Reconstruct Fingerprint Images Using Deep Learning And Sparse Autoencoder Algorithms
REAL-TIME IMAGE PROCESSING AND DEEP LEARNING 2021(2021)
摘要
Fingerprinting is one form of biometrics, a science that can be used for personal identification. It is one of the important techniques and security measures for human authentication across the globe due to its uniqueness and individualistic characteristics. Fingerprints are made up of an arrangement of ridges, called friction ridges. Each ridge consists of pores, that are attached to the glands under the skin. Several algorithms proposed different approaches to recreate fingerprint images. However, these works encountered problems with poor quality and presence of structured noise on these images. In this paper, we present a novel fingerprint system that provides more unique and robust algorithms which are capable to distinguish between individuals effectively. A sparse autoencoder (SAE) algorithm is used to reconstruct fingerprint images. It is an unsupervised deep learning model that replicates its input at the output. The architecture is designed and trained with datasets of fingerprint images that are pre-processed to be able to fit them in the model. Three datasets of fingerprint images have been utilized to validate the robustness of the model. This dataset has been split into 70% for training and 30% for testing the model. SAE is fine-tuned and optimized with L-2 and sparsity regularization, thus it increased the efficiency of learning representation for the architecture. The sparse autoencoder is a suitable deep learning model to improve the recreation of fingerprint images significantly. The proposed approach showed promising results, and it can enhance the quality of reproduced fingerprint images with a clear ridge structure and eliminating various overlapping patterns.
更多查看译文
关键词
Reconstruct fingerprint, sparse autoencoder, unsupervised deep learning model, personal identification
AI 理解论文
溯源树
样例
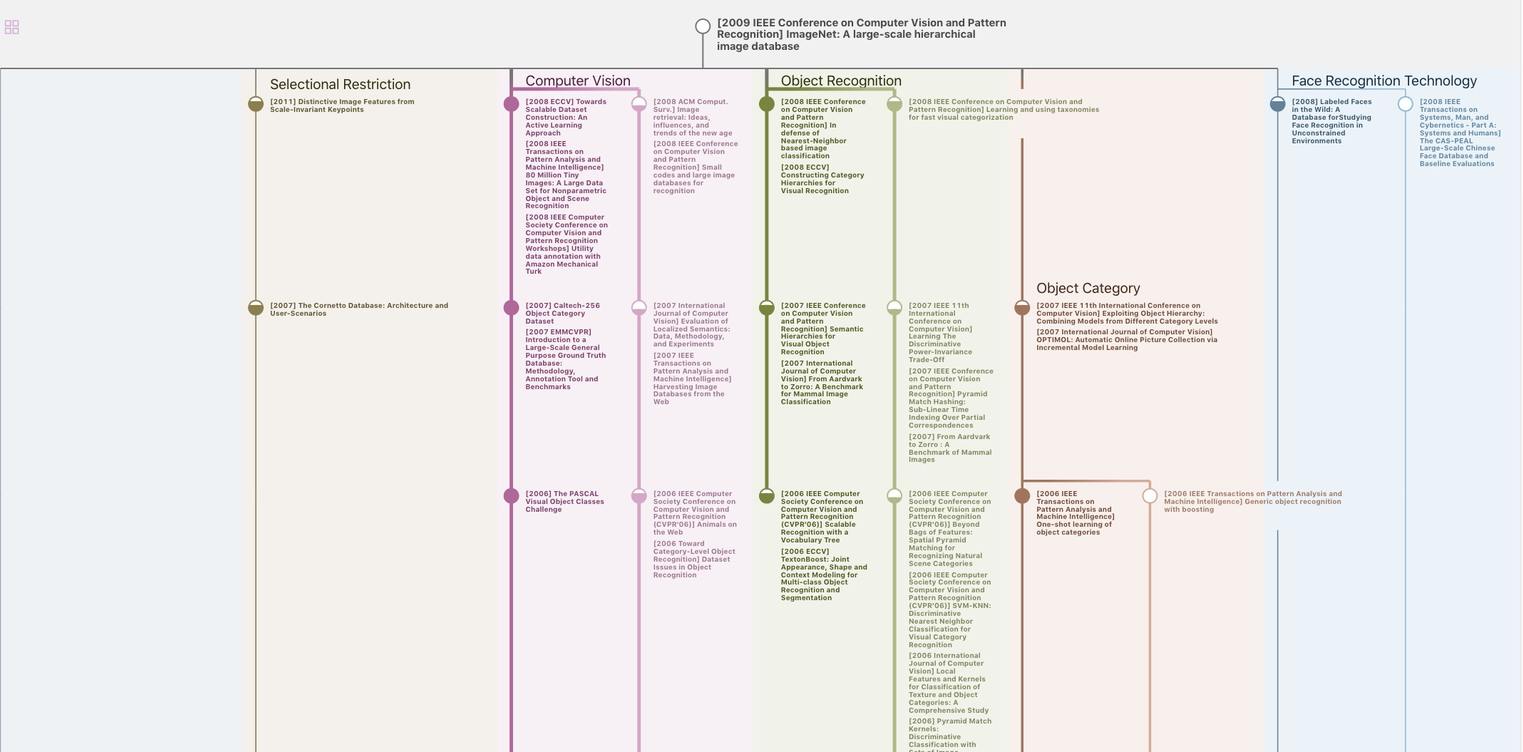
生成溯源树,研究论文发展脉络
Chat Paper
正在生成论文摘要