High-Accuracy Breast Cancer Detection In Mammography Using Efficientnet And End-To-End Training.
CANCER RESEARCH(2021)
摘要
Abstract Background:Breast cancer (BC) is the second most common cancer among women. BC screening is usually based on mammography interpreted by radiologists. Recently, some researchers have used deep learning to automatically diagnose BC in mammography and so assist radiologists. The progress of BC detection algorithms can be measured by their performance on public datasets. The CBIS-DDSM is a widely used public dataset composed of scanned mammographies, equally divided into malignant and non-malignant (benign) images. Each image is accompanied by the segmentation of the lesion. Shen et al. (Nature Sci. Rep., 2019) presented a BC detection algorithm using an “end-to-end” approach to train deep neural networks. In this algorithm, a patch classifier is first trained to classify local image patches. The patch classifier's weights are then used to initialize the whole image classifier, that is refined using datasets with the cancer status of the whole image. They achieved an AUC of 0.87 [0.84, 0.90] in classifying CBIS-DDSM images, using their best single-model, single-view breast classifier. They used ResNet (He et al., CVPR 2016) as the basis of their algorithm. Our hypothesis was that replacing the old ResNet with the modern EfficientNet (Tan et al., arXiv 2019) and MobileNetV2 (Sandler et al.,CVPR 2018) would result in greater accuracy. Methods:We tested many different models, to conclude that the best model is obtained using EfficientNet-B4 as the base model, with a MobileNetV2 block at the top, followed by a dense layer with two output categories. We trained the patch classifier using 52,528 patches with 224x224 pixels extracted from CBIS-DDSM. From each image, we extracted 20 patches: 10 patches containing the lesion and 10 from the background (without lesion). The patch classifier weights were then used to initialize the whole image classifier, that was trained using the end-to-end approach with CBIS-DDSM images resized to 1152x896 pixels, with data augmentation. The training was performed using a step learning rate of 1e-4 for the first 20 epochs then 1e-5 for the remaining 10 and batch size of 4, using 10-fold cross-validation. We used 81% of the dataset for training, 9% for validation and 10% for testing. Results:We obtained an AUC of 0.8963±0.06, using a single-model, single-view classifier and without test-time data augmentation. Conclusions:Using EfficientNet and MobileNetV2 as the basis of the BC detection algorithm (instead of ResNet), we obtained an improvement in classifying CBIS-DDSM images into malignant/non-malignant: AUC has increased from 0.87 to 0.896. Our AUC is also larger than other recent papers in the literature, such as Shu et al. (IEEE Trans Med. Image, 2020) that achieved an AUC of 0.838 in the same CBIS-DDSM dataset. Citation Format: Daniel G. Petrini, Carlos Shimizu, Gabriel V. Valente, Guilherme Folgueira, Guilherme A. Novaes, Maria L. Katayama, Pedro Serio, Rosimeire A. Roela, Tatiana C. Tucunduva, Maria Aparecida A. Folgueira, Hae Y. Kim. High-accuracy breast cancer detection in mammography using EfficientNet and end-to-end training [abstract]. In: Proceedings of the American Association for Cancer Research Annual Meeting 2021; 2021 Apr 10-15 and May 17-21. Philadelphia (PA): AACR; Cancer Res 2021;81(13_Suppl):Abstract nr 181.
更多查看译文
关键词
mammography,breast cancer,efficientnet,high-accuracy,end-to-end
AI 理解论文
溯源树
样例
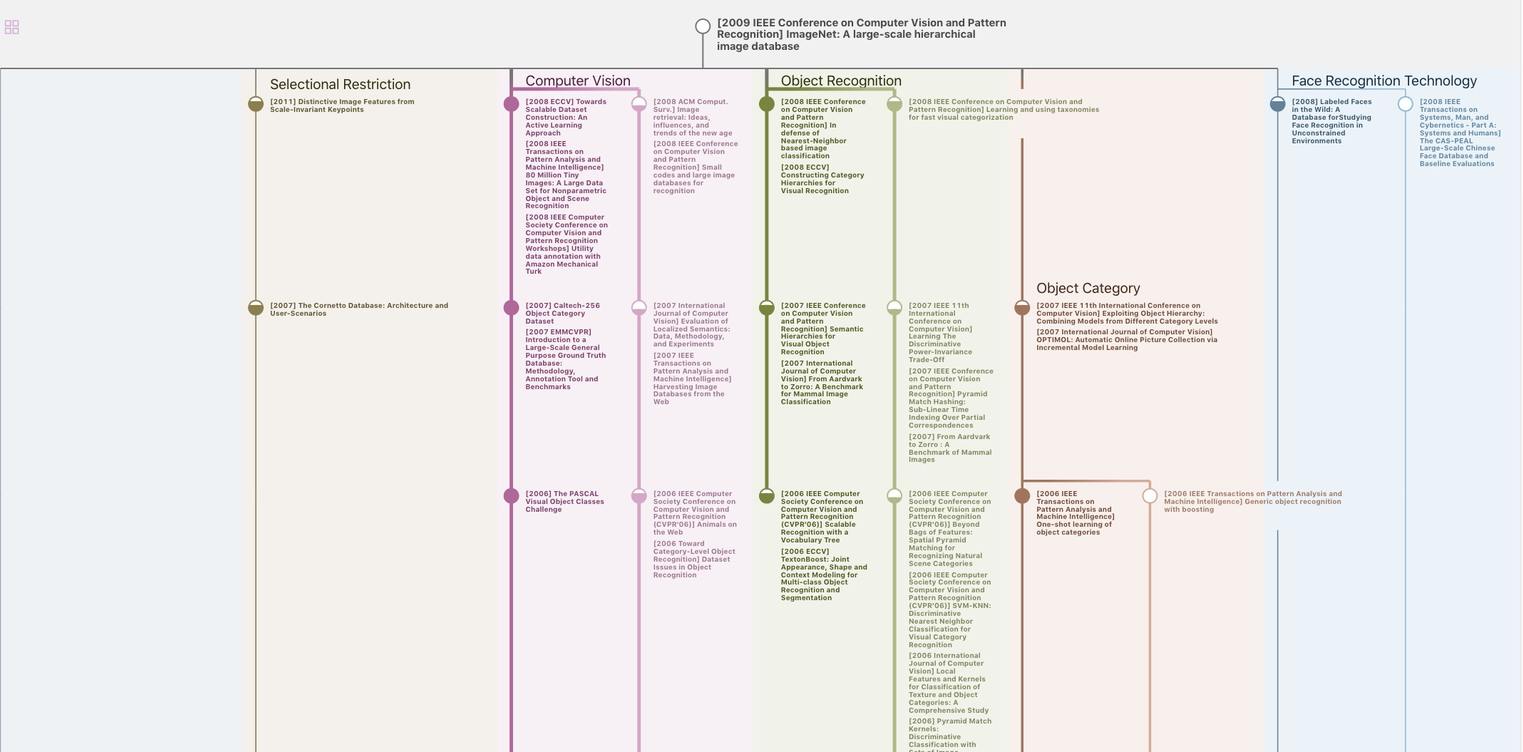
生成溯源树,研究论文发展脉络
Chat Paper
正在生成论文摘要