Trade-Off Between Diversity And Convergence In Multi-Objective Genetic Algorithms
DATA-DRIVEN MODELING FOR SUSTAINABLE ENGINEERING, ICEASSM 2017(2020)
摘要
Multi-objective genetic algorithms allow solving complex problems. They are often used to solve real-world problems. However, close scrutinizes of the execution of these algorithms show that they could suffer from premature convergence or diversity loss problems. This has an impact on the performance results. This paper introduces some tools for genetic algorithms to dynamically adapt their behaviors in order to avoid traps such as local optima. These tools lead to a trade-off between the exploitation and exploration steps. For this end, some quality criteria are introduced to assess solutions over generations. Thereafter, four execution modes are proposed to alternatively ensure diversity preservation and convergence. The results presented in this paper show that the use of these tools improves the overall performance of genetic algorithms.
更多查看译文
关键词
Multi-objective optimization, Adaptive genetic algorithm, Diversity and convergence preservation, Exploration, Exploitation
AI 理解论文
溯源树
样例
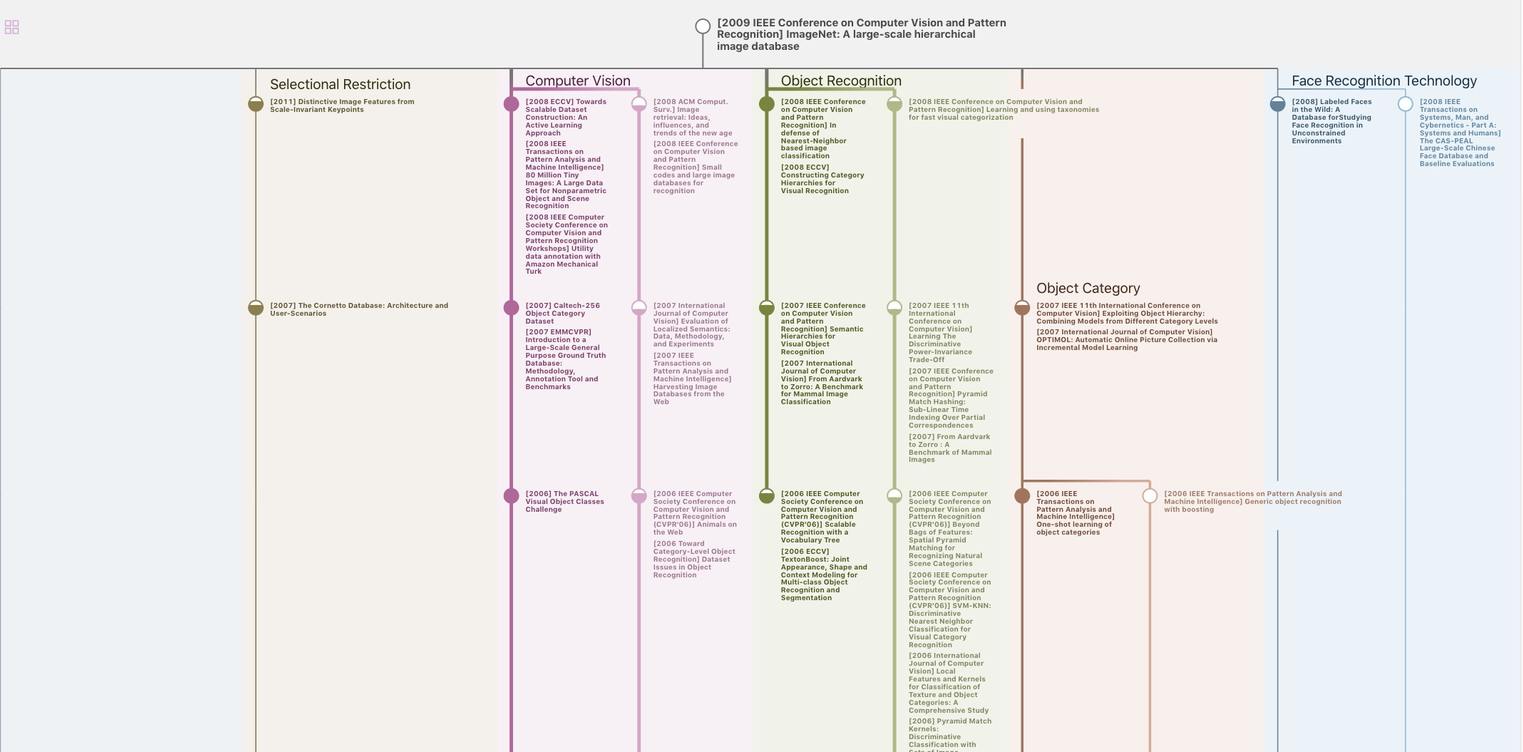
生成溯源树,研究论文发展脉络
Chat Paper
正在生成论文摘要