Multi-Label Few-Shot Learning With Semantic Inference (Student Abstract)
THIRTY-FIFTH AAAI CONFERENCE ON ARTIFICIAL INTELLIGENCE, THIRTY-THIRD CONFERENCE ON INNOVATIVE APPLICATIONS OF ARTIFICIAL INTELLIGENCE AND THE ELEVENTH SYMPOSIUM ON EDUCATIONAL ADVANCES IN ARTIFICIAL INTELLIGENCE(2021)
摘要
Few-shot learning can adapt the classification model to new labels with only a few labeled examples. Previous studies mainly focused on the scenario of a single category label per example but have not effectively solved the more challenging multi-label scenario, which has exponential-sized output space and low-data. In this paper, we propose a semantic aware meta-learning model for multi-label few-shot learning. Our approach can learn and infer the semantic correlation between unseen labels and historical labels to quickly adapt multi-label tasks based on only a few examples. Specifically, features can be mapped into the semantic space via label embeddings to exploit the label correlation, thus structuring the overwhelming output space. We design a novel semantic inference mechanism for leveraging prior knowledge learned from historical labels, which will produce good generalization performance on new labels to alleviate the overfitting caused by low-data. Finally, empirical results show that the proposed method significantly outperforms the existing state-of-the-art methods on the multi-label few-shot learning tasks.
更多查看译文
关键词
semantic inference,learning,student abstract,multi-label,few-shot
AI 理解论文
溯源树
样例
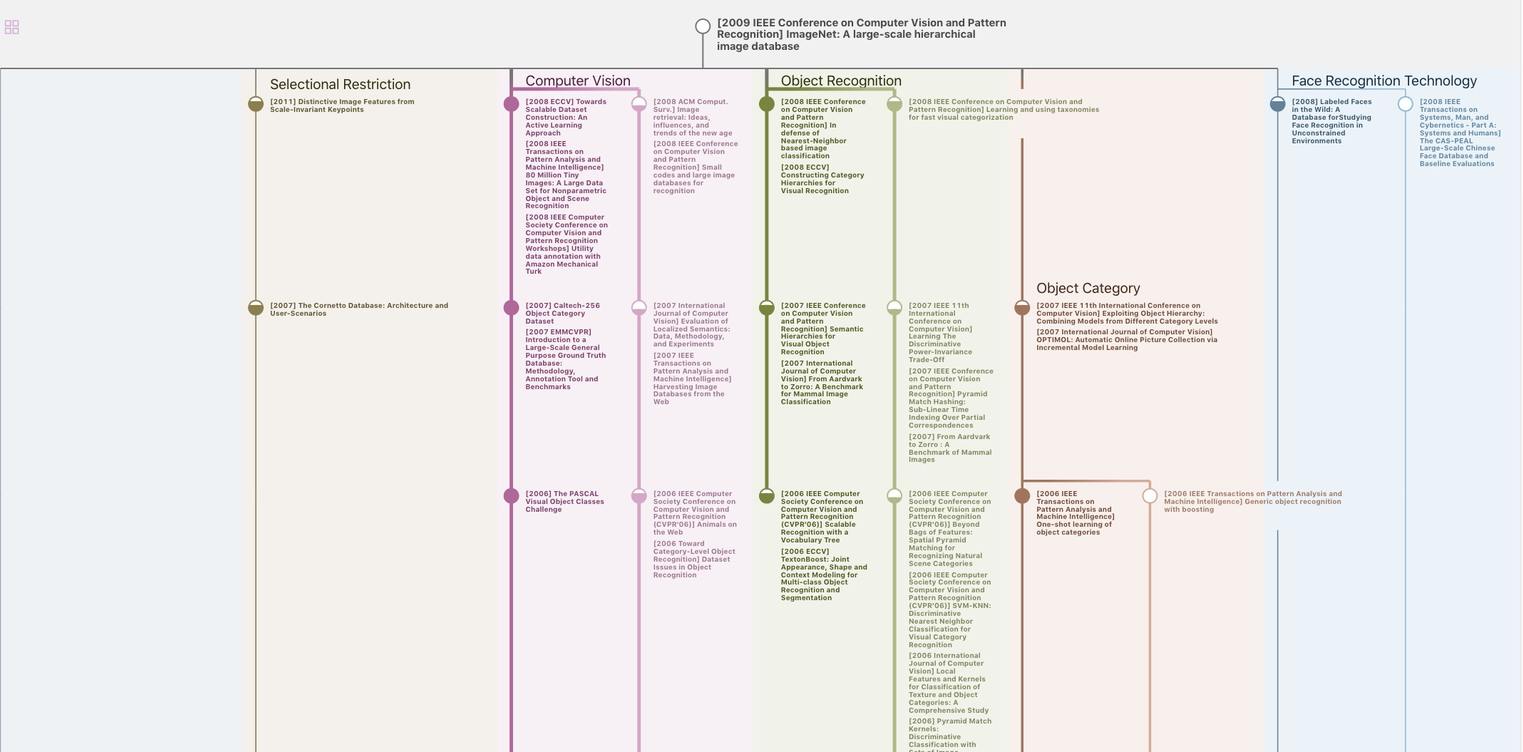
生成溯源树,研究论文发展脉络
Chat Paper
正在生成论文摘要