A multi-scale multi-attention network for dynamic facial expression recognition
Multimedia Systems(2021)
摘要
Characterizing spatial information and modelling temporal dynamics of facial images are key challenges for dynamic facial expression recognition (FER). In this paper, we propose an end-to-end multi-scale multi-attention network (MSMA-Net) for dynamic FER. In our model, the spatio-temporal features are encoded at two scales, i.e. the entire face and local facial patches. For each scale, we adopt a 2D convolutional neural network (CNN) to capture frame-based spatial information, and a 3D CNN to depict the short-term dynamics in the temporal sequence. Moreover, we propose a multi-attention mechanism by considering both spatial and temporal attention models. The temporal attention is applied on the image sequence to highlight expressive frames within the whole sequence, and the spatial attention mechanism is applied at the patch level to learn salient facial features. Comprehensive experiments on publicly available datasets (Aff-Wild2, RML, and AFEW) show that the proposed MSMA-Net model automatically highlights salient expressive frames, within which salient facial features are learned, allowing better or very competitive results compared to state-of-the-art methods.
更多查看译文
关键词
Facial expression recognition, Multi-scale multi-attention network (MSMA-Net), Spatial attention, Temporal attention
AI 理解论文
溯源树
样例
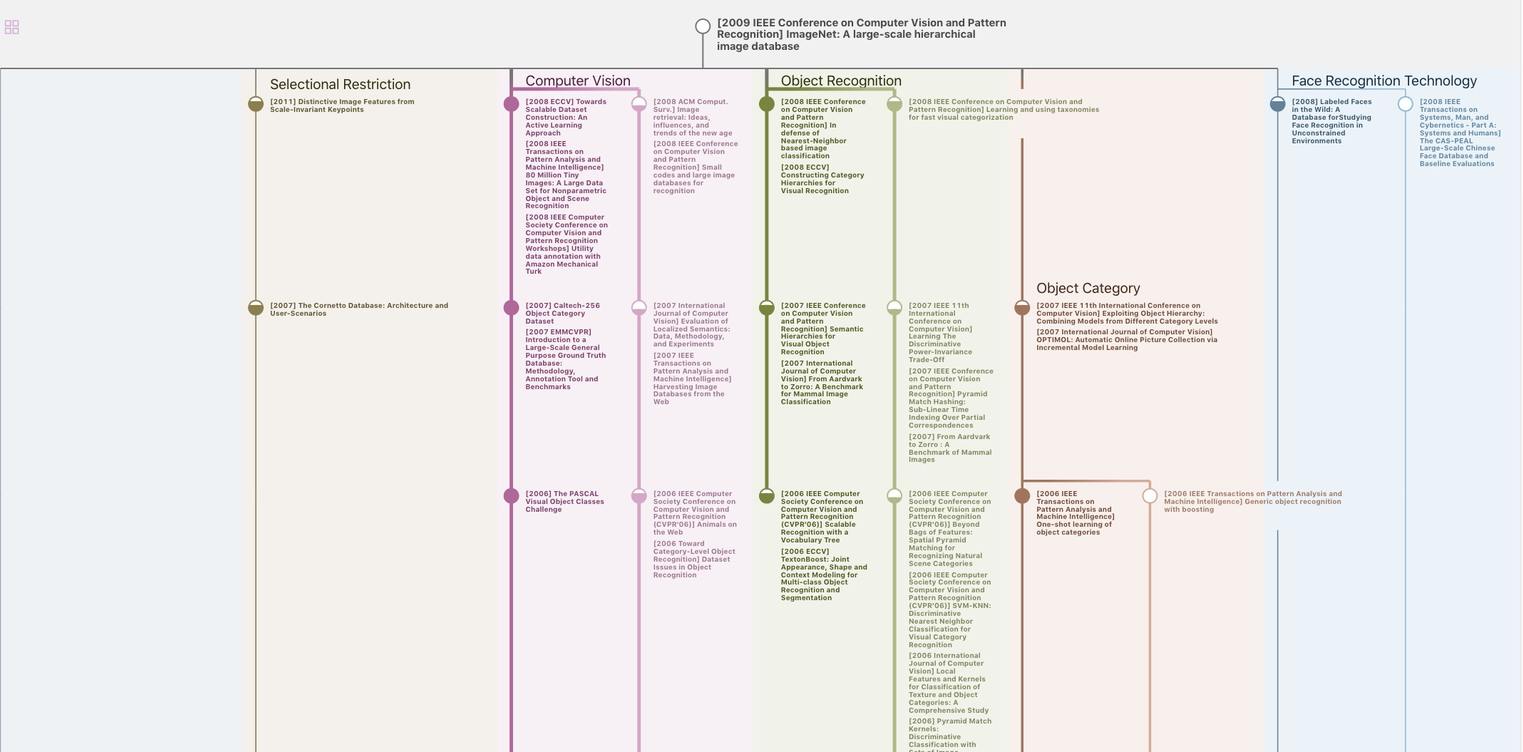
生成溯源树,研究论文发展脉络
Chat Paper
正在生成论文摘要