CECMLP: New Cipher-Based Evaluating Collaborative Multi-layer Perceptron Scheme in Federated Learning
APPLIED CRYPTOGRAPHY AND NETWORK SECURITY (ACNS 2021), PT I(2021)
摘要
Due to the large volume of available datasets and powerful computing infrastructures, federated learning has been widely explored in many scenarios, e.g. medical screening, and image processing. It refers to all participants to jointly learn shared models under the orchestration of the server without exposing their datasets. In federated learning, since the data qualities of the participants are extremely diverse, reliability is used to measure the data qualities of the participants. To make the learning task liberally and non-discriminative, participants’ reliability privacy related to their data quality should be well preserved. However, the existing work assumed that the reliability of participants is transparent for the server provider, resulting in a severe challenge in practical applications. To thwart this challenge, we propose a novel federated learning scheme, which prevents each participant’s training set privacy and reliability privacy from being revealed to the public. Moreover, to further reduce the impact of unreliable participants and improve training efficiency, we design a cipher-based reliability weighted method to differentiate and intensify different contributions of the (un)reliable participants for joint model training. Security analysis shows that our proposed scheme can achieve the desired security requirements. Moreover, extensive performance evaluations demonstrate that our design achieves higher accuracy and is more robust against unreliable participants than conventional federated learning.
更多查看译文
关键词
Federated learning,Privacy preservation,Cipher-based weighted method
AI 理解论文
溯源树
样例
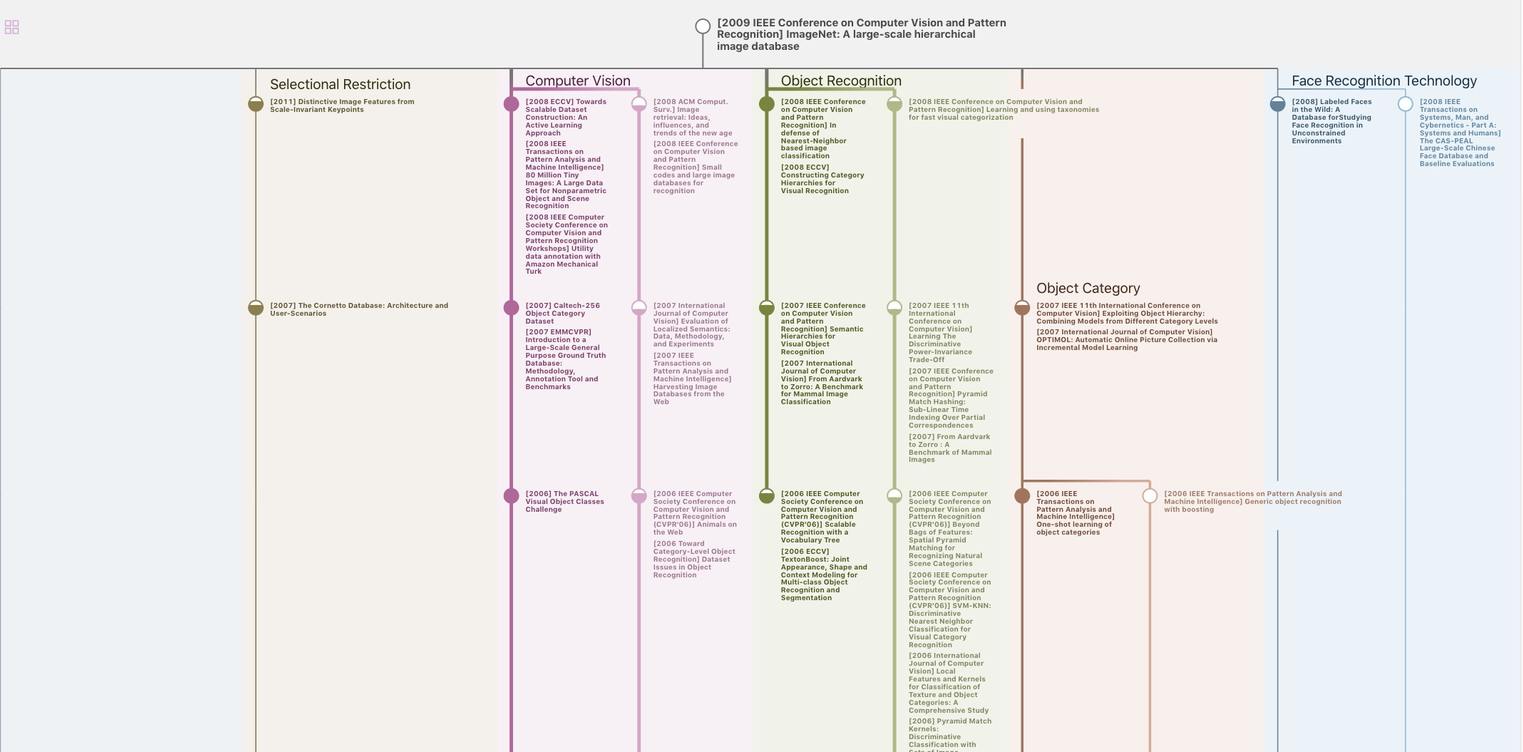
生成溯源树,研究论文发展脉络
Chat Paper
正在生成论文摘要