Vision-Based Navigation in Indoor Environments Without Using Image Database
PROCEEDINGS OF THE 27TH INTERNATIONAL TECHNICAL MEETING OF THE SATELLITE DIVISION OF THE INSTITUTE OF NAVIGATION (ION GNSS 2014)(2014)
AI 理解论文
溯源树
样例
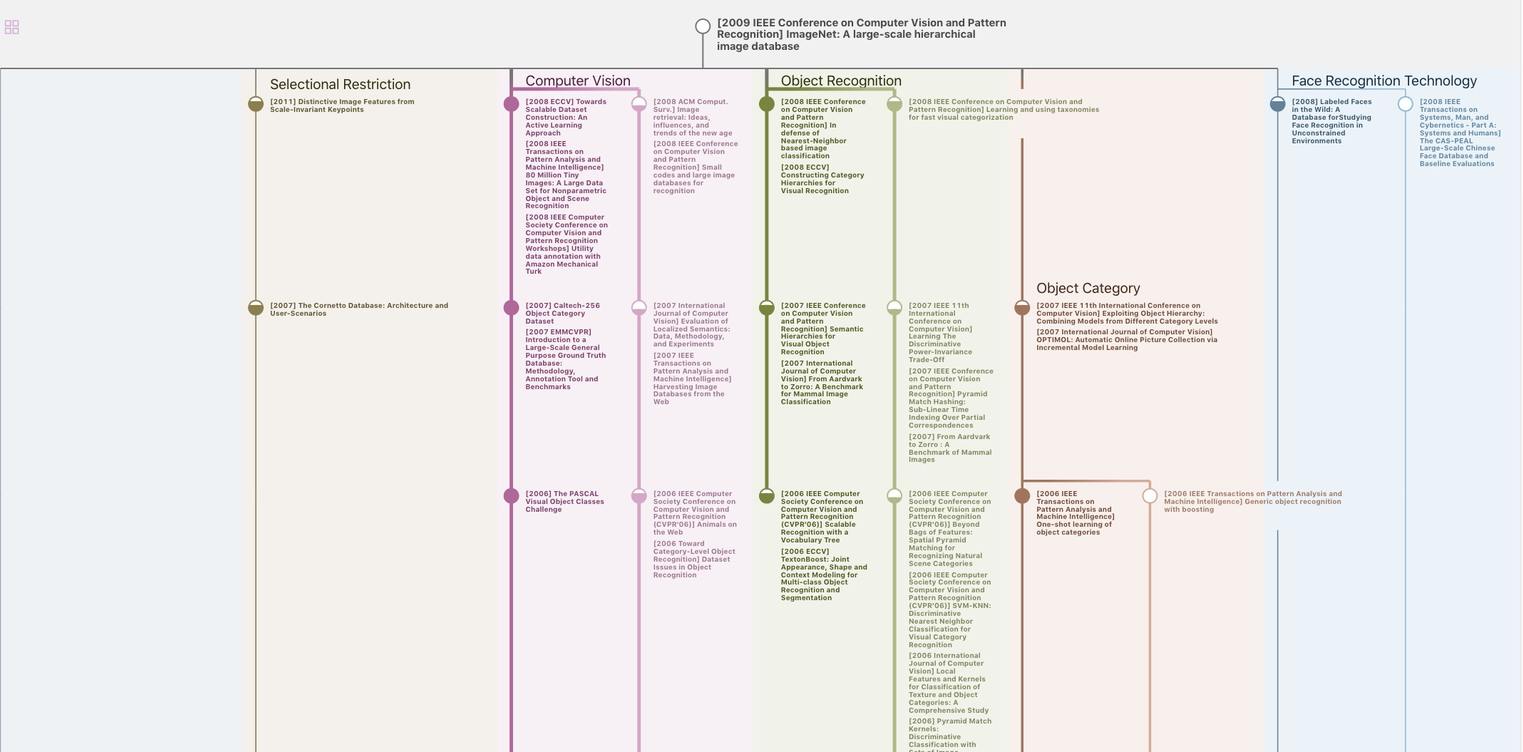
生成溯源树,研究论文发展脉络
Chat Paper
正在生成论文摘要