Detection Of Tumor Morphology Mentions In Clinical Reports In Spanish Using Transformers
ADVANCES IN COMPUTATIONAL INTELLIGENCE, IWANN 2021, PT I(2021)
摘要
The aim of this study is to systematically examine the performance of transformer-based models for the detection of tumor morphology mentions in clinical documents in Spanish. For this purpose, we analyzed 3 transformer models supporting the Spanish language, namely multilingual BERT, BETO and XLM-RoBERTa. By means of a transferlearning-based approach, the models were first pretrained on a collection of real-world oncology clinical cases with the goal of adapting transformers to the distinctive features of the Spanish oncology domain. The resulting models were further fine-tuned on the Cantemist-NER task, addressing the detection of tumor morphology mentions as a multi-class sequence-labeling problem. To evaluate the effectiveness of the proposed approach, we compared the obtained results by the domain-specific version of the examined transformers with the performance achieved by the general-domain version of the models. The results obtained in this paper empirically demonstrated that, for every analyzed transformer, the clinical version outperformed the corresponding general-domain model on the detection of tumor morphology mentions in clinical case reports in Spanish. Additionally, the combination of the transfer-learning-based approach with an ensemble strategy exploiting the predictive capabilities of the distinct transformer architectures yielded the best obtained results, achieving a precision value of 0.893, a recall of 0.887 and an F1-score of 0.89, which remarkably surpassed the prior state-of-the-art performance for the Cantemist-NER task.
更多查看译文
关键词
Transformers, Tumor morphology mentions, Natural language processing, Deep learning, Oncology
AI 理解论文
溯源树
样例
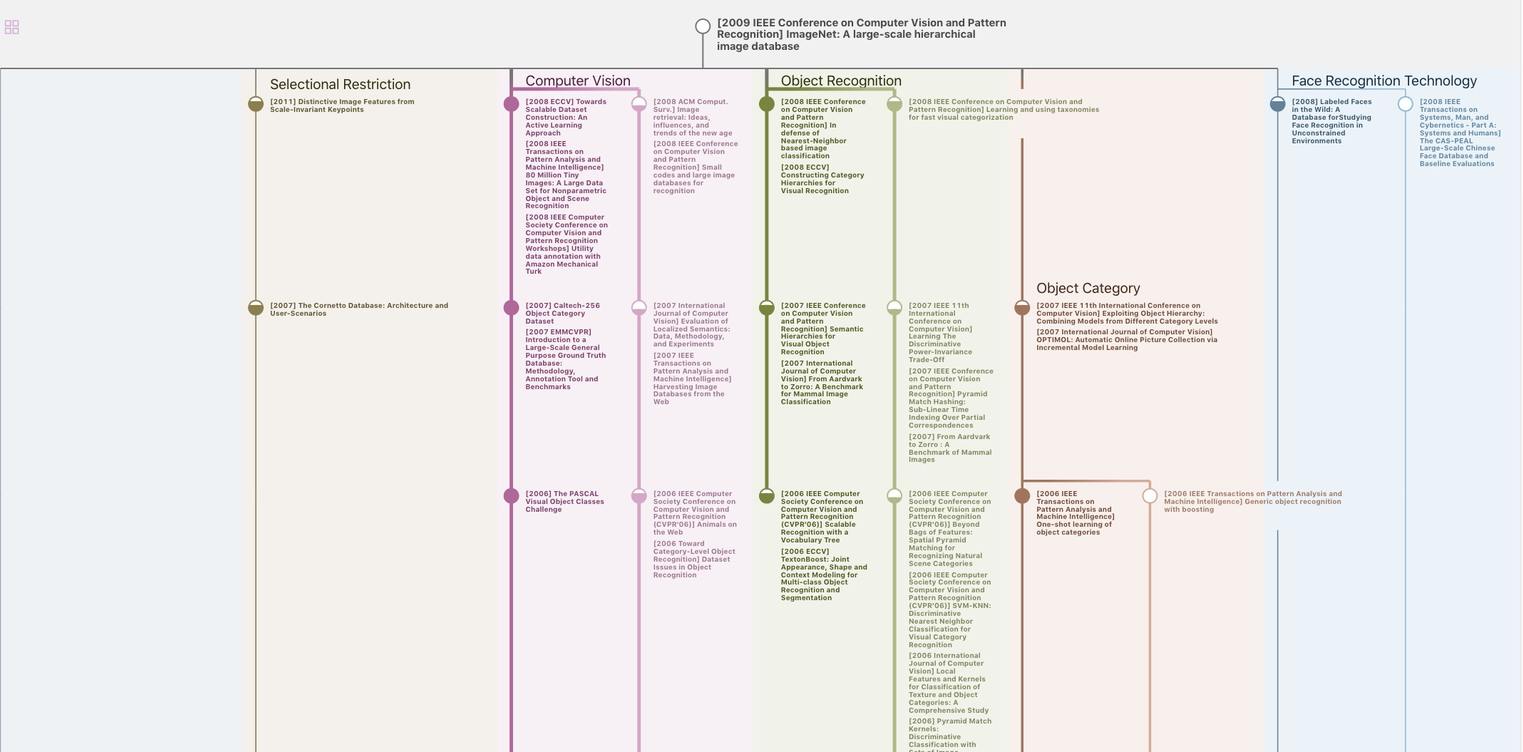
生成溯源树,研究论文发展脉络
Chat Paper
正在生成论文摘要