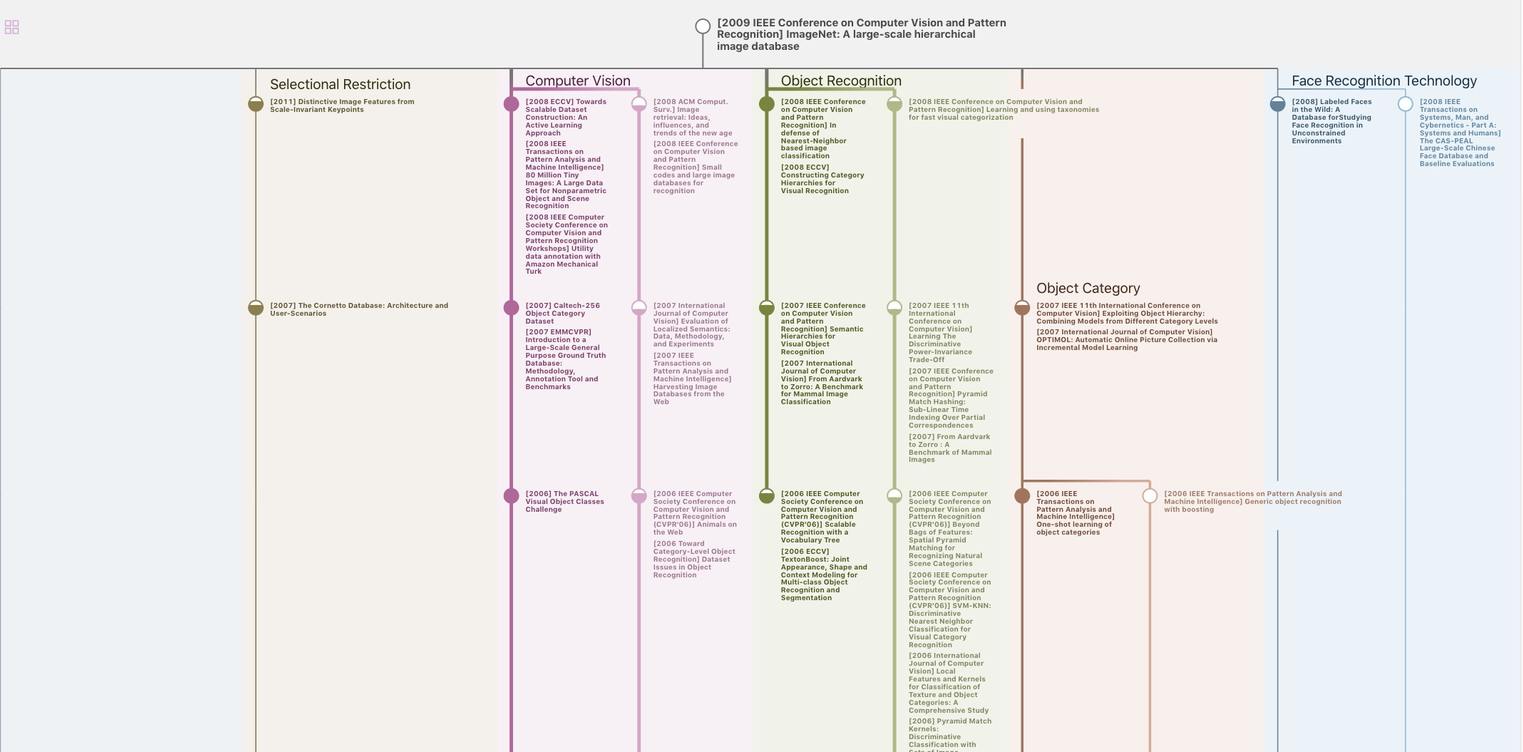
生成溯源树,研究论文发展脉络
Chat Paper
正在生成论文摘要