End-to-end classification on basal-cell carcinoma histopathology whole-slides images
MEDICAL IMAGING 2021 - DIGITAL PATHOLOGY(2021)
摘要
The increasing incidence of basal-cell carcinoma BCC skin cancer caused the amount of work for pathologists to rise to unprecedented levels. This, and the advent of digital pathology and deep learning algorithms, calls for algorithms to detect BCC in histopathology whole-slide-images. Commonly, to develop deep learning models in this field, outlined annotations have to be acquired, which are tedious and time-consuming to obtain. An alternative is end-to-end learning, which provides relief in labeling data by using a single label to predict a clinical outcome. We compared two end-to-end learning methods, multiple-instance-learning (MIL) and streaming, to detect BCC in 420 slides collected from 72 BCC positive patients. We achieved an ROC with AUC of 0.96 and 0.98 for streaming and MIL, respectively. Saliency and probability maps showed that both methods were able to learn classification of BCC in an end-to-end way using slide-level labels.
更多查看译文
AI 理解论文
溯源树
样例
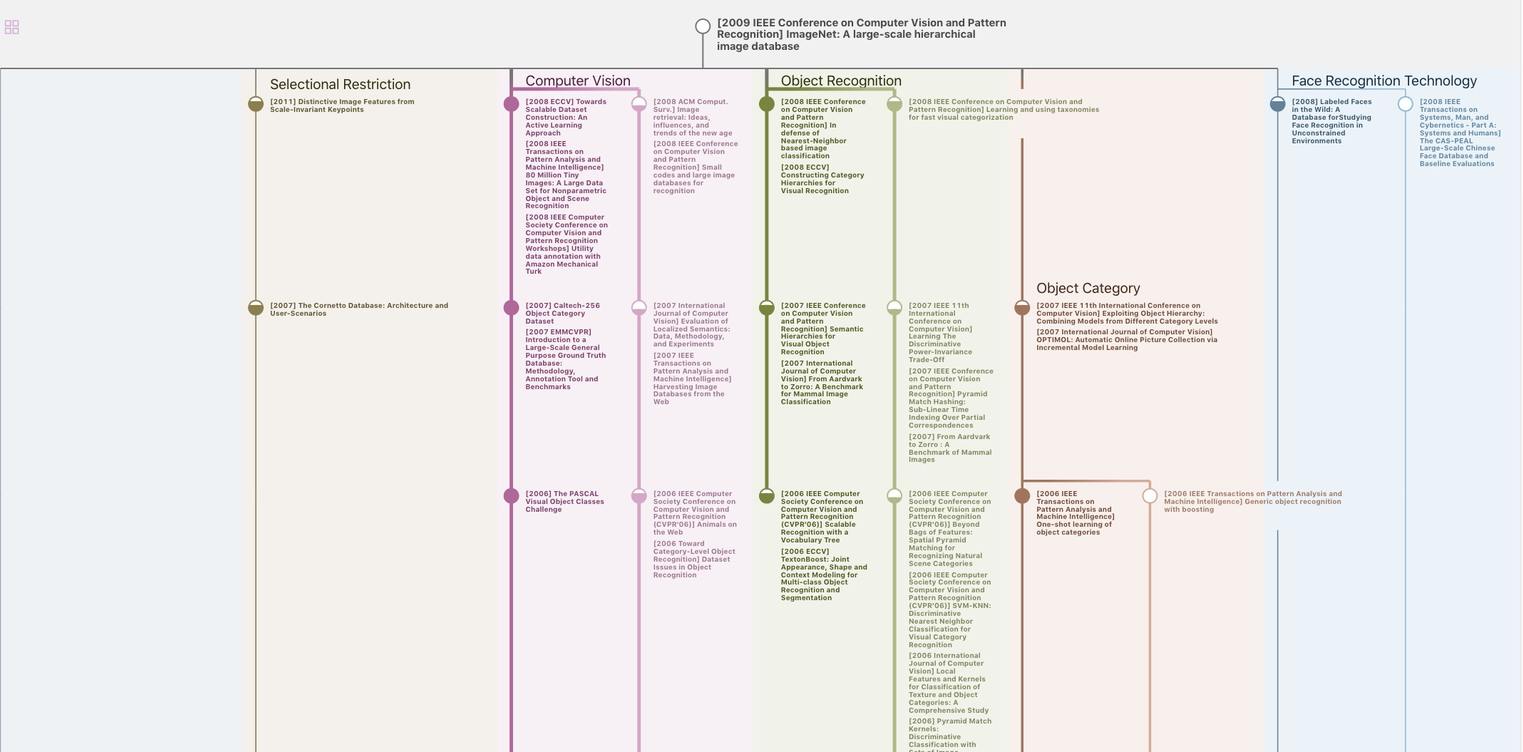
生成溯源树,研究论文发展脉络
Chat Paper
正在生成论文摘要