Rmu-Net: A Novel Residual Mobile U-Net Model For Brain Tumor Segmentation From Mr Images
ELECTRONICS(2021)
摘要
The most aggressive form of brain tumor is gliomas, which leads to concise life when high grade. The early detection of glioma is important to save the life of patients. MRI is a commonly used approach for brain tumors evaluation. However, the massive amount of data provided by MRI prevents manual segmentation in a reasonable time, restricting the use of accurate quantitative measurements in clinical practice. An automatic and reliable method is required that can segment tumors accurately. To achieve end-to-end brain tumor segmentation, a hybrid deep learning model RMU-Net is proposed. The architecture of MobileNetV2 is modified by adding residual blocks to learn in-depth features. This modified Mobile Net V2 is used as an encoder in the proposed network, and upsampling layers of U-Net are used as the decoder part. The proposed model has been validated on BraTS 2020, BraTS 2019, and BraTS 2018 datasets. The RMU-Net achieved the dice coefficient scores for WT, TC, and ET of 91.35%, 88.13%, and 83.26% on the BraTS 2020 dataset, 91.76%, 91.23%, and 83.19% on the BraTS 2019 dataset, and 90.80%, 86.75%, and 79.36% on the BraTS 2018 dataset, respectively. The performance of the proposed method outperforms with less computational cost and time as compared to previous methods.
更多查看译文
关键词
semantic segmentation, BraTS 2020, BraTS 2019, BraTS 2018, deep learning, MobileNetV2, U-Net, ResNet
AI 理解论文
溯源树
样例
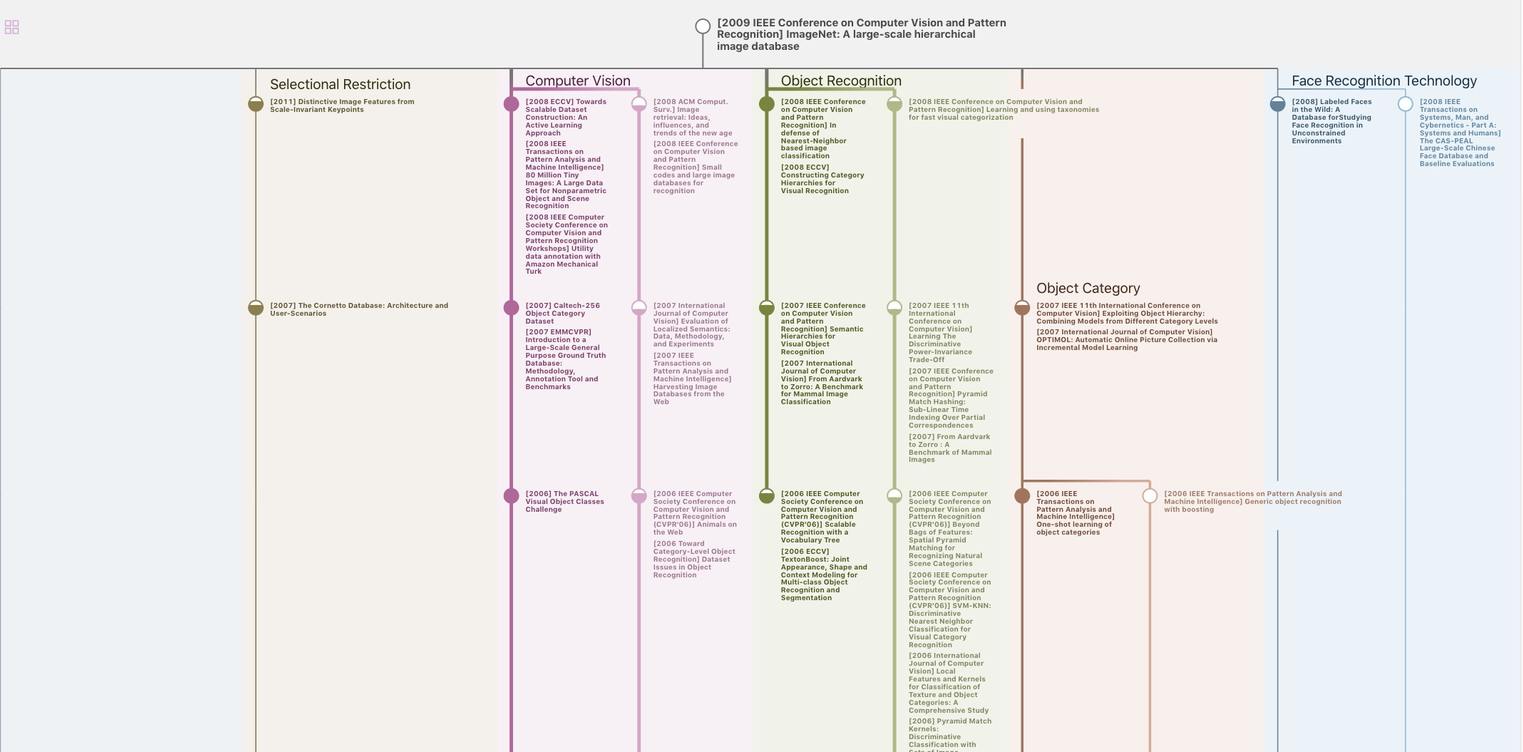
生成溯源树,研究论文发展脉络
Chat Paper
正在生成论文摘要