Extending Policy Shaping To Continuous State Spaces (Student Abstract)
THIRTY-FIFTH AAAI CONFERENCE ON ARTIFICIAL INTELLIGENCE, THIRTY-THIRD CONFERENCE ON INNOVATIVE APPLICATIONS OF ARTIFICIAL INTELLIGENCE AND THE ELEVENTH SYMPOSIUM ON EDUCATIONAL ADVANCES IN ARTIFICIAL INTELLIGENCE(2021)
摘要
Policy Shaping (Griffith et al. 2013), is a Human-in-the-loop Reinforcement Learning (HRL) algorithm. We extend this work to continuous states with our algorithm, Deep Policy Shaping (DPS). DPS uses a feedback neural network that learns the optimality of actions from noisy feedback combined with an RL algorithm. In simulation, we find that DPS outperforms or matches baselines averaged over multiple hyperparameter settings and varying feedback correctness.
更多查看译文
关键词
policy shaping,continuous state spaces,student abstract
AI 理解论文
溯源树
样例
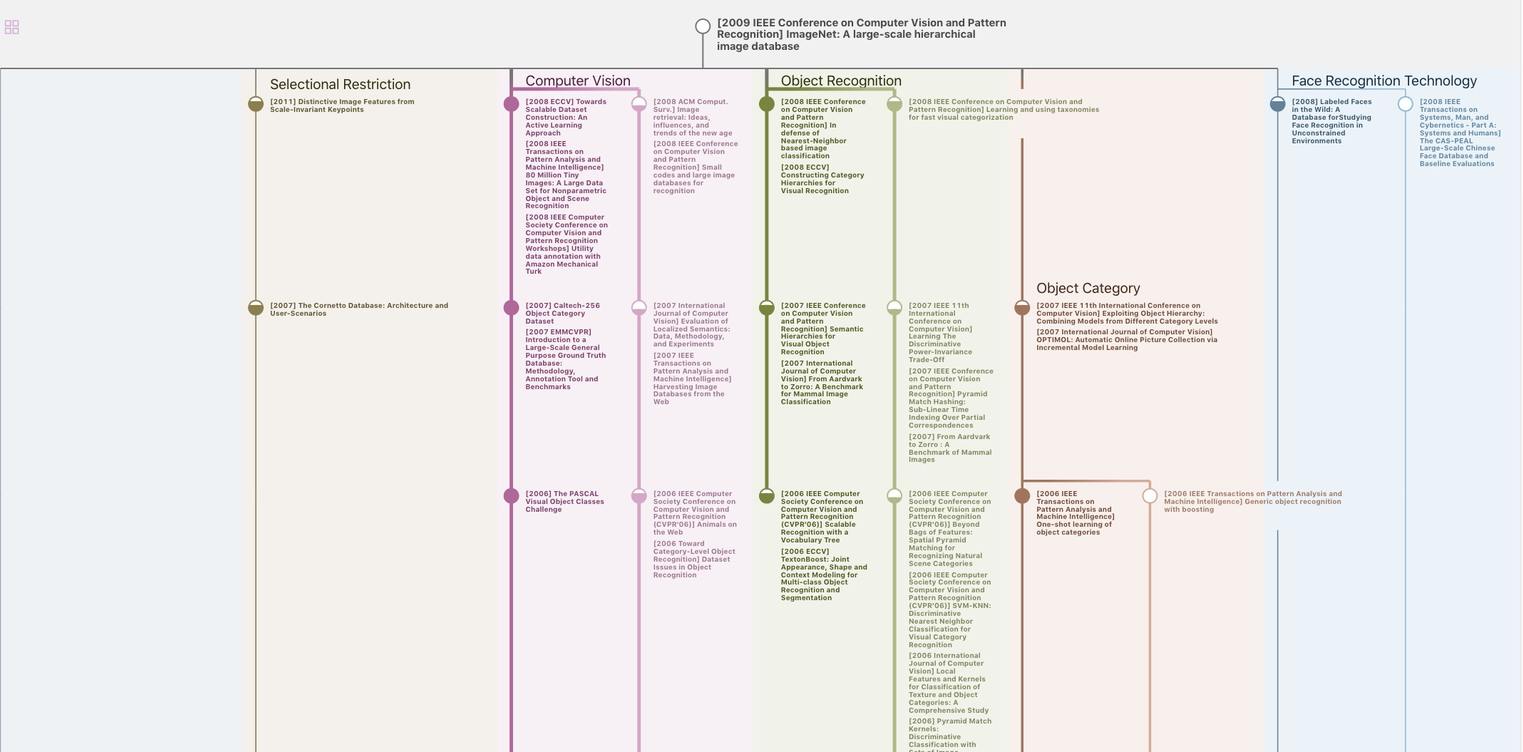
生成溯源树,研究论文发展脉络
Chat Paper
正在生成论文摘要