Scalable Partial Explainability In Neural Networks Via Flexible Activation Functions (Student Abstract)
THIRTY-FIFTH AAAI CONFERENCE ON ARTIFICIAL INTELLIGENCE, THIRTY-THIRD CONFERENCE ON INNOVATIVE APPLICATIONS OF ARTIFICIAL INTELLIGENCE AND THE ELEVENTH SYMPOSIUM ON EDUCATIONAL ADVANCES IN ARTIFICIAL INTELLIGENCE(2021)
摘要
Current state-of-the-art neural network explanation methods (e.g. Saliency maps, DeepLIFT, LIME, etc.) focus more on the direct relationship between NN outputs and inputs rather than the NN structure and operations itself, hence there still exists uncertainty over the exact role played by neurons. In this paper, we propose a novel neural network structure with Kolmogorov-Arnold Superposition Theorem based topology and Gaussian Processes based flexible activation function to achieve partial explainability of the neuron inner reasoning. The model feasibility is verified in a case study on binary classification of the banknotes.
更多查看译文
关键词
scalable partial explainability,flexible activation functions,neural networks,student abstract
AI 理解论文
溯源树
样例
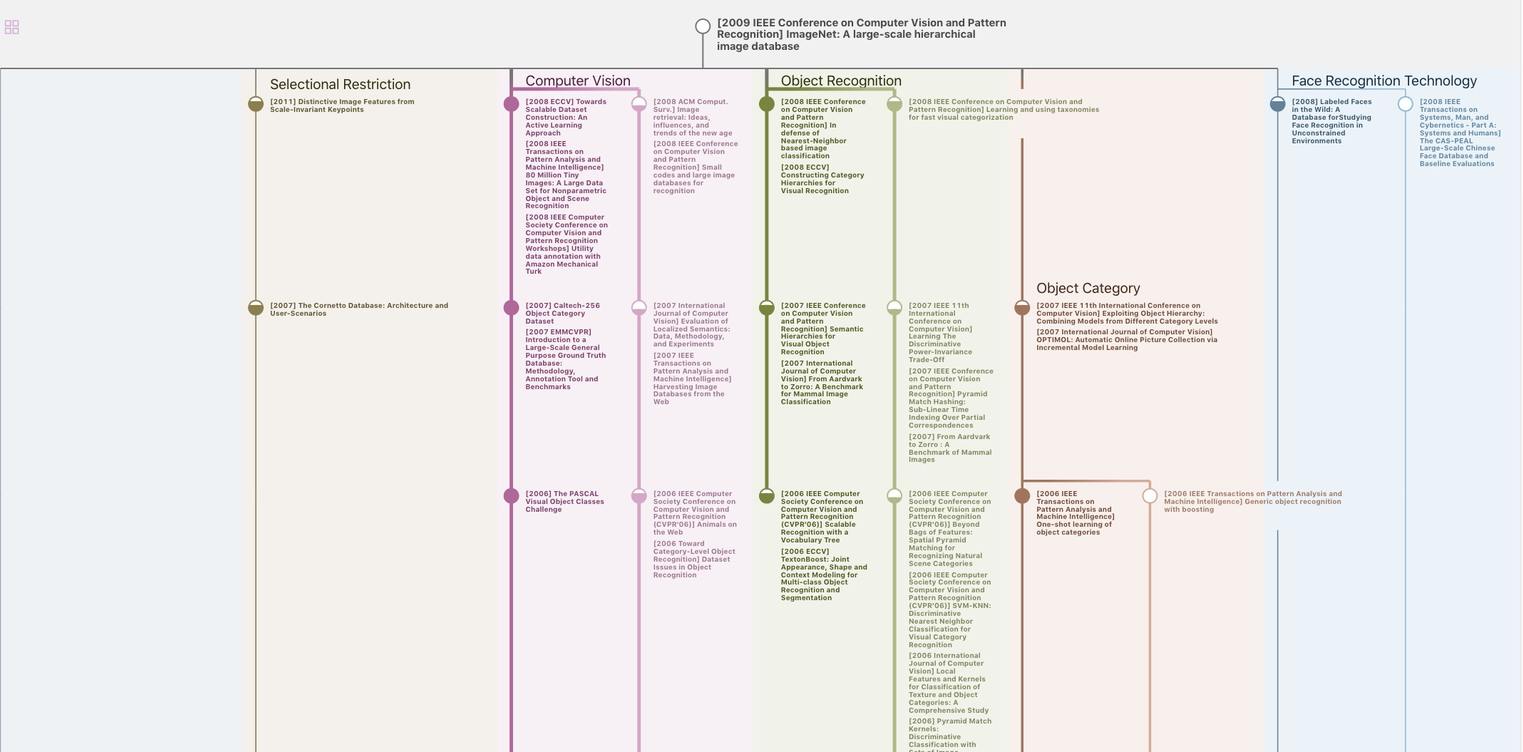
生成溯源树,研究论文发展脉络
Chat Paper
正在生成论文摘要