A generalized regression neural network–based method for predicting cumulative infiltration volume in shallow slopes
BULLETIN OF ENGINEERING GEOLOGY AND THE ENVIRONMENT(2021)
摘要
Developing a method for quickly estimating the cumulative infiltration in shallow slopes is essential for engineers to evaluate slope stability. In this paper, the hydraulic parameters of the Yan’an loess in China were obtained through physical mechanics test. Numerical experiments with different slope inclinations, soil thicknesses, rainfall intensities, and initial moisture contents were carried out to obtain the cumulative infiltration curves. The Philip model was used to fit the cumulative infiltration curves. The slope inclination, soil thickness, rainfall intensity, and initial moisture content were served as the input variables, and the parameters of the Philip model were the output variables. A generalized regression neural network–based method was proposed to estimate the cumulative infiltration quickly. Three indicators (coefficient of determination, mean squared error, and mean absolute error) show that the performance of the proposed method is superior to those of the multiple linear regression model, the back propagation neural network, and radial basis function neural network. Parametric analysis indicates that rainfall intensity is the most sensitive parameter affecting cumulative infiltration.
更多查看译文
关键词
Shallow slope,Cumulative infiltration,Loess,Generalized regression neural network,Philip model
AI 理解论文
溯源树
样例
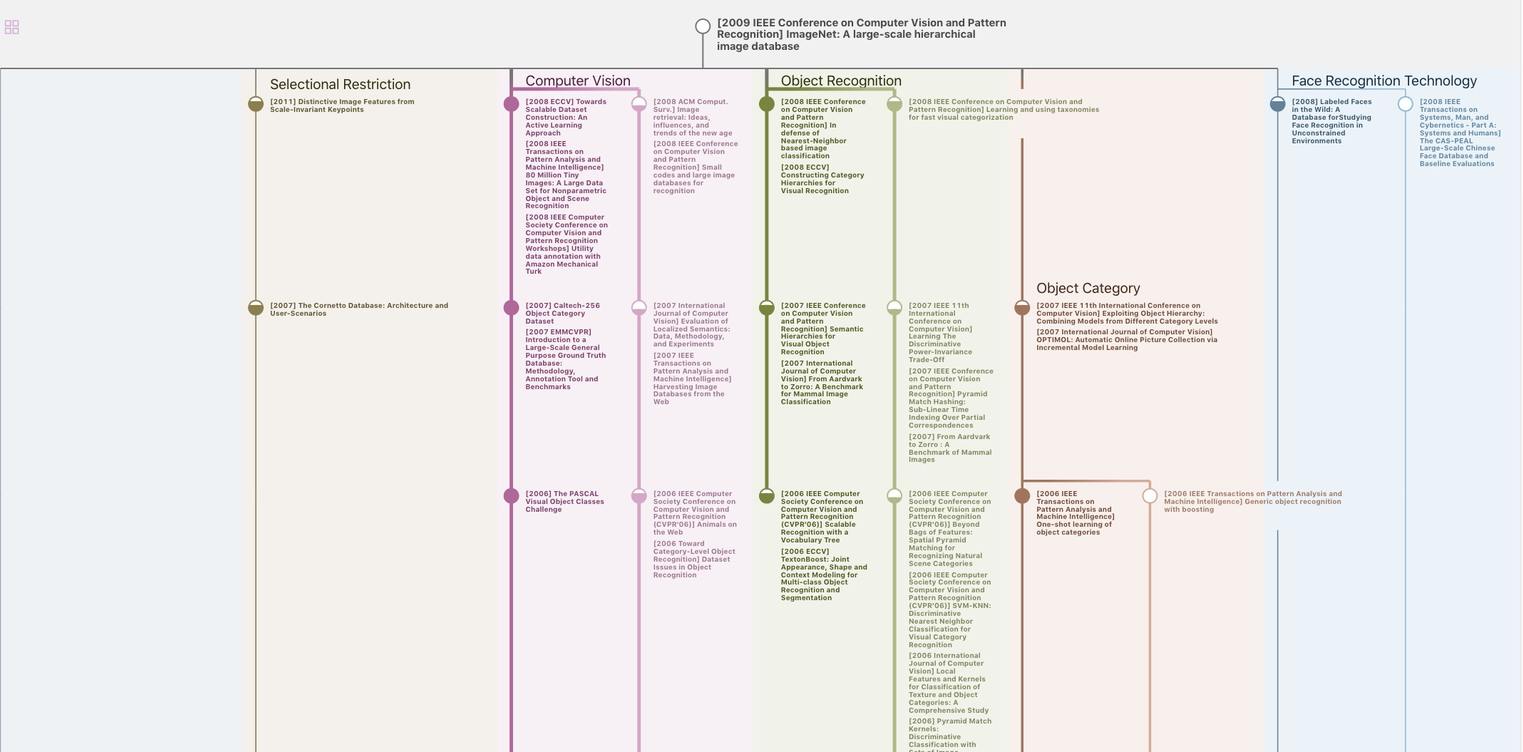
生成溯源树,研究论文发展脉络
Chat Paper
正在生成论文摘要